A comprehensive radiopathological nomogram for the prediction of pathological staging in gastric cancer using CT-derived and WSI-based features
TRANSLATIONAL ONCOLOGY(2024)
摘要
Objective: This study aims to develop and validate an innovative radiopathomics model that combines radiomics and pathomics features to effectively differentiate between stages I-II and stage III gastric cancer (pathological staging).Methods: Our study included 200 patients with well-defined stages of gastric cancer divided into a training cohort (n = 140) and a test cohort (n = 60). Radiomics features were extracted from contrast-enhanced CT images using PyRadiomics, while pathomics features were obtained from whole slide images of pathological specimens through a fine-tuned deep learning model (ResNet-18). After rigorous feature dimensionality reduction and selection, we constructed radiomics models (SVM_rad, LR_rad, and MLP_rad) and pathomics models (SVM_path, LR_path, and MLP_path) utilizing support vector machine (SVM), logistic regression (LR), and multilayer perceptron (MLP) algorithms. The optimal radiomics and pathomics models were chosen based on comprehensive evaluation criteria such as ROC curves, Hosmer-Lemeshow tests, and calibration curve tests. Feature patterns extracted from the best-performing radiomics model (MLP_rad) and pathomics model (SVM_rad) were integrated to create a powerful radiopathomics nomogram.Results: From a pool of 1834 radiomics features extracted from CT images, 14 were selected to construct radiomics models. Among these, the MLP_rad model exhibited the most robust predictive performance (AUC, training cohort: 0.843; test cohort: 0.797). Likewise, 10 pathomics features were chosen from 512 extracted from whole slide images to build pathomics models, with the SVM_path model demonstrating the highest predictive efficiency (AUC, training cohort: 0.937; test cohort: 0.792). The combined radiopathomics nomogram model exhibited optimal discriminative ability (AUC, training cohort: 0.951; test cohort: 0.837), as confirmed by decision curve analysis (DCA), which indicated superior clinical effectiveness.Conclusion: This study presents a cutting-edge radiopathomics nomogram model designed to predict pathological staging in gastric cancer, distinguishing between stages I-II and stage III. Our research leverages preoperative CT images and histopathological slides to forecast gastric cancer staging accurately, potentially facilitating the estimation of staging before radical gastric cancer surgery in the future.
更多查看译文
关键词
Computed tomography,Whole slide image,Gastric cancer,Radiopathomics,Radiomics,Pathomics,Machine Learning
AI 理解论文
溯源树
样例
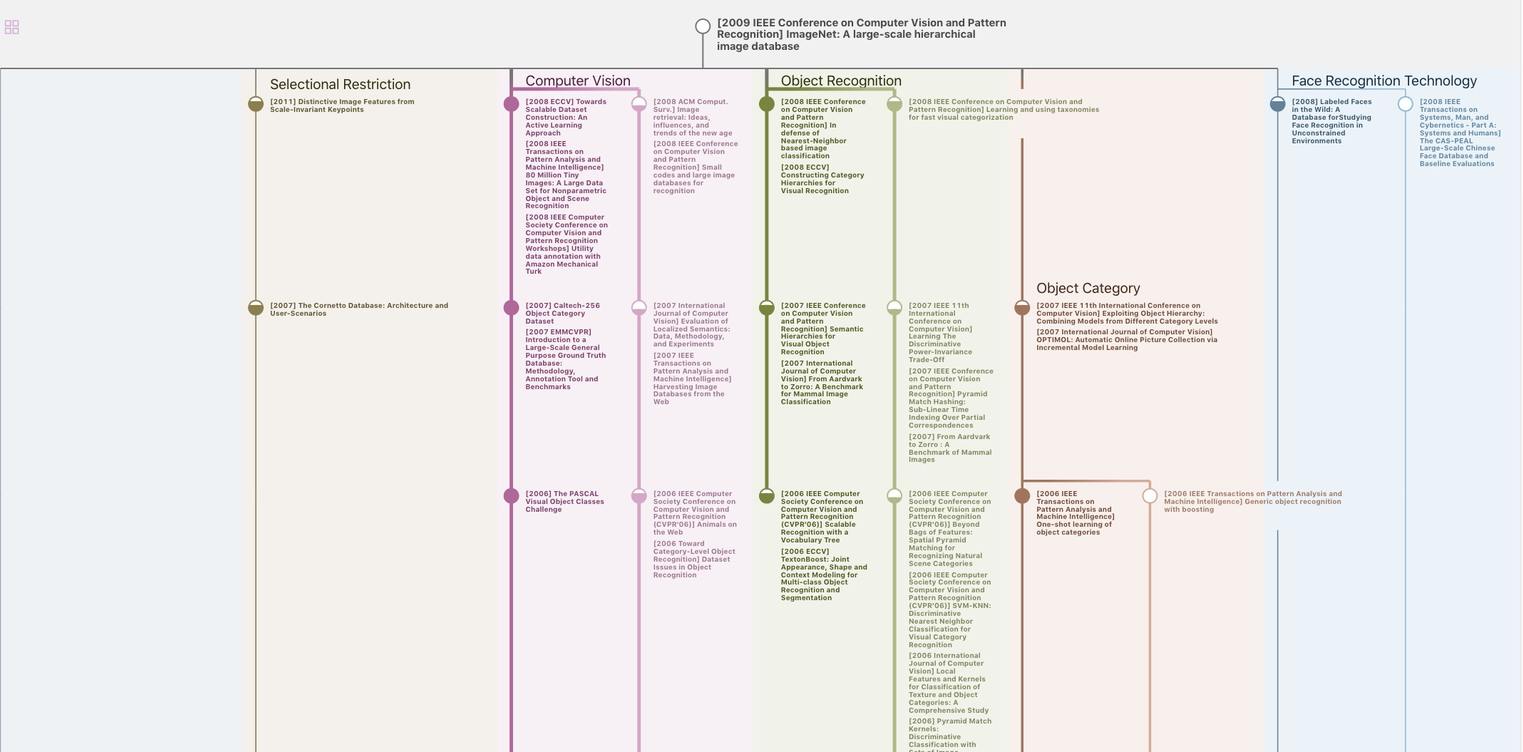
生成溯源树,研究论文发展脉络
Chat Paper
正在生成论文摘要