Dynamic Label Smoothing and Semantic Transport for Unsupervised Domain Adaptation on Object Recognition
IEEE TRANSACTIONS ON CONSUMER ELECTRONICS(2023)
摘要
The application of domain adaptation techniques has emerged as a valuable approach for reducing the cost of data annotation in object recognition domains. Despite its usefulness, domain adaptation is often impeded by domain shift issues that can lead to suboptimal performance. To address this challenge, previous works have attempted to align the global distribution across two domains. However, this approach may not be adequate for handling misalignment near classifier boundaries, which can cause a bias towards the source domain. In this paper, we introduce a novel label-smoothing strategy and semantic transport optimization method for unsupervised domain adaptation. Our approach leverages a memory bank for dynamic smooth rate learning and employs a semantic alignment optimization that treats it as an optimal transport problem. We also integrate class proportions into the optimization to enhance the discriminative ability of target features. To further improve the performance, we incorporate these two strategies into adversarial-based adaptation methods. We conduct comprehensive experiments on three common benchmarks to evaluate the performance of our method. Our results show that our proposed approach achieves competitive performance compared to existing methods. We make our codes publicly available at https://github.com/feifei-cv/DLST.
更多查看译文
关键词
Unsupervised domain adaptation,label smoothing,memory bank,semantic alignment
AI 理解论文
溯源树
样例
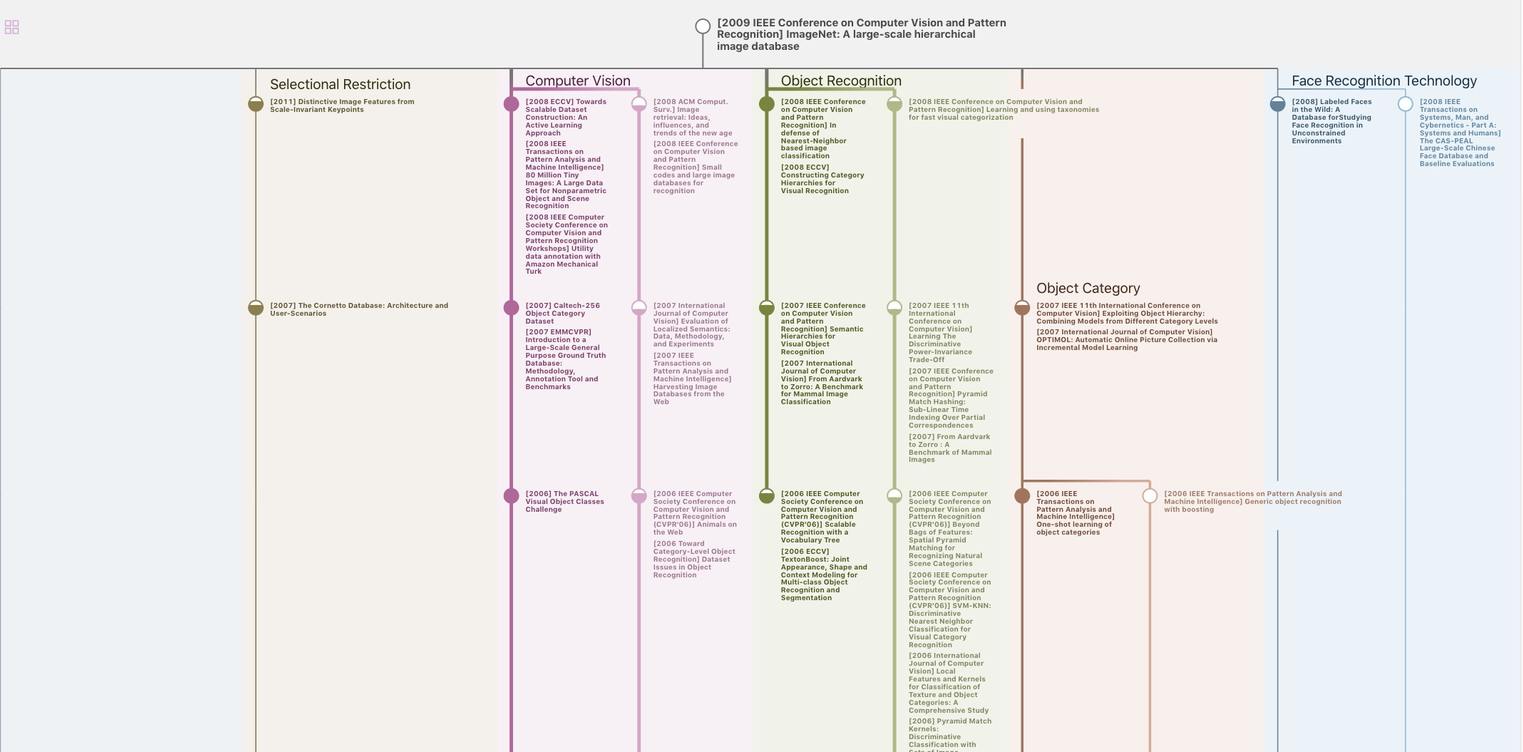
生成溯源树,研究论文发展脉络
Chat Paper
正在生成论文摘要