Wake and Power Prediction of Horizontal-Axis Wind Farm under Yaw-Controlled Conditions with Machine Learning
ENERGY CONVERSION AND MANAGEMENT(2023)
Key words
Wind farm,XGBoost,Yaw control,Power estimation,Machine learning
AI Read Science
Must-Reading Tree
Example
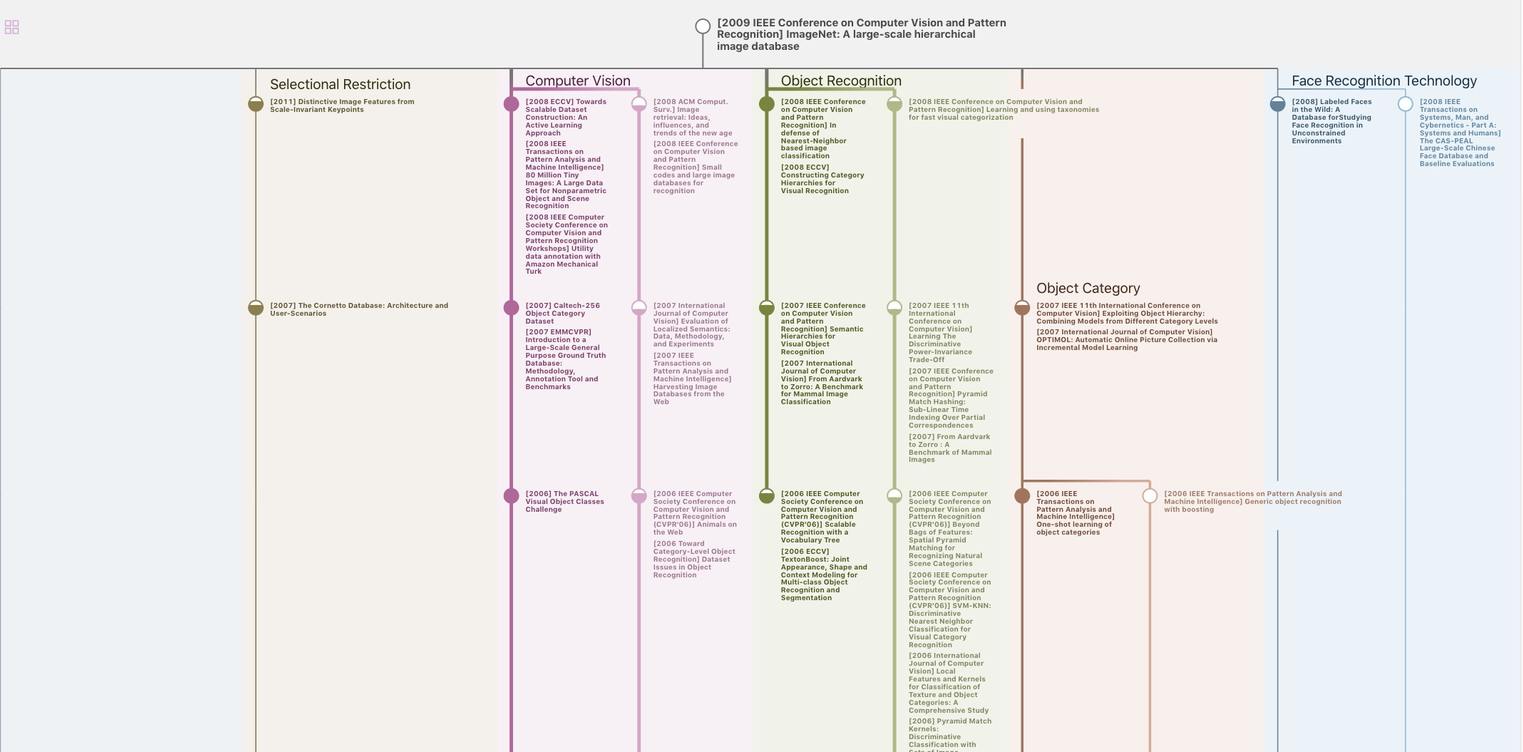
Generate MRT to find the research sequence of this paper
Chat Paper
Summary is being generated by the instructions you defined