Causal Inference with Orthogonalized Regression Adjustment: Taming the Phantom
arXiv (Cornell University)(2022)
摘要
Standard regression adjustment gives inconsistent estimates of causal effects when there are time-varying treatment effects and time-varying covariates. Loosely speaking, the issue is that some covariates are post-treatment variables because they may be affected by prior treatment status, and regressing out post-treatment variables causes bias. More precisely, the bias is due to certain non-confounding latent variables that create colliders in the causal graph. These latent variables, which we call phantoms, do not harm the identifiability of the causal effect, but they render naive regression estimates inconsistent. Motivated by this, we ask: how can we modify regression methods so that they hold up even in the presence of phantoms? We develop an estimator for this setting based on regression modeling (linear, log-linear, probit and Cox regression), proving that it is consistent for the causal effect of interest. In particular, the estimator is a regression model fit with a simple adjustment for collinearity, making it easy to understand and implement with standard regression software. From a causal point of view, the proposed estimator is an instance of the parametric g-formula. Importantly, we show that our estimator is immune to the null paradox that plagues most other parametric g-formula methods.
更多查看译文
关键词
orthogonalized regression adjustment,phantom
AI 理解论文
溯源树
样例
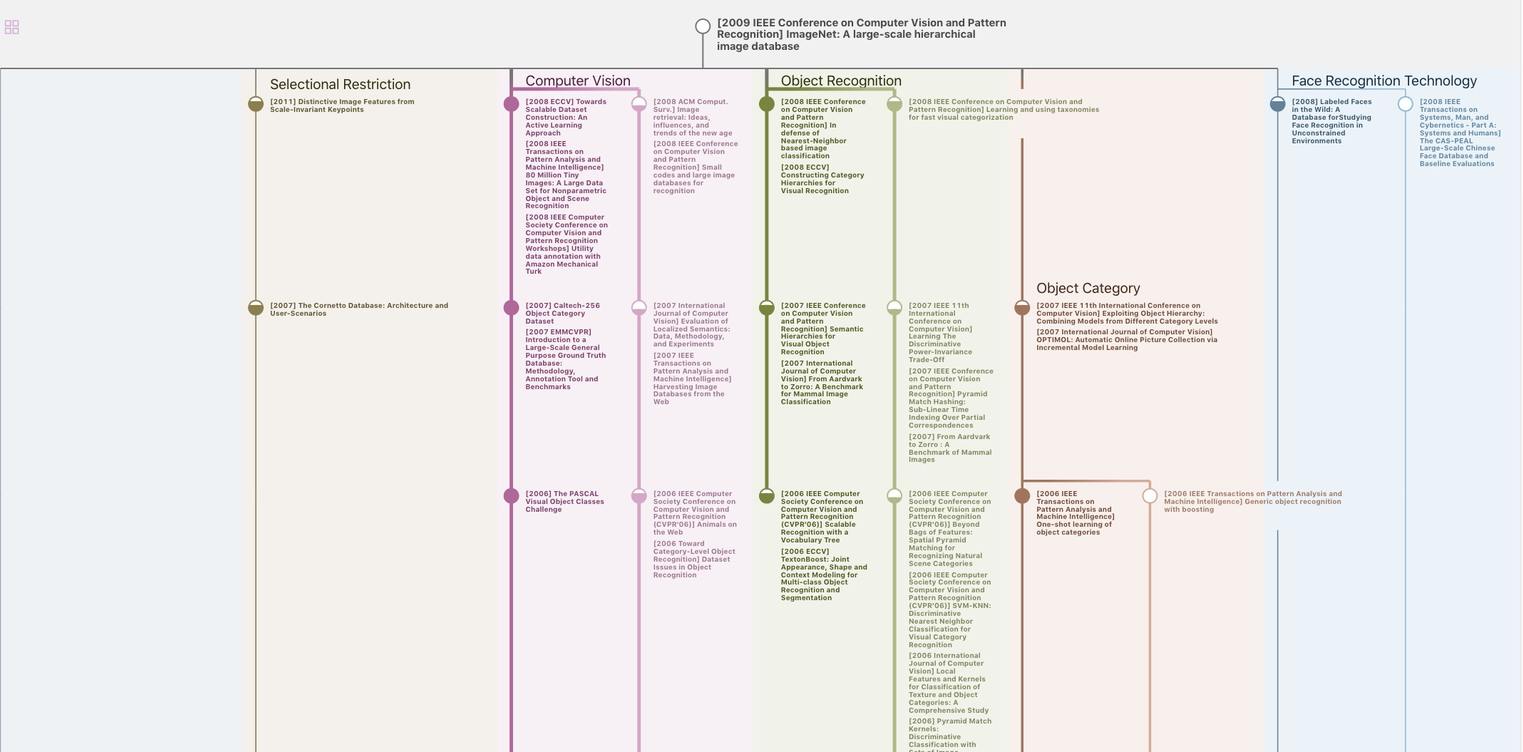
生成溯源树,研究论文发展脉络
Chat Paper
正在生成论文摘要