Utilising machine learning algorithms to predict in vitro fertilisation cycle outcomes with endometrium as a key predictor
ULTRASOUND IN OBSTETRICS & GYNECOLOGY(2023)
摘要
There are many factors that play important roles in the outcome of IVF, such as age, BMI, hormone levels, endometrial thickness, volume and blood flow etc. These different factors make it complex to evaluate the outcome of IVF cycle before implantation. We assume that when relevant predictive features are used as model inputs, machine learning models can be used to predict the implantation result of a single embryo. The purpose of this study is to provide the implantation probability of a single embryo by combining morphological parameters, patient demographics and cycle characteristics. We use various well-known predictive factors as input features of the model, build a new decision support system, and analyse the relative predictive ability of each feature. In the IVF cycle, 19 data were recorded, including patient demographics, IVF cycle characteristics, embryonic morphological data and the number of transferred embryos, and endometrial variables to determine potential features. This study uses a chi-squared testing approach to rank the salience of all features. A new subset of features is formed based on the ranking results of the filtered features and the performance of the deep learning network is combined to determine the optimal features. In this study, the data set was randomly divided into five parts, one for training and validation and one for testing. The deep learning model proposed in this study is a 9-layer structure containing an input layer, an output layer, and 7 hidden layers. A clinical pregnancy prediction model was established using the data of 740 cycles, and the above 19 feature variables. The pregnancy prediction model performed well in the ROC curve, with good prediction accuracy and the area under the ROC curve. Among the 19 feature variables, 9 were endometrial variables. Our study's AI model provides reliable outcome predictions for embryo transplantation, and a network service program for clinical application of the AI prediction model is currently being developed.
更多查看译文
关键词
fertilisation cycle outcomes,endometrium,machine learning,predictor
AI 理解论文
溯源树
样例
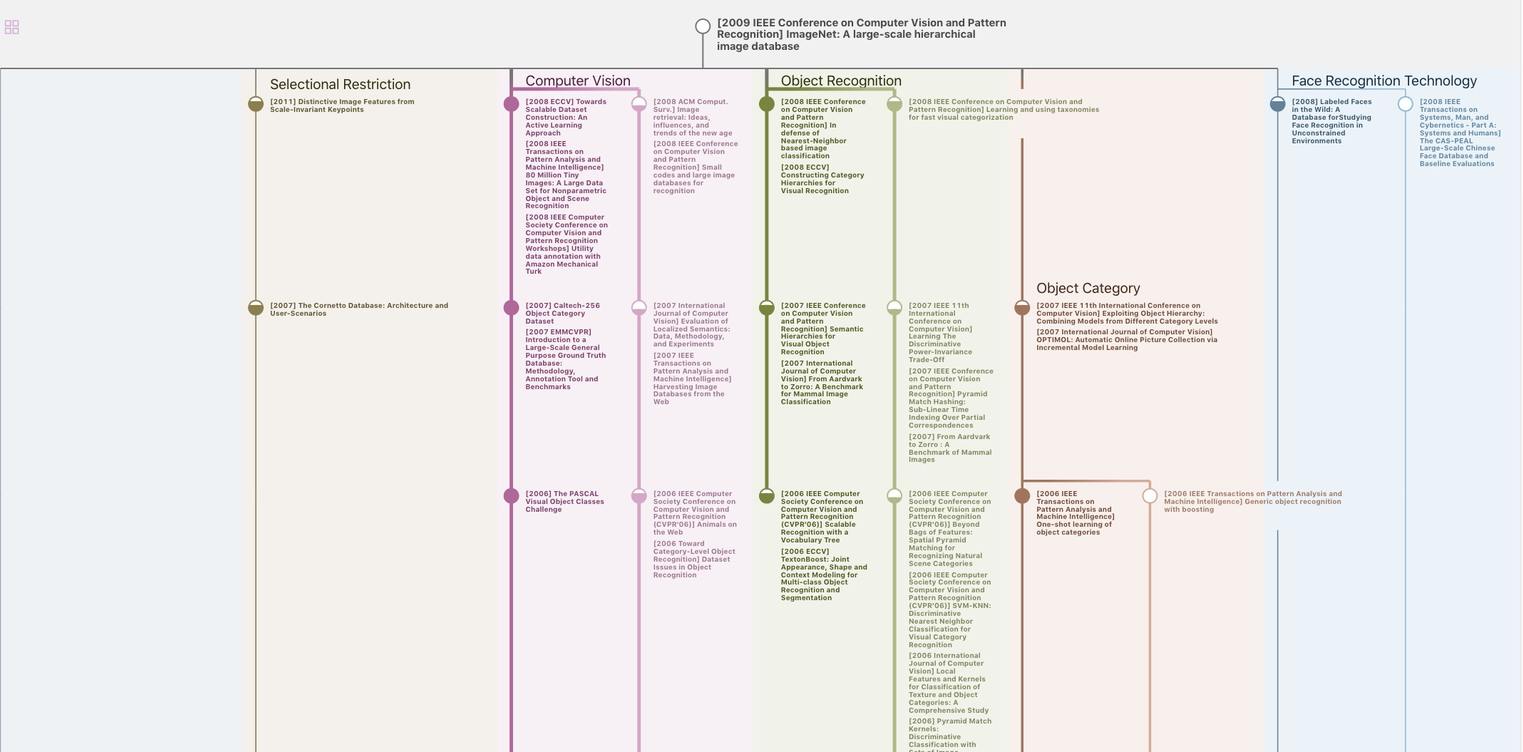
生成溯源树,研究论文发展脉络
Chat Paper
正在生成论文摘要