Distributed Reinforcement Learning Based Delay Sensitive Decentralized Resource Scheduling.
2023 21st International Symposium on Modeling and Optimization in Mobile, Ad Hoc, and Wireless Networks (WiOpt)(2023)
摘要
We address the problem of distributed resource allocation in wireless systems in the presence of dynamic user traffic and coupling resulting from interference. We propose a Reinforcement Learning (RL) framework based on a separation of concerns between frequency reuse for interference mitigation and opportunistic user scheduling. In particular we explore a setting where a stochastic game is set up among base stations to learn frequency reuse patterns and solved using multi-agent RL given an underlying choice for user scheduling. We establish the existence and convergence to a Nash equilibrium of the proposed setting. The performance of our framework and theoretical findings are evaluated through simulation and compared to more aggressive oracle-aided centralized baselines. The resulting frequency reuse policy is shown to achieve 5–25% improvements in capacity and associated delay performance over a centralized interference aware max weight scheduling policy across BSs. Furthermore, a reduced physical resource utilization on the order of 9–34% leads to a higher energy efficiency as compared to the centralized benchmark.
更多查看译文
AI 理解论文
溯源树
样例
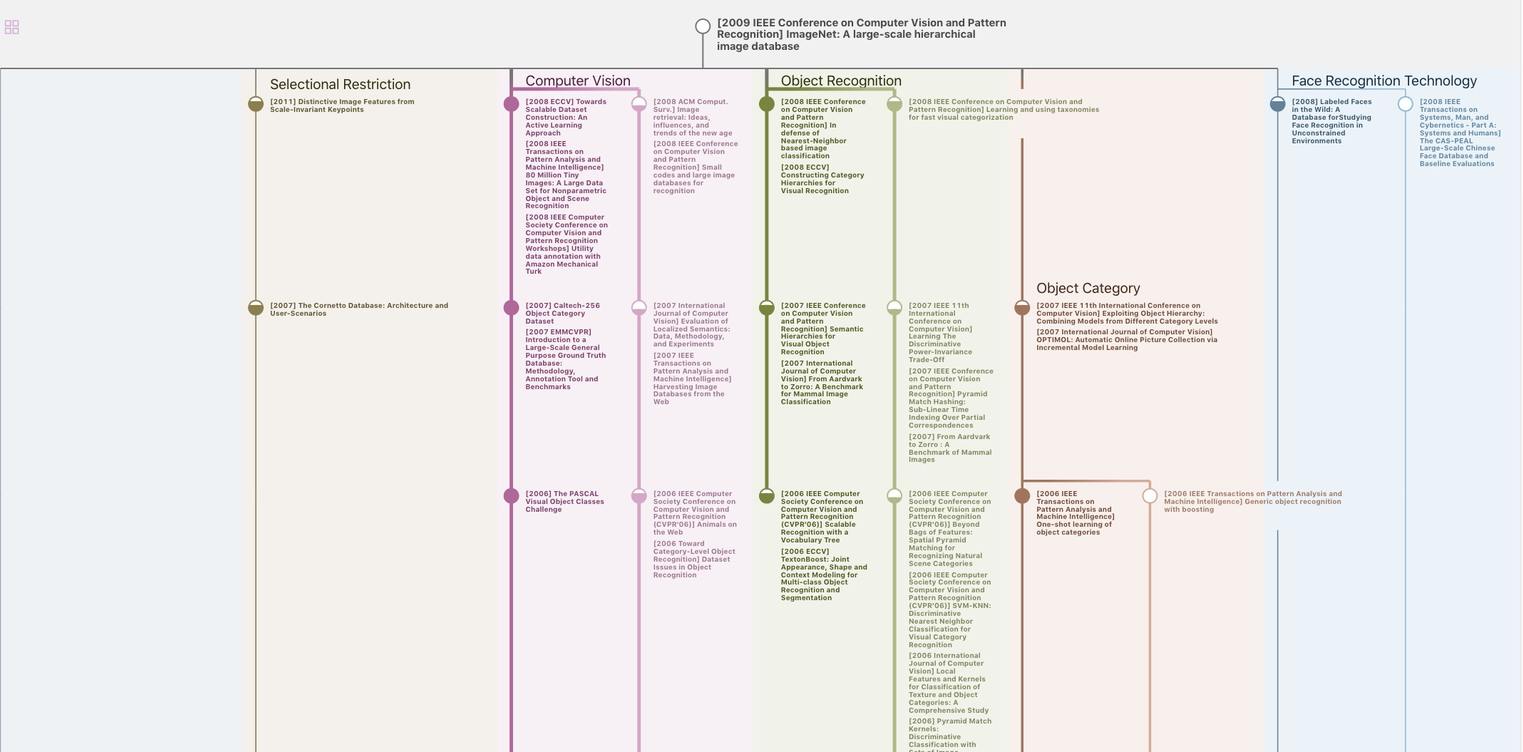
生成溯源树,研究论文发展脉络
Chat Paper
正在生成论文摘要