Mask Atari for Deep Reinforcement Learning as POMDP Benchmarks
arXiv (Cornell University)(2022)
摘要
We present Mask Atari, a new benchmark to help solve partially observable Markov decision process (POMDP) problems with Deep Reinforcement Learning (DRL)-based approaches. To achieve a simulation environment for the POMDP problems, Mask Atari is constructed based on Atari 2600 games with controllable, moveable, and learnable masks as the observation area for the target agent, especially with the active information gathering (AIG) setting in POMDPs. Given that one does not yet exist, Mask Atari provides a challenging, efficient benchmark for evaluating the methods that focus on the above problem. Moreover, the mask operation is a trial for introducing the receptive field in the human vision system into a simulation environment for an agent, which means the evaluations are not biased from the sensing ability and purely focus on the cognitive performance of the methods when compared with the human baseline. We describe the challenges and features of our benchmark and evaluate several baselines with Mask Atari.
更多查看译文
关键词
deep reinforcement learning,atari
AI 理解论文
溯源树
样例
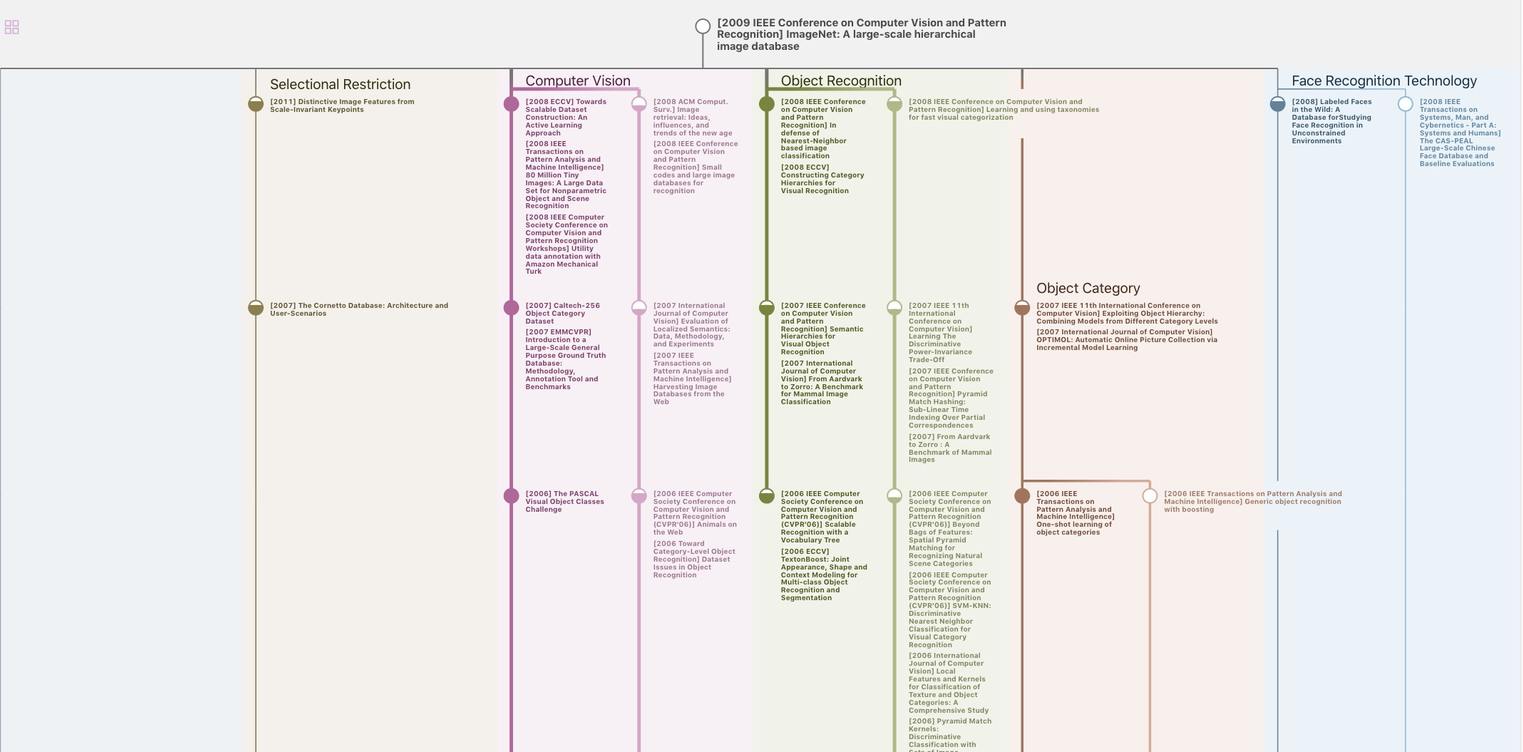
生成溯源树,研究论文发展脉络
Chat Paper
正在生成论文摘要