Scheduling Real-time Deep Learning Services as Imprecise Computations
arXiv (Cornell University)(2020)
Key words
scheduling,deep learning,services,real-time
AI Read Science
Must-Reading Tree
Example
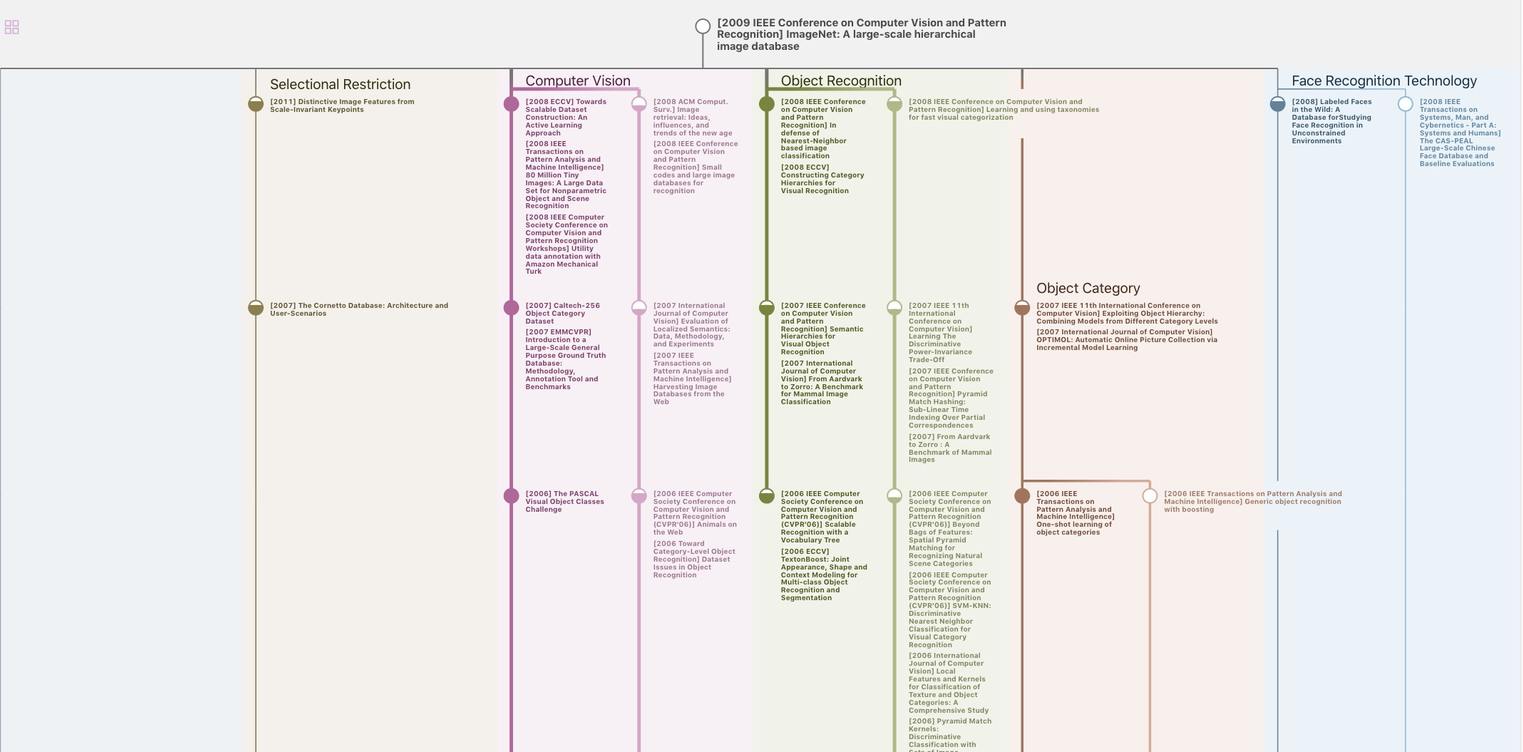
Generate MRT to find the research sequence of this paper
Chat Paper
Summary is being generated by the instructions you defined