Key Toxic Components and Sources Affecting Oxidative Potential of Atmospheric Particulate Matter Using Interpretable Machine Learning: Insights from Fog Episodes
JOURNAL OF HAZARDOUS MATERIALS(2024)
Key words
Fine particulate matter,Reactive oxygen species,Chemical composition,Positive matrix factorization,Machine learning
AI Read Science
Must-Reading Tree
Example
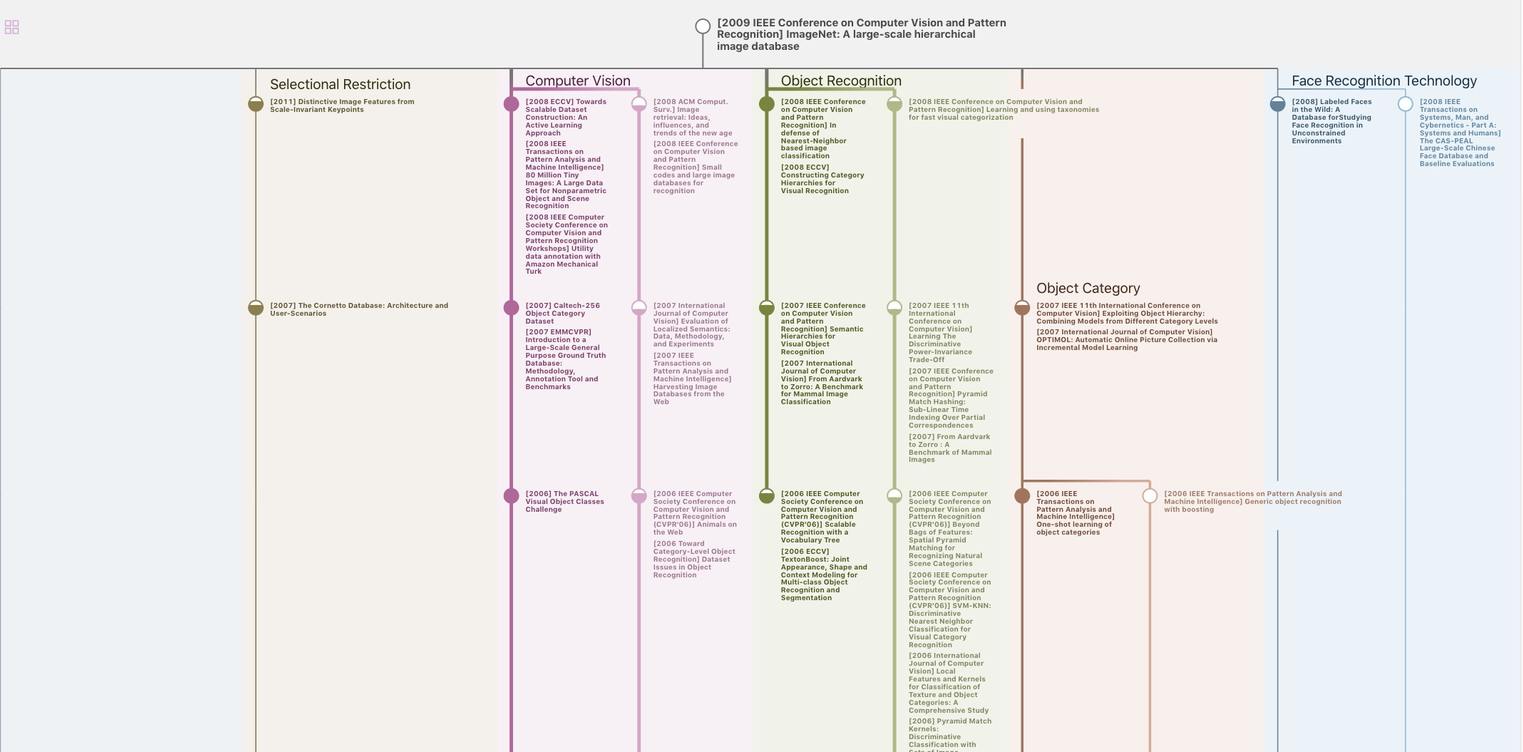
Generate MRT to find the research sequence of this paper
Chat Paper
Summary is being generated by the instructions you defined