Stack bricolage and infrastructural impermanence in financial machine-learning modelling
Journal of Cultural Economy(2023)
摘要
ABSTRACTHoping that the promises of machine-learning can be realised in financial markets, investment management and trading firms increasingly employ machine-learning techniques to extract exploitable informational edge from large datasets. In addition to heavy investments in technology and the human resources capable of manipulating it, this development has led to increased use of open-source machine-learning and data-management resources. Drawing on 44 interviews with developers and users of machine-learning techniques in finance, we explore how such platforms and other open-source resources are understood and used by said practitioners. Building on work in the Social Studies of Finance (SSF) on financial modelling and platformisation, we argue that these users of machine learning in finance engage in what we term stack bricolage activities, when they reuse disparate open-source resources in their modelling work. We argue that stack bricolage creates dependencies on open-source cloud resources characterised by infrastructural impermanence, which is a result of their substitutability and maintenance sensitivity. Our study contributes to the emerging SSF literature on machine-learning modelling cultures and debates in Science and Technology Studies and adjacent fields on the reuse of data and software in platformised cloud infrastructures.KEYWORDS: Financial marketsinfrastructural impermanencemachine-learningplatformsreusestack bricolage AcknowledgementWe are grateful to the Editor, Philip Roscoe, Point-editor, Koray Caliskan, and anonymous reviewers of Journal of Cultural Economy for their profound critical engagement with our paper and a very constructive editorial process. We also want to thank Kasper Lindskow, the members of the Technology and Society cluster at the Copenhagen Business School's Department of Management, Society and Communication, Christian Borch, Bo Hee Min, and Daniel Souleles. Finally, we thank Laura Clough for diligent copy-editing.Disclosure statementNo potential conflict of interest was reported by the author(s).Correction StatementThis article has been republished with minor changes. These changes do not impact the academic content of the article.Notes1 Machine learning is a subset of artificial intelligence that enables software to learn patterns from data. It refers to ‘the practice of automating the discovery of rules and patterns from data, however dispersed and heterogeneous it may be, and drawing inferences from those patterns, without explicit programming’ (Fourcade and Johns Citation2020, 804). In short, it comprises a suite of calculative techniques – from simple decision trees to highly complex multi-layered deep artificial neural networks – that ‘all pivot around ways of transforming, constructing or imposing some kind of shape on the data and using that shape to discover, decide, classify, rank, cluster, recommend, label or predict what is happening or what will happen’ in a given problem-context (A. Mackenzie Citation2015, 432; see also, Alpaydin Citation2016). Alternative data, on the other hand, is an umbrella term that covers a wide range of extractable, useable, and potentially useful types of data – sentiment data from social media platforms, sensor data from various devices, satellite imagery, etc. – beyond the standard financial data sources of price, trade, and order book data (Denev and Amen 2020; Hansen and Borch Citation2022).2 While some firms rely on external open-source data and software libraries, others build their own. In-house code libraries are common among financial firms committed to quantitative finance. As a quantitative analyst working in a major UK investment bank noted, ‘unless you are a quant working in a hedge fund developing trading strategies, you are probably working with guys in the back office analyzing risk models and maintaining libraries of code’. The rationale behind in-house code libraries is purely utilitarian, maximising the use or output of the effort put into writing the code and not seeing it go to waste. This idea of sourcing, storing and reactivating knowledge in code libraries contributes to a kind of institutionalisation of stack bricolage activities.3 In their study, MacKenzie and Spears show how copula functions – used by actuarial researchers to model the increased likelihood that the death of one spouse would increase the chances of the death of the other (the so-called ‘broken-heart’ syndrome) –, were adopted and adapted to fit investment banking (D. MacKenzie and Spears Citation2014b, 405). This was interesting knowledge for insurance companies, because accounting for the correlation of the mortality risks of spouses reduced the value of the joint annuity (D. MacKenzie and Spears Citation2014b, 406). Modelling joint survival was made relevant for financial risk management by the statistician, actuarial scientist and investment bank quant, David X. Li, who, in the late 1990s, facilitated the migration of the copula functions from insurance to credit derivatives pricing. Moreover, Li made the translation of the copula modelling of joint survival of spouses to the context of firms’ likelihood of default. He ‘treated a corporation’s default as analogous to a person’s death: the risks of different corporations defaulting were correlated, just as the mortality risks of spouses were, and copula functions could be used to model that correlation’ (D. MacKenzie and Spears Citation2014b, 406).4 The 182 interviews were conducted by Hansen, Daniel Souleles, Christian Borch and Bo Hee Min as part of the research project ‘Algorithmic finance: inquiring into the reshaping of financial markets’ (AlgoFinance). In addition to interviewing, said members of the AlgoFinance research team did participant observation mainly in quantitative investment management firms and proprietary algorithmic trading firms.Additional informationFundingThis work was supported by Danmarks Frie Forskningsfond [9131-00115B]; H2020 European Research Council [725706].
