Porosity prediction in tight sandstone reservoirs based on a one-dimensional convolutional neural network-gated recurrent unit model
APPLIED GEOPHYSICS(2023)
摘要
Characterizing reservoir porosity is crucial for oil and gas exploration and reservoir evaluation. Due to the increasing demands of oil and gas exploration and development, characterizing reservoir porosity to the required precision using current methods is challenging. Therefore, this study proposes a Pearson correlation-random forest (RF) scheme to select optimal seismic attributes for predicting reservoir porosity and a one-dimensional convolutional neural network-gated recurrent unit (1D CNN-GRU) joint model for reservoir porosity prediction based on well logs and seismic attribute data. First, Pearson correlation-RF is used to select the optimal combination of seismic attribute data suitable for network training. The model learns the nonlinear mapping between porosity logs at well sites and seismic attribute data. It can precisely predict three-dimensional porosity volumes by extending these mappings to nonwell areas. By performing tests near a tight sandstone reservoir, the predicted porosities of the proposed 1D CNN-GRU joint model were a better fit for true porosity values than those of single-network models. Furthermore, the proposed model obtained a laterally contiguous description of the shape and porosity distribution of the tight sandstone reservoir. By integrating advanced machine learning techniques with seismic data analysis, this method provides new approaches and ideas for wide-area porosity predictions for tight sandstone reservoirs using seismic data and opens up possibilities for more detailed and accurate subsurface mapping.
更多查看译文
关键词
Porosity prediction,attribute selection,random forest,convolutional neural network,gated recurrent unit
AI 理解论文
溯源树
样例
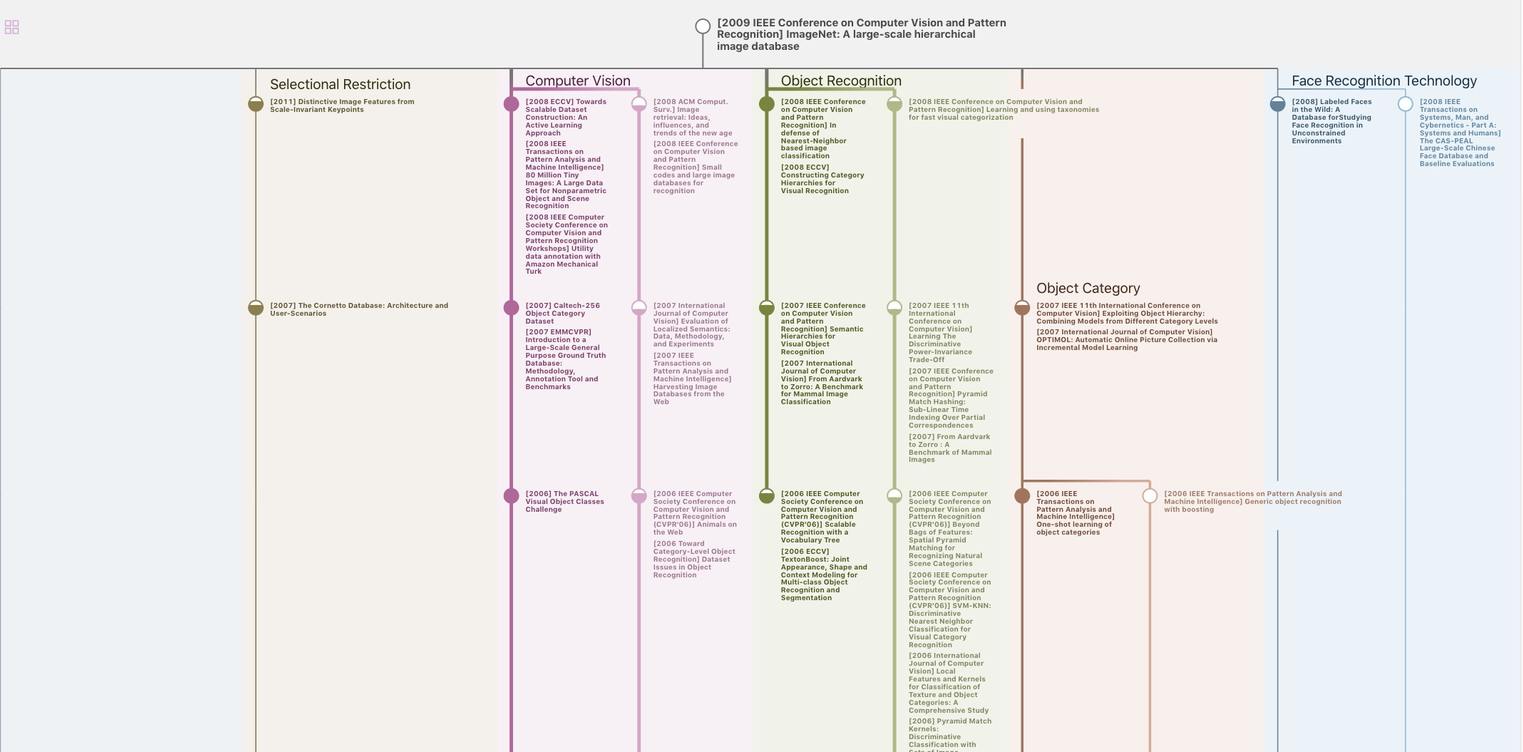
生成溯源树,研究论文发展脉络
Chat Paper
正在生成论文摘要