Efficient Deep-Learning 4D Automotive Radar Odometry Method.
IEEE Trans. Intell. Veh.(2024)
摘要
Odometry is a crucial technology for the autonomous positioning of intelligent vehicles. While estimating the odometry from LiDAR and cameras has progressed recently, it remains to be seen how to estimate the odometry from a 4D radar, an emerging sensor with unique advantages over cameras and LiDAR. In this study, a deep-learning-based 4D radar odometry method, named 4DRO-Net, is proposed. The method employs a coarse-to-fine hierarchical optimization technique based on a sliding window to estimate and refine an autonomous vehicle's pose in an iterative manner. A feature-extraction network for 4D radar point clouds is proposed to achieve efficient learning of sparse point clouds. An initial pose-generation module is constructed to obtain the initial pose, which is used to warp the first point cloud and bridge the distance to the second point cloud. A velocity-aware attention cost volume module is then developed to correlate the warped first point cloud with the second point cloud to obtain point-motion information. The velocity information of the radar points is used to learn the attention weights to increase the robustness of the motion information estimation. Motion information is used to regress the corrected pose, which is then used to refine the initial pose to obtain a more accurate final pose. The superior performance and effectiveness of our 4D radar odometry method are demonstrated on both the View-of-Delft and an in-house dataset.
更多查看译文
关键词
Deep radar odometry,autonomous driving,4D radar
AI 理解论文
溯源树
样例
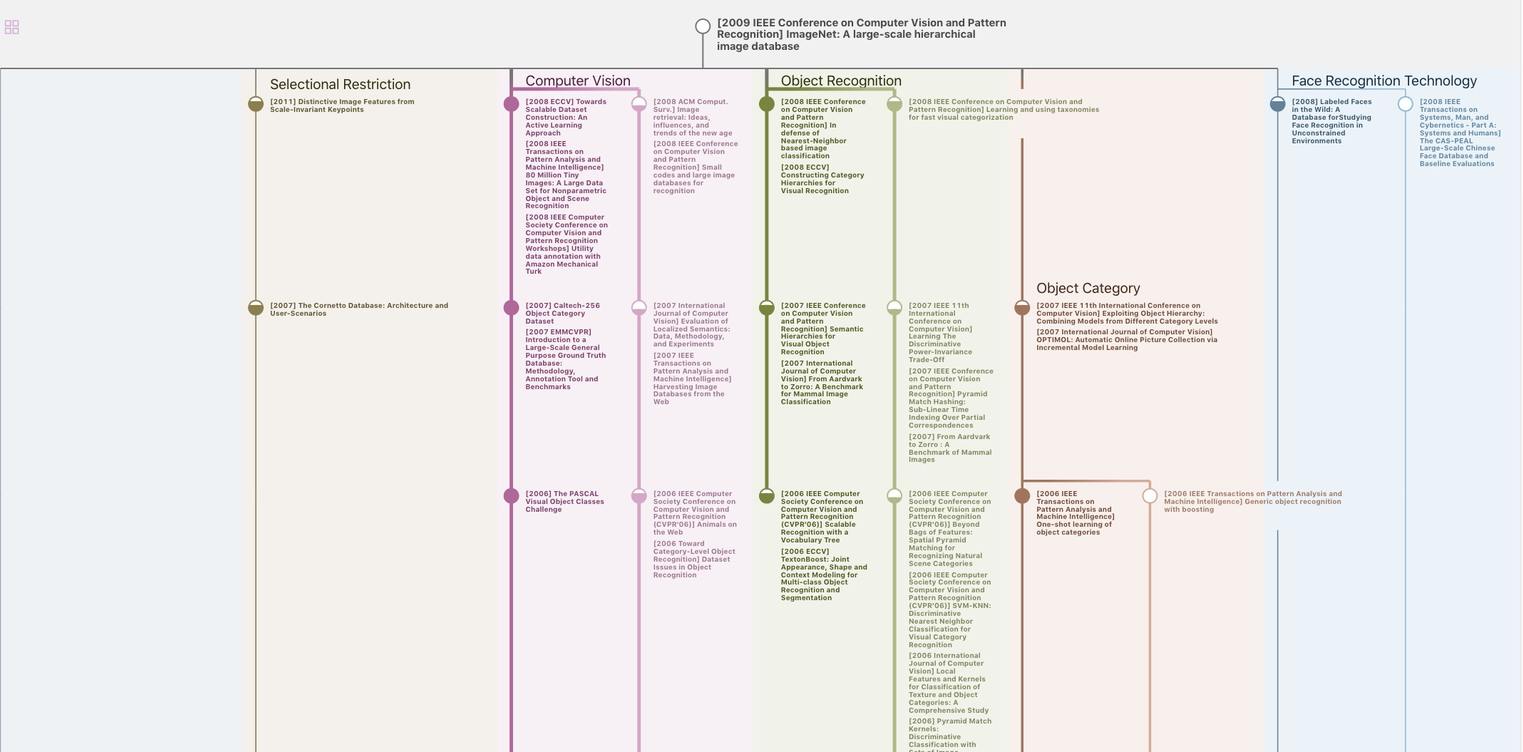
生成溯源树,研究论文发展脉络
Chat Paper
正在生成论文摘要