DDOD: Dive Deeper into the Disentanglement of Object Detector
IEEE TRANSACTIONS ON MULTIMEDIA(2024)
摘要
Compared to many other dense prediction tasks, object detection plays a fundamental role in visual perception and scene understanding. Dense object detection, aiming at localizing objects directly from the feature map, has drawn great attention due to its low cost and high efficiency. Though it has been developed for a long time, the training pipeline of dense object detectors is still compromised to lots of conjunctions. In this paper, we demonstrate the existence of three conjunctions lying in the current paradigm of one-stage detectors: 1) only samples assigned as positive in classification head are used to train the regression head; 2) classification and regression share the same input feature and computational fields defined by the parallel head architecture; and 3) samples distributed in different feature pyramid layers are treated equally when computing the loss. Based on this, we propose Disentangled Dense Object Detector (DDOD), a simple, direct, and efficient framework for 2D detection with strong performance. We derive two DDOD variants (i.e., DR-CNN, and DDETR) following the basic one-stage/two-stage and recently developed transformer-based pipelines. Specifically, we develop three effective disentanglement mechanisms and integrate them into the current state-of-the-art object detectors. Extensive experiments on MS COCO benchmark show that our approach obtains significant enhancements with negligible extra overhead on various detectors. Notably, our best model reaches 55.4 mAP on the COCO test-dev set, achieving new state-of-the-art performance on this competitive benchmark. Additionally, we validate our model on several challenging tasks including small object detection and crowded object detection. The experimental results further prove the superiority of disentanglement on these conjunctions.
更多查看译文
关键词
Disentanglement,feature representation learning,imbalance learning,object detection
AI 理解论文
溯源树
样例
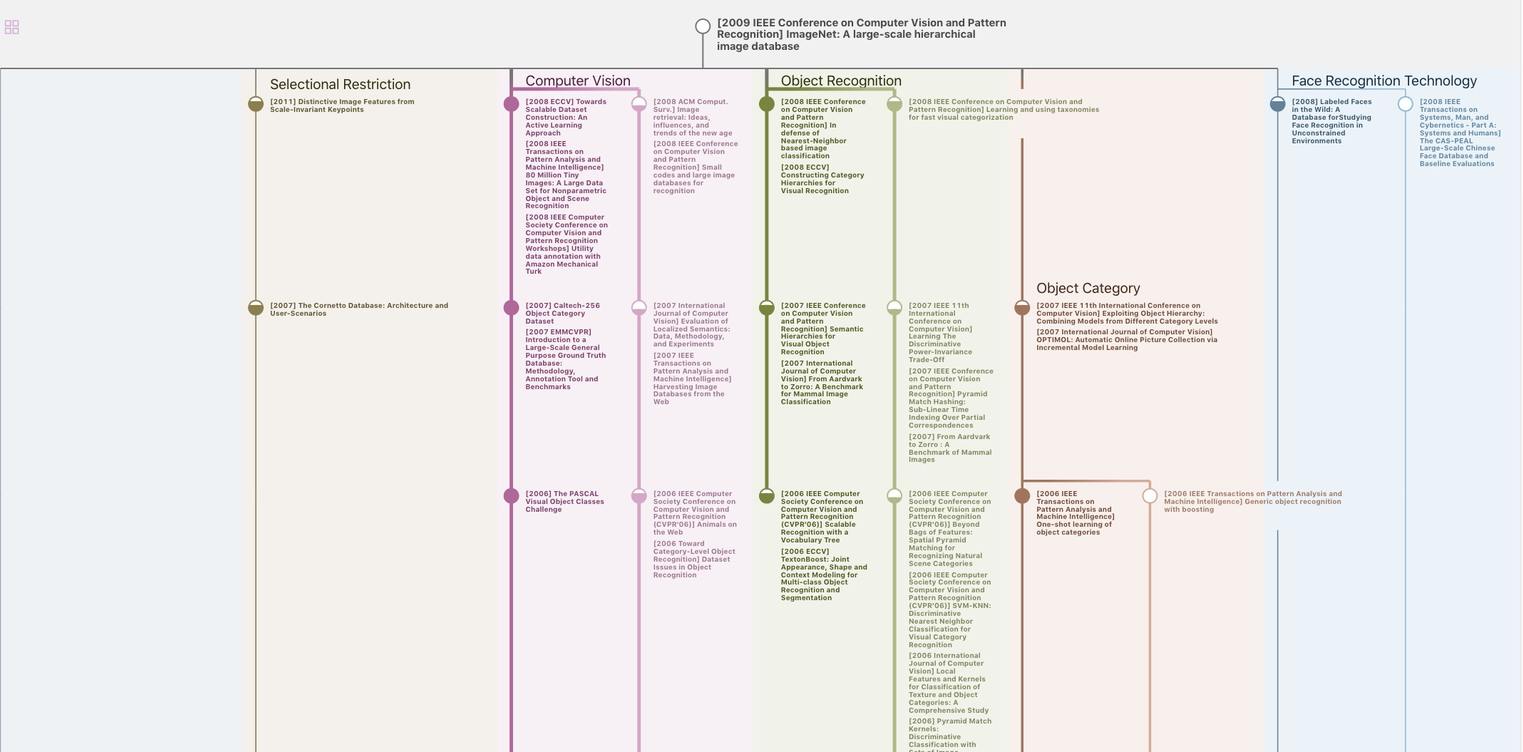
生成溯源树,研究论文发展脉络
Chat Paper
正在生成论文摘要