Stochastic trust-region and direct-search methods: A weak tail bound condition and reduced sample sizing
arXiv (Cornell University)(2022)
摘要
Using tail bounds, we introduce a new probabilistic condition for function estimation in stochastic derivative-free optimization which leads to a reduction in the number of samples and eases algorithmic analyses. Moreover, we develop simple stochastic direct-search and trust-region methods for the optimization of a potentially non-smooth function whose values can only be estimated via stochastic observations. For trial points to be accepted, these algorithms require the estimated function values to yield a sufficient decrease measured in terms of a power larger than 1 of the algoritmic stepsize. Our new tail bound condition is precisely imposed on the reduction estimate used to achieve such a sufficient decrease. This condition allows us to select the stepsize power used for sufficient decrease in such a way to reduce the number of samples needed per iteration. In previous works, the number of samples necessary for global convergence at every iteration $k$ of this type of algorithms was $O(\Delta_{k}^{-4})$, where $\Delta_k$ is the stepsize or trust-region radius. However, using the new tail bound condition, and under mild assumptions on the noise, one can prove that such a number of samples is only $O(\Delta_k^{-2 - \varepsilon})$, where $\varepsilon > 0$ can be made arbitrarily small by selecting the power of the stepsize in the sufficient decrease test arbitrarily close to $1$. The global convergence properties of the stochastic direct-search and trust-region algorithms are established under the new tail bound condition.
更多查看译文
关键词
trust-region,direct-search
AI 理解论文
溯源树
样例
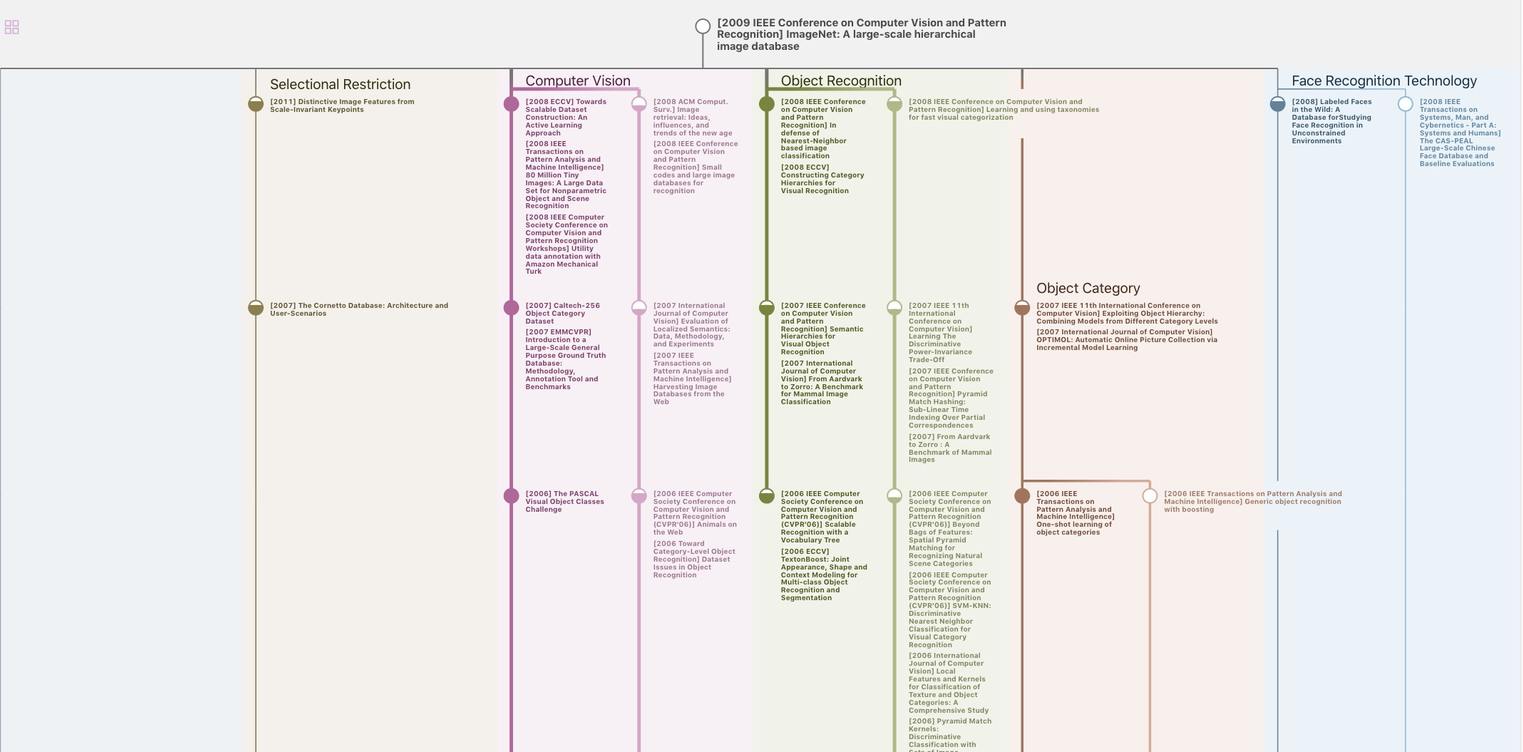
生成溯源树,研究论文发展脉络
Chat Paper
正在生成论文摘要