A computationally efficient sequential regression imputation algorithm for multilevel data
JOURNAL OF APPLIED STATISTICS(2023)
摘要
Due to the computational burden, especially in high-dimensional settings, sequential imputation may not be practical. In this paper, we adopt computationally advantageous methods by sampling the missing data from their perspective predictive distributions, which leads to significantly improved computation time in the class of variable-by-variable imputation algorithms. We assess the computational performance in a comprehensive simulation study. We then compare and contrast the performance of our algorithm with commonly used alternatives. The results show that our method has a significant advantage over the commonly used alternatives with respect to computational efficiency and inferential quality. Finally, we demonstrate our methods in a substantive problem aimed at investigating the effects of area-level behavioral, socioeconomic, and demographic characteristics on poor birth outcomes in New York State among singleton births.
更多查看译文
关键词
Sequential regression imputation,multilevel data,computational efficiency,fast variable by variable imputation,multiple imputation by chained equations
AI 理解论文
溯源树
样例
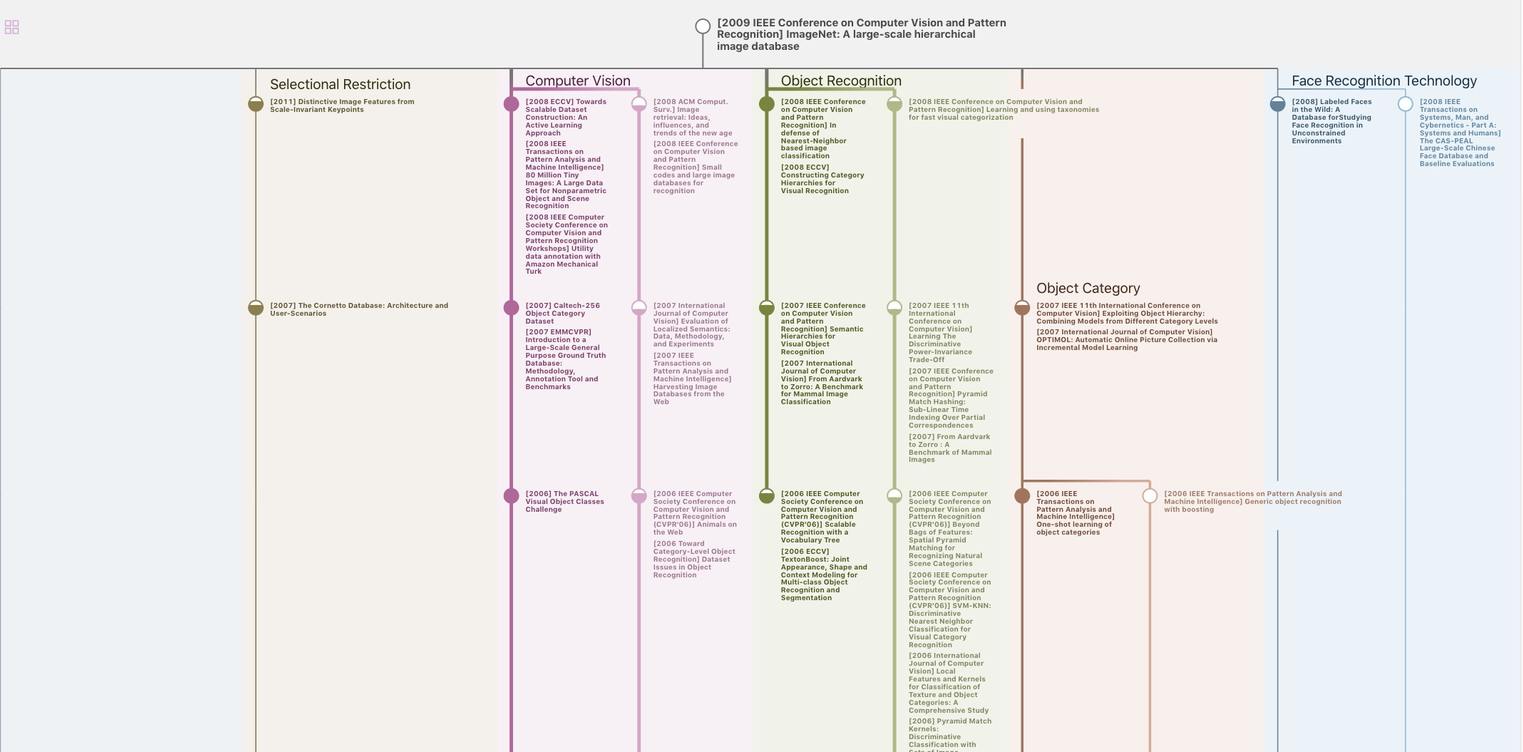
生成溯源树,研究论文发展脉络
Chat Paper
正在生成论文摘要