Segmentation of White Matter Hyperintensities and Ischaemic Stroke Lesions in Structural MRI
MEDICAL IMAGE UNDERSTANDING AND ANALYSIS, MIUA 2023(2024)
摘要
White matter hyperintensities (WMH) and ischaemic stroke lesions are frequently seen on brain magnetic resonance images (MRI) of people with cerebral small vessel disease (SVD). Segmentation and differentiation of these lesions is important in diagnosis, prognosis and management, but this is challenging to automate because they have similar appearance. In this study, we used MRI scans from four cohorts of people with sporadic SVD with both WMH and ischaemic stroke, acquired under diverse imaging protocols, totalling 297 individuals. We compared two state-of-the-art medical image segmentation frameworks and investigated the data characteristics affecting the performance. We found that nnU-Net and an ensemble of two Auto3DSeg-trained models outperform (p < 0.05 for WMH and stroke Dice Coefficients (DSC)) a model previously proposed for this task, achieving mean DSC of 0.613 and 0.615 respectively compared with 0.512. The segmentation performance was better when the stroke lesions were subcortical than when they were cortical, with a mean DSC of 0.635 compared with 0.580, for nnU-Net. We found that including only a small number (n = 12) of scans with lower contrast and lower resolution to the high quality training data (n = 150) resulted in improvements (p < 0.05) in the WMH DSC and 95(th) percentile of the Hausdorff Distance (95% HD) for stroke on the 61 lower quality scans in the test set. In conclusion, cortical stroke lesions are more likely to be confounded with WMH due to their size and shape, despite their different locations, and including only a few would-be out-of-distribution examples in the training data can result in statistically significant performance gains.
更多查看译文
关键词
Cerebral small vessel disease,Machine learning,Medical image segmentation
AI 理解论文
溯源树
样例
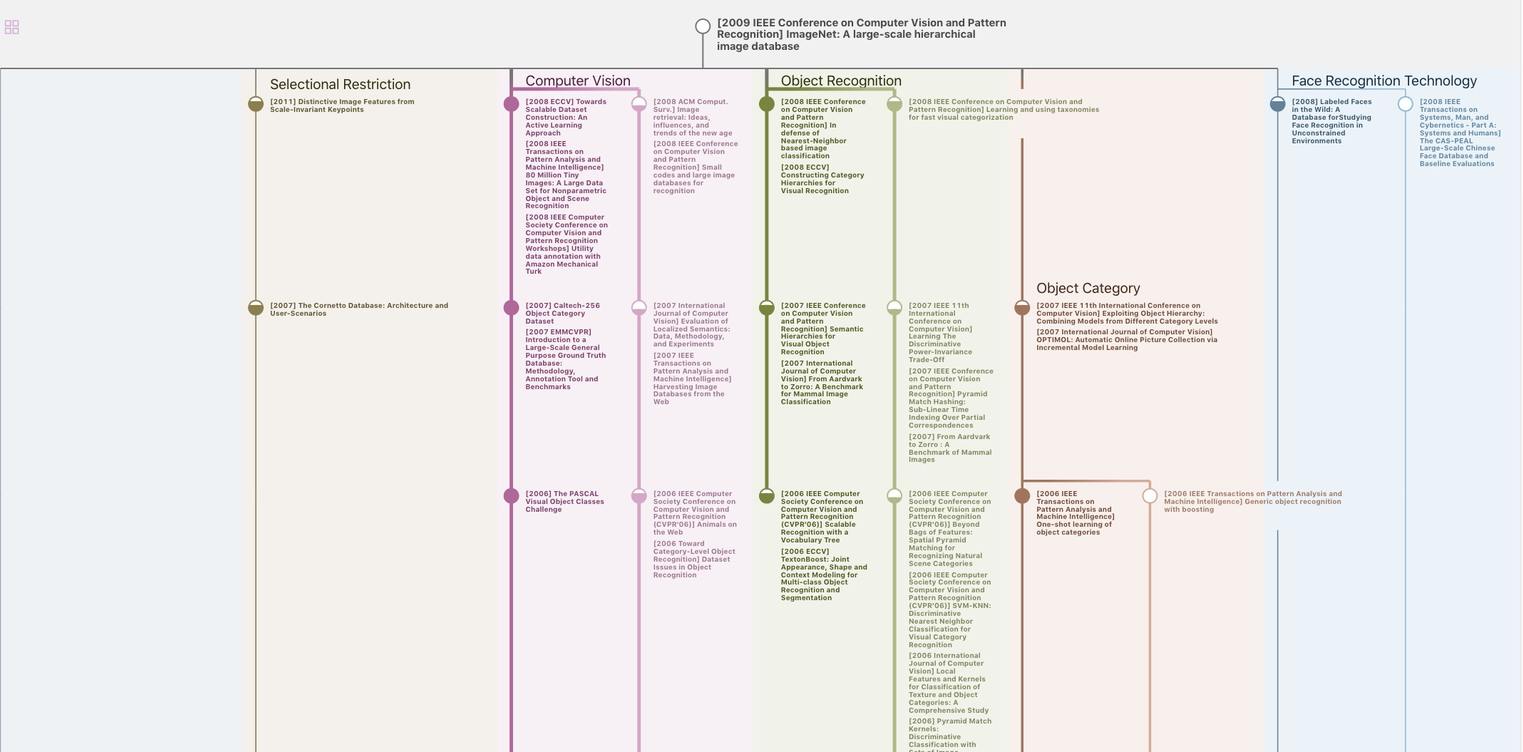
生成溯源树,研究论文发展脉络
Chat Paper
正在生成论文摘要