Imbalanced Few-Shot Learning Based on Meta-transfer Learning
ARTIFICIAL NEURAL NETWORKS AND MACHINE LEARNING, ICANN 2023, PT VIII(2023)
摘要
Few-shot learning is a challenging task that aims to learn to adapt to new tasks with only a few labeled samples. Meta-learning is a promising approach to address this challenge, but the learned meta-knowledge on training sets may not always be useful due to class imbalance, task imbalance, and distribution imbalance. In this paper, we propose a novel few-shot learning method based on meta-transfer learning, which is called Meta-Transfer Task-Adaptive Meta-Learning (MT-TAML). Meta-transfer learning is used to transfer the weight parameters of a pre-trained deep neural network, which makes up for the deficiency of using shallow networks as the feature extractor. To address the imbalance problem in realistic few-shot learning scenarios, we introduce a learnable parameter balance meta-knowledge for each task. Additionally, we propose a novel task training strategy that selects the difficult class in each task and re-samples from it to form the difficult task, thereby improving the model's accuracy. Our experimental results show that MT-TAML outperforms existing few-shot learning methods by 2-4%. Furthermore, our ablation experiments confirm the effectiveness of the combination of meta-transfer learning and learnable equilibrium parameters.
更多查看译文
关键词
meta-learning,few-shot learning,meta-transfer learning
AI 理解论文
溯源树
样例
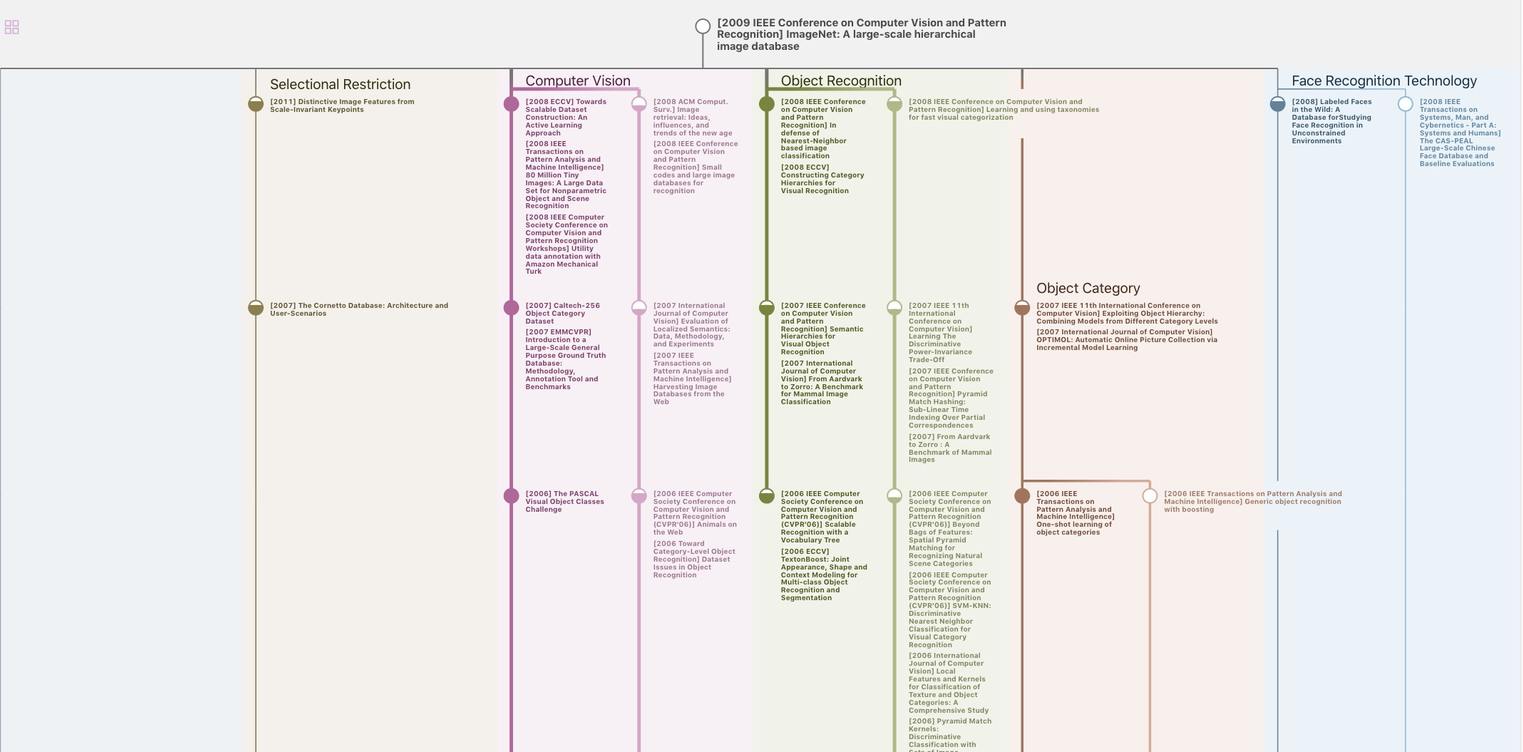
生成溯源树,研究论文发展脉络
Chat Paper
正在生成论文摘要