An automated deep learning pipeline for detecting user errors in spirometry test
BIOMEDICAL SIGNAL PROCESSING AND CONTROL(2024)
摘要
Spirometer is used as a major diagnostic tool for obstructive airway diseases and a monitoring tool for therapy response and disease staging over time. It is a sophisticated medical device employed to quantify flow and volume of air exhaled by a subject during a specific testing period. The essential metrics obtained from the spirometry test, play a crucial role in enabling healthcare professionals to thoroughly evaluate the respiratory health and condition of the individual under examination. Several spirometer measurements including Forced Vital Capacity (FVC) and Forced Expiratory Volume (FEV) serve as guidelines for diagnosis and prognosis of Chronic Obstructive Pulmonary Diseases (COPD) and asthma. However, user errors caused by different reasons, including improper handling of the equipment and poor performance during the maneuvers of the expiratory airflow, end up in incorrect treatment directions. To ensure accurate results, spirometry tests traditionally require the presence of a skilled professional to identify and address these errors promptly. A novel machine learning approach is proposed in this paper to automatically identify four such user errors based on Volume Time and Flow-Volume graphs. By detecting specific errors and providing immediate feedback to patients, reliability and accuracy of spirometry results will be improved and the need for trained professionals will be reduced. The implementation facilitates the widespread adoption of spirometry, particularly in low-resource telemedicine settings. This work implements a binary classification model distinguishing between normal and error test samples, achieving a prediction accuracy of 93%. Additionally, a 4-way classification model is presented for identifying individual error sub-types, demonstrating a prediction accuracy of 94%.
更多查看译文
关键词
Spirometry,Forced Vital Capacity,Forced Expiratory Volume,Convolutional Neural Network,FV graph,VT graph,Early Termination,Extra Breathing,Excessive Extrapolated Volume,Sub-Maximal Blast
AI 理解论文
溯源树
样例
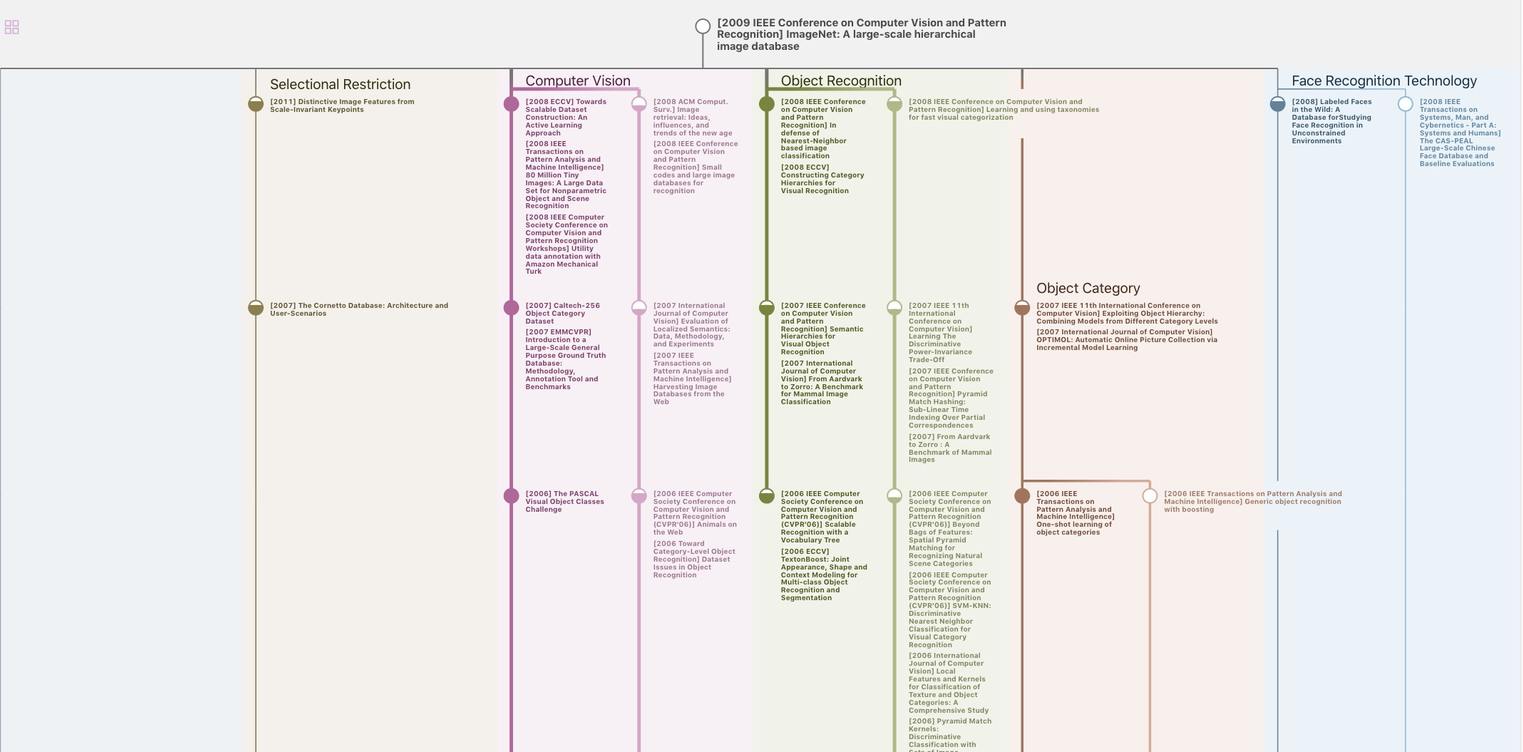
生成溯源树,研究论文发展脉络
Chat Paper
正在生成论文摘要