更多查看译文
关键词
infrastructural impermanence,modelling,machine-learning machine-learning
AI 理解论文
溯源树
样例
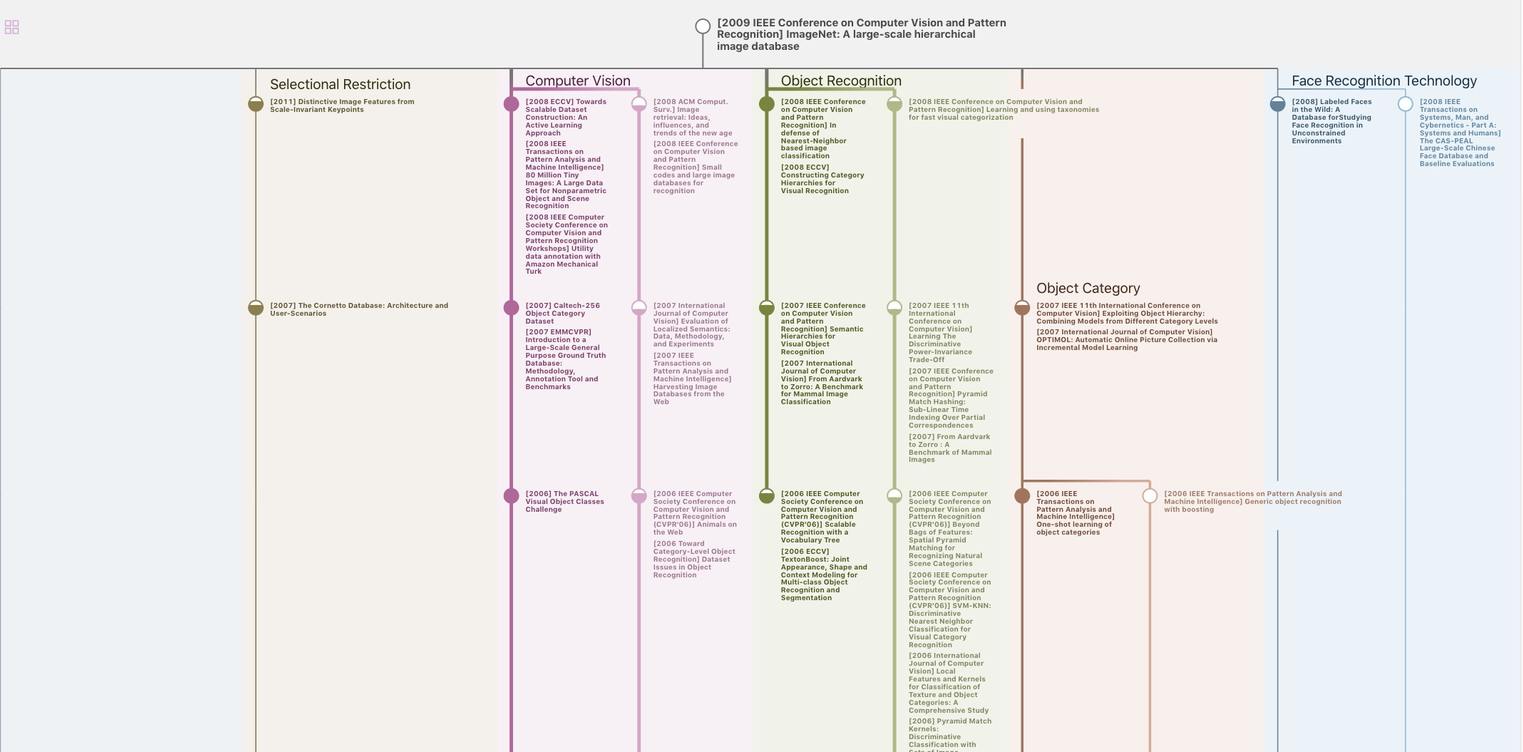
生成溯源树,研究论文发展脉络
Chat Paper
正在生成论文摘要