Recognizing pathology of renal tumor from macroscopic cross-section image by deep learning
Research Square (Research Square)(2022)
摘要
Abstract Objectives : This study aims to develop and evaluate the deep learning-based classification model for recognizing the pathology of renal tumor from macroscopic cross-section image. Methods : A total of 467 pathology-confirmed patients who received radical nephrectomy or partial nephrectomy were retrospectively enrolled. The experiment of distinguishing malignant and benign renal tumor are conducted followed by performing the multi-subtypes classification models for recognizing four subtypes of benign tumor and four subtypes of malignant tumorsrespectively. The classification models used the same backbone networks which are based on the convolutional neural network (CNN), including EfficientNet-B4, ResNet-18 and VGG-16. The performance of the classification models was evaluated by area under the receiver operating characteristic curve (AUC),sensitivity, specificity and accuracy. Besides, we performed the quantitative comparison among these CNN models. Results : For the model to differentiate the malignant tumor from the benign tumor, three CNN models all obtained relatively satisfactory performance and the highest AUC was achieved by the ResNet-18 model (AUC=0.9226). There is not statistically significance between EfficientNet-B4 and ResNet-18 architectures and both of them are significantly statistically better than the VGG-16 model. The micro-averaged AUC, macro-averaged sensitivity, macro-averaged specificity and micro-averaged accuracy for the VGG-16 model to distinguish the malignant tumor subtypes achieved 0.9398, 0.5774, 0.8660 and 0.7917 respectively. The performance of the EfficientNet-B4 is not better than that of VGG-16 in terms of micro-averaged AUC except for other metrics. For the models to recognize the benign tumor subtypes, the EfficientNet-B4 ranked the best performance but had not significantly statistical difference with other two models with respect to micro-averaged AUC. Conclusions : The classification results were relatively satisfactory, which showed the potential for clinical application when analyzing the renal tumor macroscopic cross-section images. Automatically distinguishing the malignant tumor from benign tumor and identifying the subtypes pathology of renal tumor could make the patient-management process more efficient.
更多查看译文
关键词
renal tumor,deep learning,pathology,cross-section
AI 理解论文
溯源树
样例
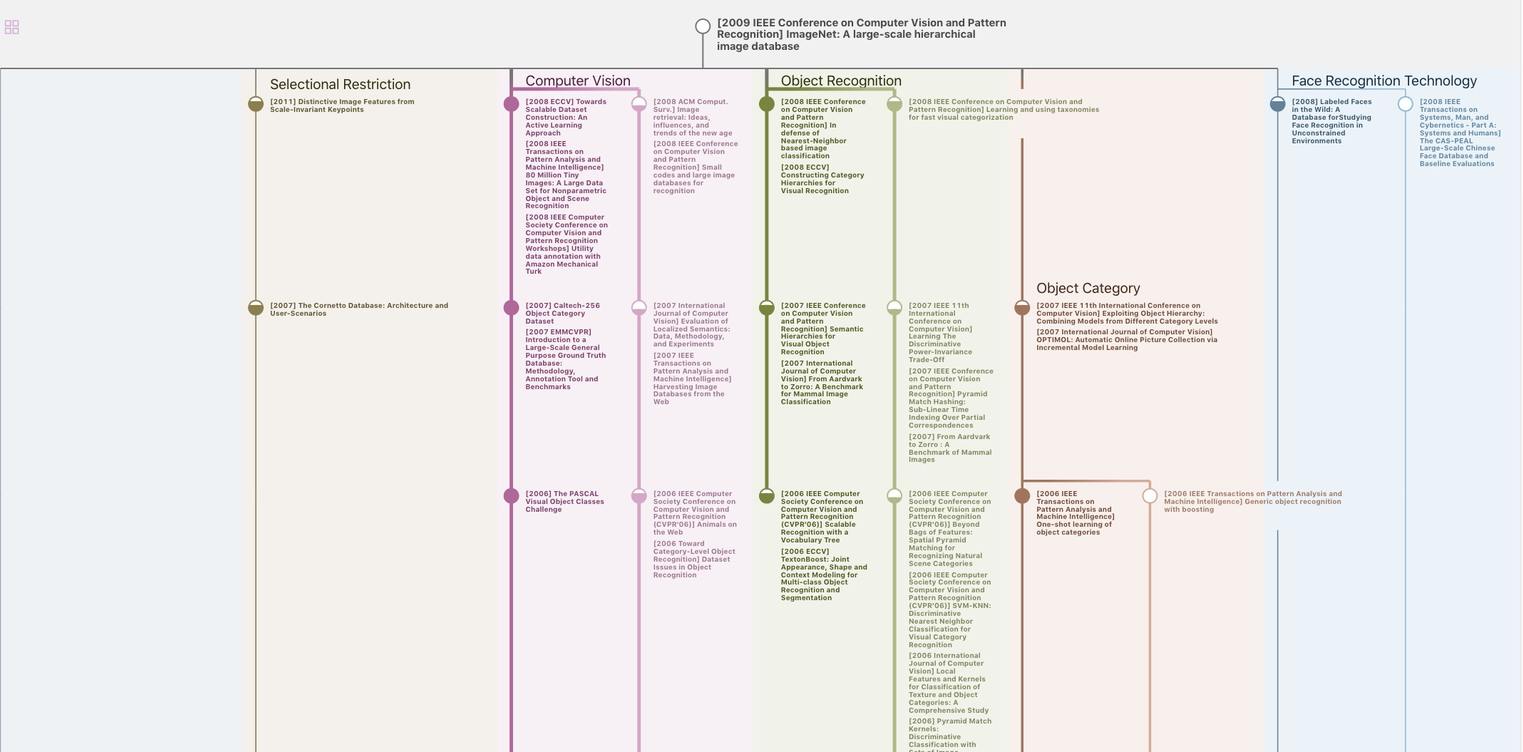
生成溯源树,研究论文发展脉络
Chat Paper
正在生成论文摘要