Discovering Symbolic Policy for Building Control using Reinforcement Learning
IFAC-PapersOnLine(2023)
摘要
We propose a learning framework for interpretable HVAC control in buildings using deep reinforcement learning (DRL). Our framework includes a data-driven surrogate environment to emulate building dynamics and a Deep Symbolic Policy for discovering interpretable control policies. We focus on maintaining the temperature within the desired range for occupant comfort. Our results show that the discovered symbolic policies are interpretable and perform well compared to standard DRL algorithms. Additionally, the discovered policies in surrogate models exhibit transferability to physics-based environments with minimal performance degradation.
更多查看译文
关键词
Deep Reinforcement Learning,Symbolic Policies,Transfer Learning,Building Control
AI 理解论文
溯源树
样例
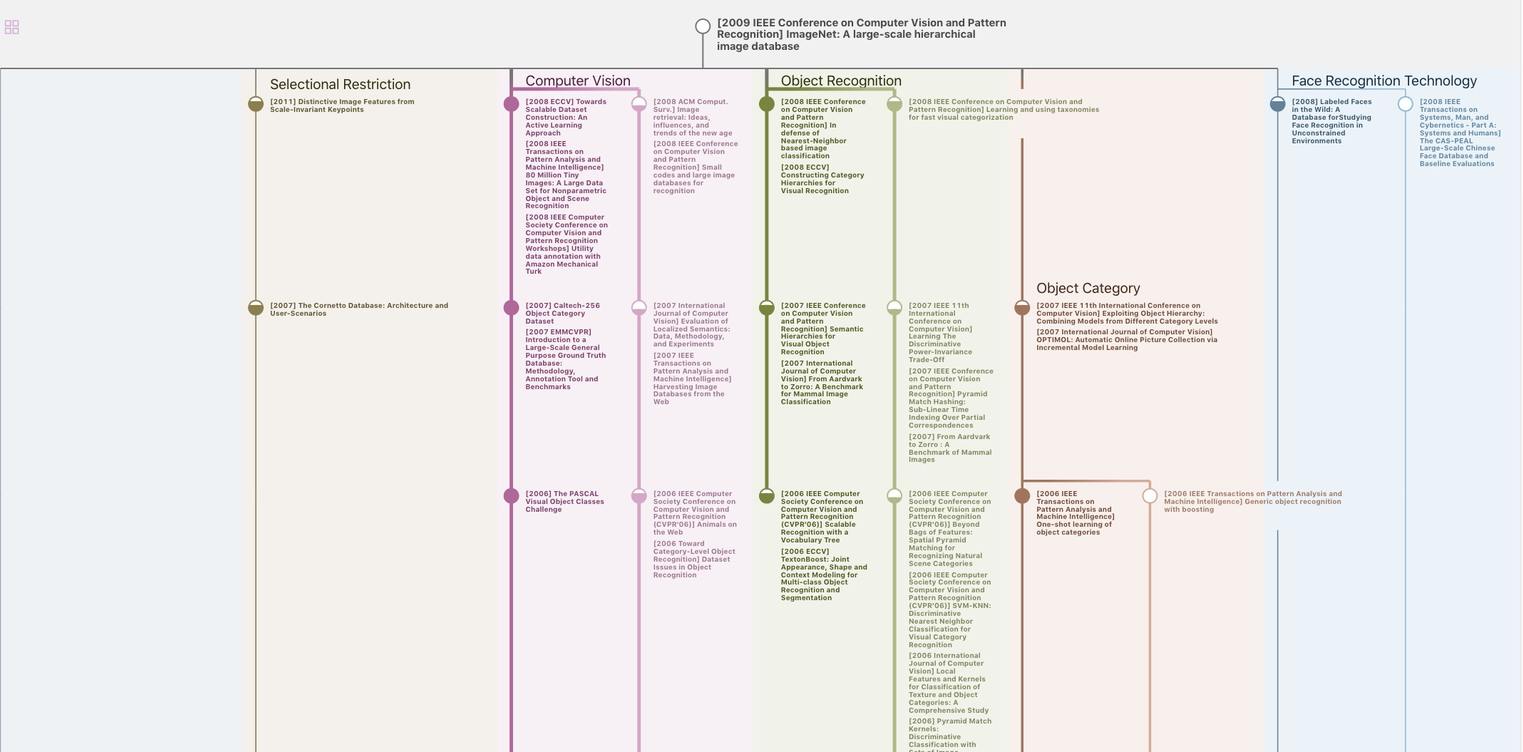
生成溯源树,研究论文发展脉络
Chat Paper
正在生成论文摘要