Quantitative evaluation of collector flotation performance II: The creation of a collector property index based on molecular structure
SEPARATION AND PURIFICATION TECHNOLOGY(2024)
摘要
The evaluation and prediction of flotation performance is a key step in the development of high-performance collectors for the efficient flotation separation of low-grade complex ores. In our previous work, we have created the flotation index (FI) for a comprehensive and standardized evaluation of collector flotation performance. However, as an experiment-based index, FI does not possess the quantitative predictive power that is fundamental to rational flotation reagent design. In this work, the collector properties were obtained by quantum chemistry (QC) calculation. By ranking the importance of the QC properties, machine learning (ML) aids the selection of key properties representing different aspects of a collector (polar, non-polar and overall polarity). The key properties were incorporated into a theory-based collector property index (CPI). Based on a quantitative structure-activity relationship (QSAR), the bridge between theory and experiment were established by searching for the relationship CPI and FI, enabling convenient evaluation and prediction of collector flotation performance. The accuracy of our QSAR model was verified using the galena-pyrite separation system. Our QSAR model demonstrates transferability in that it can predict collectors with diverse skeletons or types, pushing the limit of most conventional QSAR models, which only apply to collectors with similar skeletons or types. This work provides an alternative pathway for the rational design and performance prediction of flotation surfactants.
更多查看译文
关键词
Collector flotation performance,Sulfide mineral,Quantitative structure -activity relationship (QSAR),Machine learning (ML),Quantum chemistry (QC)
AI 理解论文
溯源树
样例
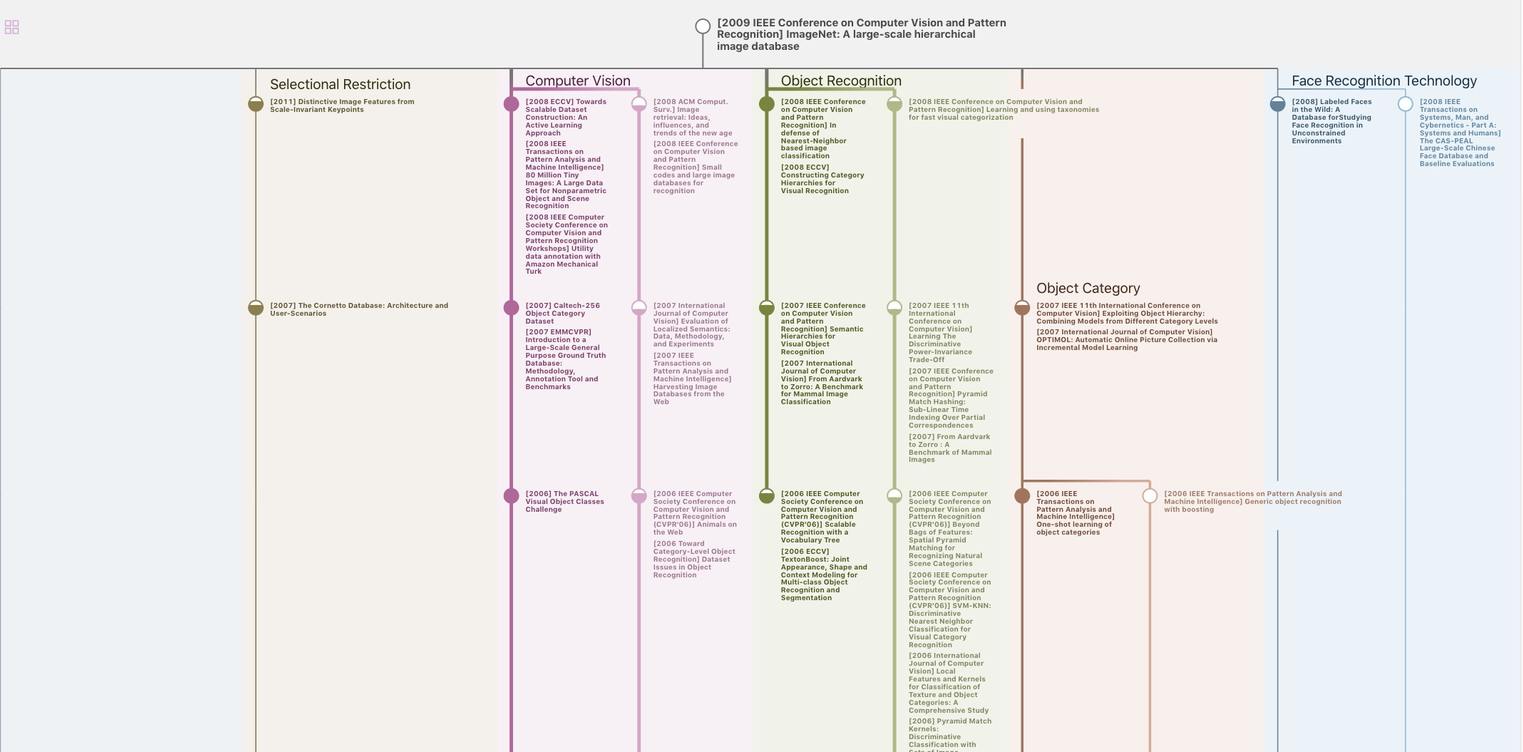
生成溯源树,研究论文发展脉络
Chat Paper
正在生成论文摘要