Functional form or Machine-Learning-Based Ground-Motion Models? An application to the Italian dataset
Research Square (Research Square)(2023)
摘要
Abstract This paper examines the advantages and drawbacks of the use of a functional form in empirical ground-motion modelling compared to machine learning algorithms. Typically, models based on linear regression and predefined functional forms have limits in representing complex nonlinear behaviour of source, attenuation and site effects present in the data. We investigate the efficiency of different machine learning algorithms using the dataset of Italian strong motion records, consisting of 5,607 records relative to 146 earthquakes and 1,657 stations, employed to derive the most recent ground motion model for Italy. We quantify the differences in the predictive capabilities of both approaches in terms of standard deviation, which is broken down into between-event, between-station and event- and site-corrected components, implemented as random effects. When datasets are sufficiently large, various ML algorithms tend to provide more accurate estimates compared to the conventional linear regression-based method and the Gaussian Process Regression is the best-performing algorithm with the Italian dataset. The conventional regression-based methods are a better tool when limited data is available.
更多查看译文
关键词
italian dataset,models,machine-learning-based,ground-motion
AI 理解论文
溯源树
样例
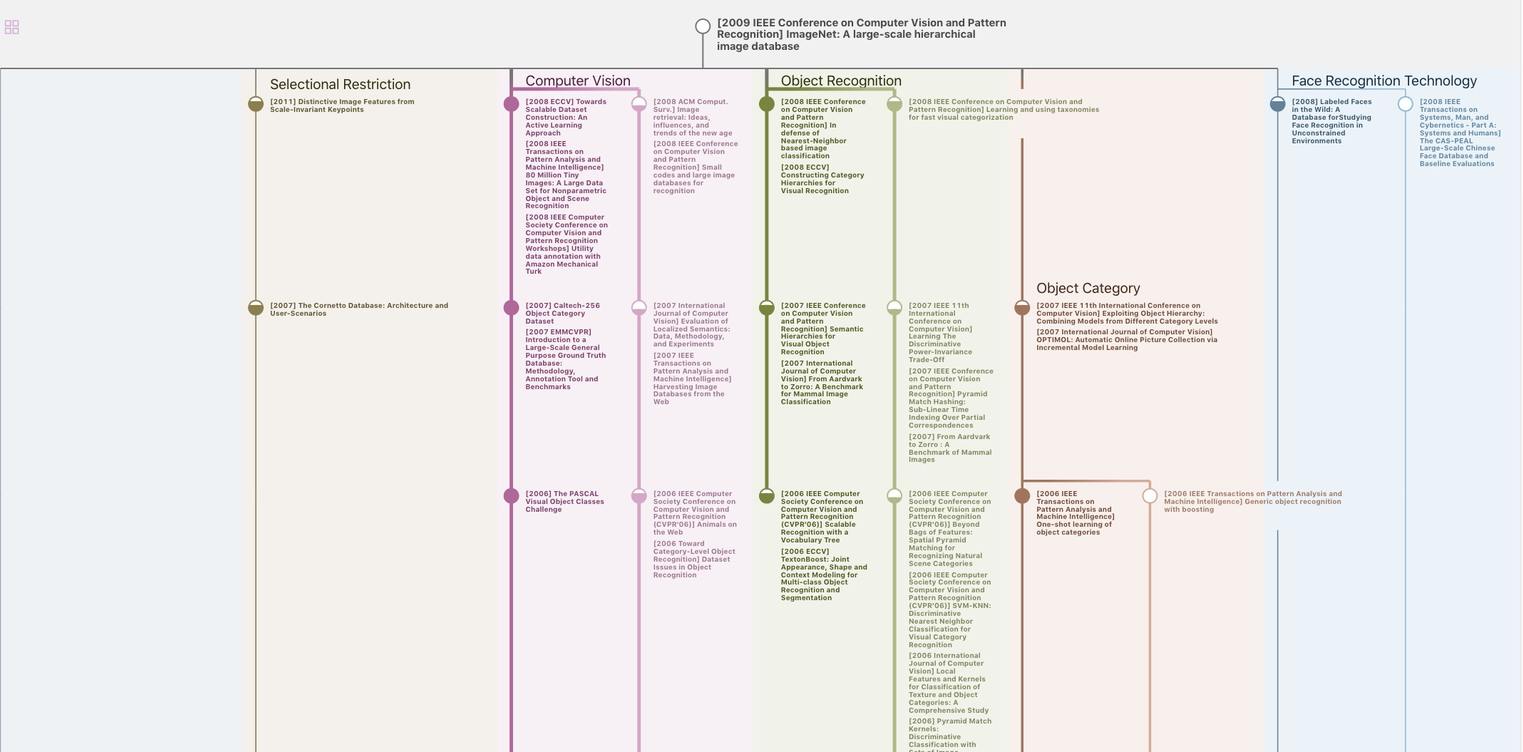
生成溯源树,研究论文发展脉络
Chat Paper
正在生成论文摘要