Random Forests for Survival Analysis and High-Dimensional Data
Springer handbooks(2023)
摘要
One of the most commonly encountered problems in biomedical studies is analyzing censored survival data. Survival analysis differs from standard regression problems by one central feature: the event of interest may not be fully observed. Therefore, statistical methods used to analyze this data must be adapted to handle the missing information. In this chapter, we provide a brief introduction of right-censored survival data and introduce survival random forest models for analyzing them. Random forests are among the most popular machine learning algorithms. During the past decade, they have seen tremendous success in biomedical studies for prediction and decision-making. In addition to the statistical formulation, we also provide details of tuning parameters commonly considered in practice. An analysis example of breast cancer relapse free survival data is used as a demonstration. We further introduce the variable importance measure that serves as a variable selection tool in high-dimensional analysis. These examples are carried out using a newly developed R package RLT, which is available on GitHub.
更多查看译文
关键词
survival analysis,forests,high-dimensional
AI 理解论文
溯源树
样例
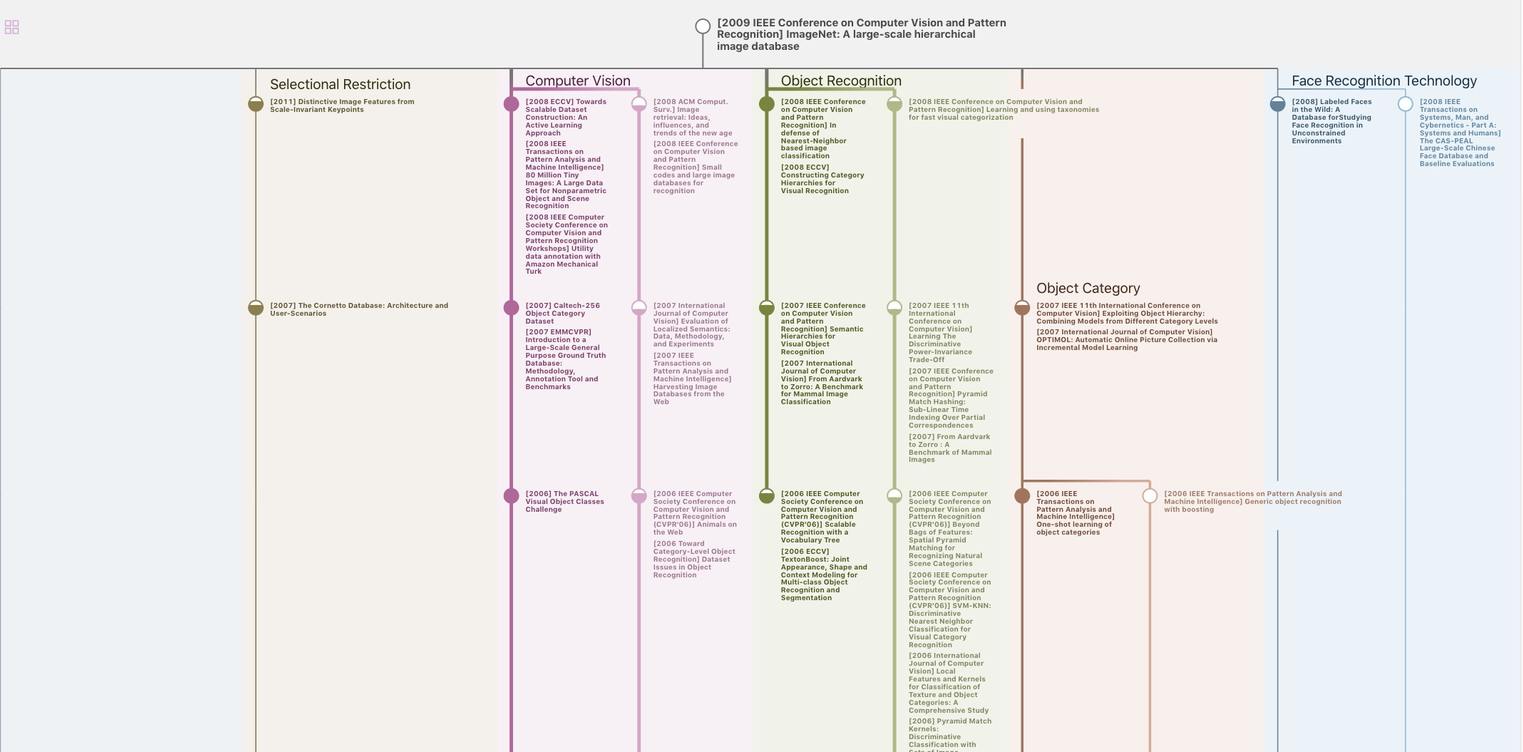
生成溯源树,研究论文发展脉络
Chat Paper
正在生成论文摘要