Diversity Over Size: On the Effect of Sample and Topic Sizes for Argument Mining Datasets
arXiv (Cornell University)(2022)
摘要
The task of Argument Mining, that is extracting argumentative sentences for a specific topic from large document sources, is an inherently difficult task for machine learning models and humans alike, as large Argument Mining datasets are rare and recognition of argumentative sentences requires expert knowledge. The task becomes even more difficult if it also involves stance detection of retrieved arguments. Given the cost and complexity of creating suitably large Argument Mining datasets, we ask whether it is necessary for acceptable performance to have datasets growing in size. Our findings show that, when using carefully composed training samples and a model pretrained on related tasks, we can reach 95% of the maximum performance while reducing the training sample size by at least 85%. This gain is consistent across three Argument Mining tasks on three different datasets. We also publish a new dataset for future benchmarking.
更多查看译文
关键词
argument mining datasets,topic sizes,diversity,sample
AI 理解论文
溯源树
样例
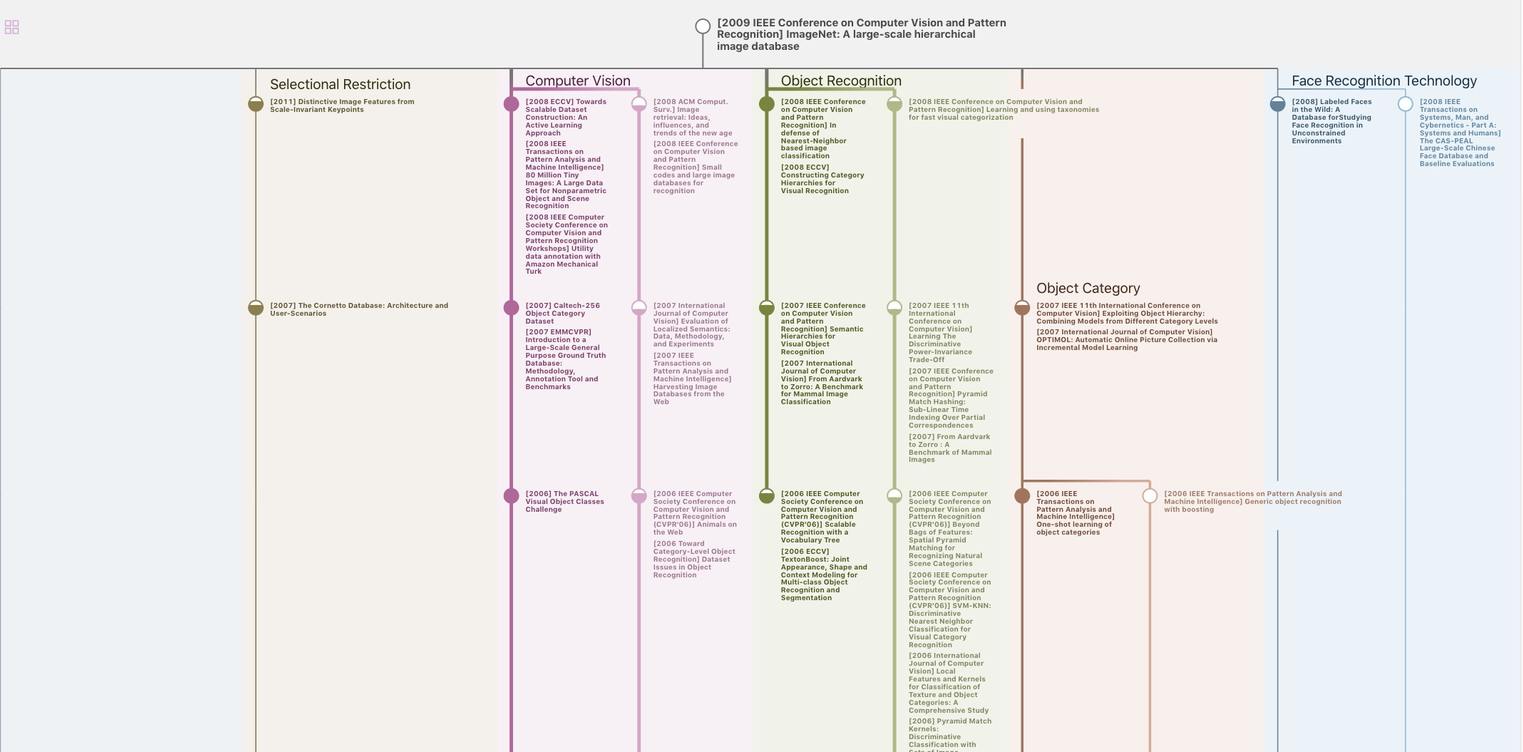
生成溯源树,研究论文发展脉络
Chat Paper
正在生成论文摘要