De-accumulated error collaborative learning framework for predicting Alzheimer's disease progression
BIOMEDICAL SIGNAL PROCESSING AND CONTROL(2024)
摘要
Alzheimer's disease (AD) is a chronic neurodegenerative disorder where precise prediction of progression is crucial for improving clinical diagnosis. However, missing data often complicates AD prediction in clinical practice. Existing studies primarily have employed recurrent neural networks to impute missing data, but this approach suffers from the "error accumulation"problem, leading to unsatisfactory prediction results. To address this issue, this study proposes the LSTM-TSGAIN collaborative learning framework, which consists of the following three main aspects: using a generative adversarial imputation method to reduce LSTM prediction errors; collaborative training of the adversarial imputation fusion module and the time series learning module to improve the performance of the model; the model inputs were adjusted to variable lengths to accommodate the differences in the number of visits of different subjects. The effectiveness of the model is demonstrated through experiments using longitudinal data from the ADNI dataset of 1256 subjects, which shows its superiority over state-of-the-art methods.
更多查看译文
关键词
Alzheimer's disease,Imputation of missing data,Disease progression prediction,Reducing error accumulation,Collaborative learning framework
AI 理解论文
溯源树
样例
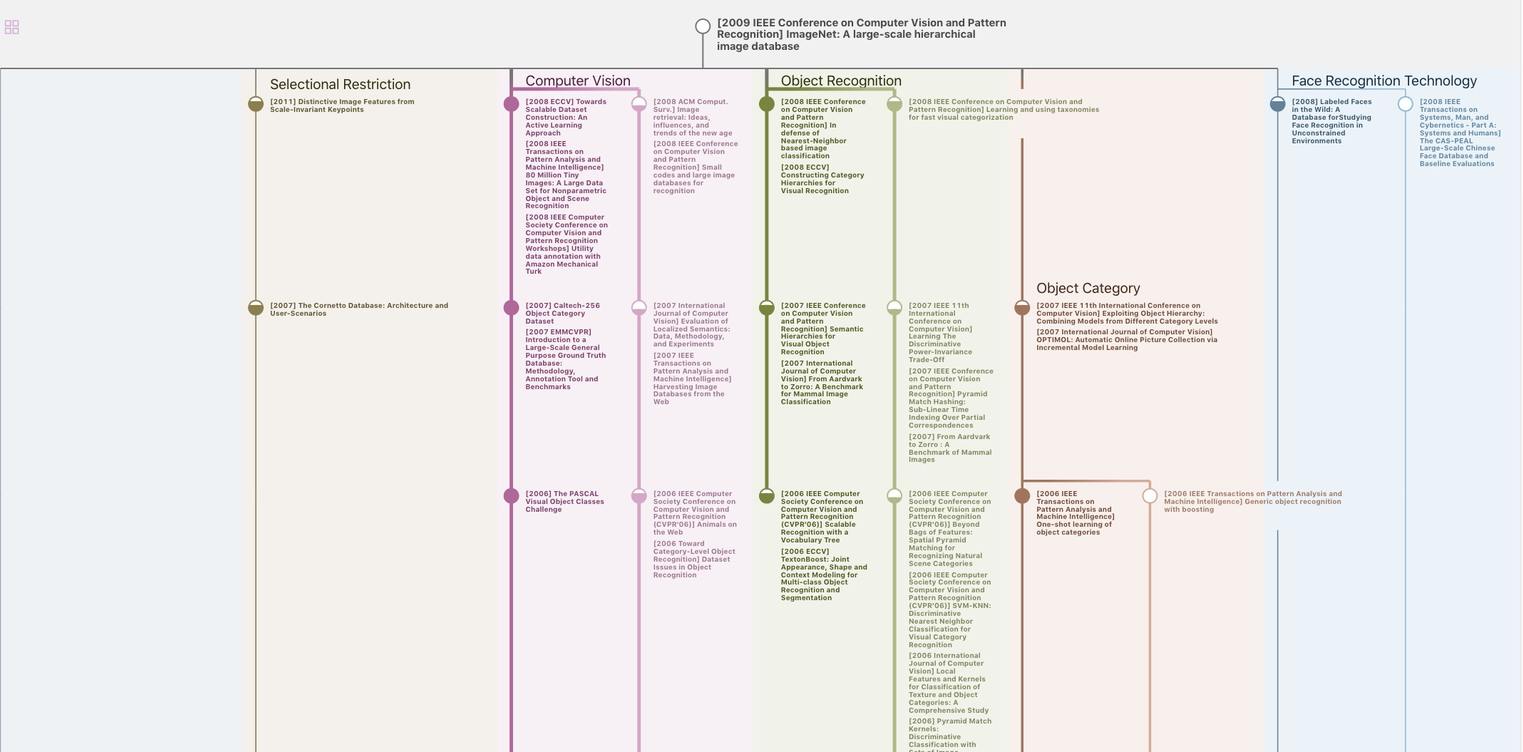
生成溯源树,研究论文发展脉络
Chat Paper
正在生成论文摘要