Markov-bridge generation of transition paths and its application to cell-fate choice
arxiv(2023)
摘要
We present a method to sample Markov-chain trajectories constrained to both the initial and final conditions, which we term Markov bridges. The trajectories are conditioned to end in a specific state at a given time. We derive the master equation for Markov bridges, which exhibits the original transition rates scaled by a time-dependent factor. Trajectories can then be generated using a refined version of the Gillespie algorithm. We illustrate the benefits of our method by sampling trajectories in the M\"uller-Brown potential. This allows us to generate transition paths which would otherwise be obtained at a high computational cost with standard Kinetic Monte Carlo methods because commitment to a transition path is essentially a rare event. We then apply our method to a single-cell RNA sequencing dataset from mouse pancreatic cells to investigate the cell differentiation pathways of endocrine-cell precursors. By sampling Markov bridges for a specific differentiation pathway we obtain a time-resolved dynamics that can reveal features such as cell types which behave as bottlenecks. The ensemble of trajectories also gives information about the fluctuations around the most likely path. For example, we quantify the statistical weights of different branches in the differentiation pathway to alpha cells.
更多查看译文
AI 理解论文
溯源树
样例
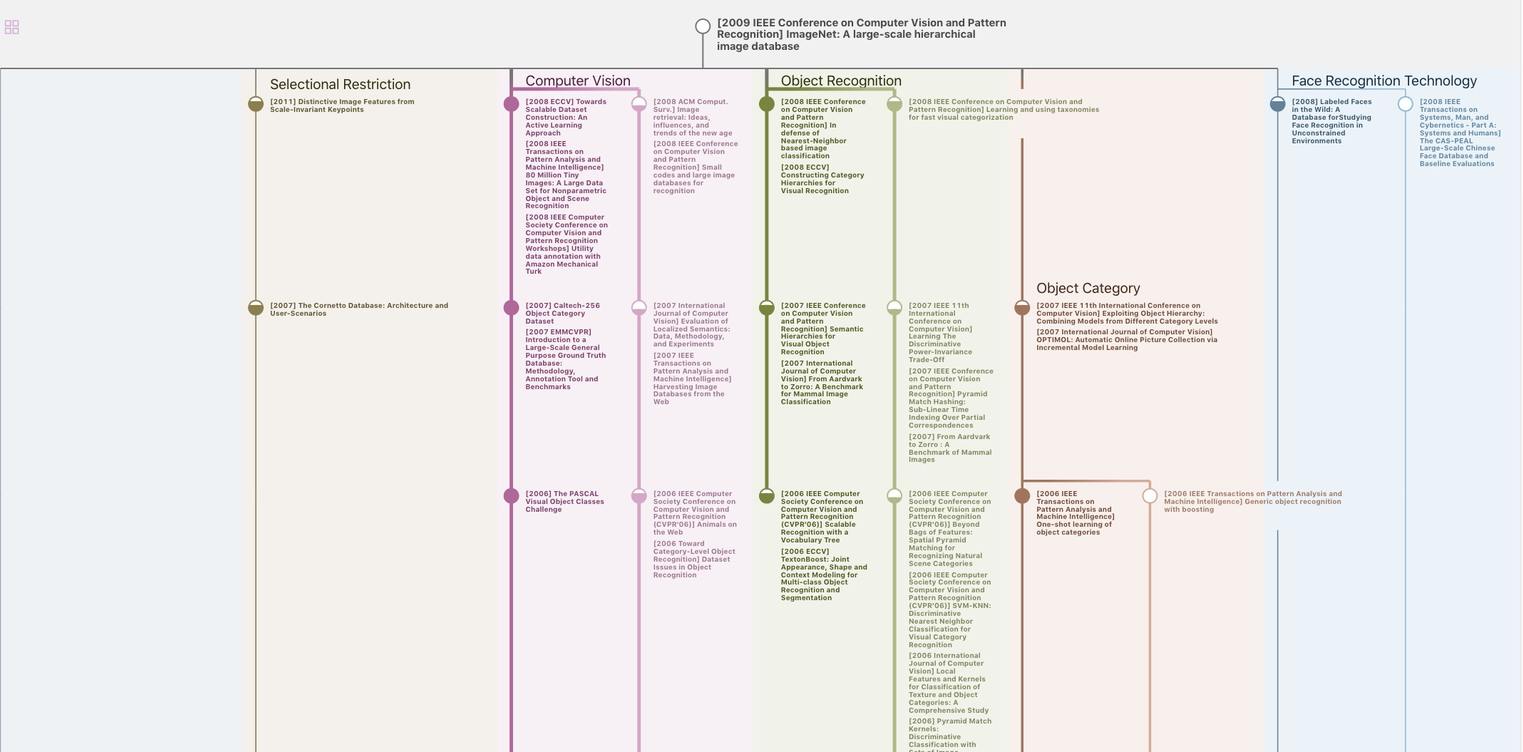
生成溯源树,研究论文发展脉络
Chat Paper
正在生成论文摘要