A new multi-view multi-label model with privileged information learning
INFORMATION SCIENCES(2024)
摘要
In multi-view multi-label learning (MVML), the data is described by multiple feature views and annotated by a number of categorical labels. At present, most of the existing MVML methods are proposed based on subspace learning, neural networks and so on. There is little work done on support vector machine (SVM)-based MVML. In this paper, we propose a novel SVM-based multi-view multi-label learning method with privileged information learning (MVMLP). By introducing the idea of privileged information learning, MVMLP implements both the consensus principle and complementarity principle in MVML. Specifically, we enforce similarity between the outputs of different views to explore the consensus information. Moreover, we allow different views to serve as privileged information for each other, such that the complementary information among distinct views can be introduced into the training process. MVMLP constructs an SVM-based model for each view and trains the models of multiple views jointly. The derived learning problem can be efficiently solved by adapting the Franke-Wolfe algorithm. Experimental results on real-life datasets show that MVMLP delivers explicitly better classification performance than state-of-the-art MVML methods.
更多查看译文
关键词
Multi-label learning,Multi-view learning,Privileged information,Support vector machine
AI 理解论文
溯源树
样例
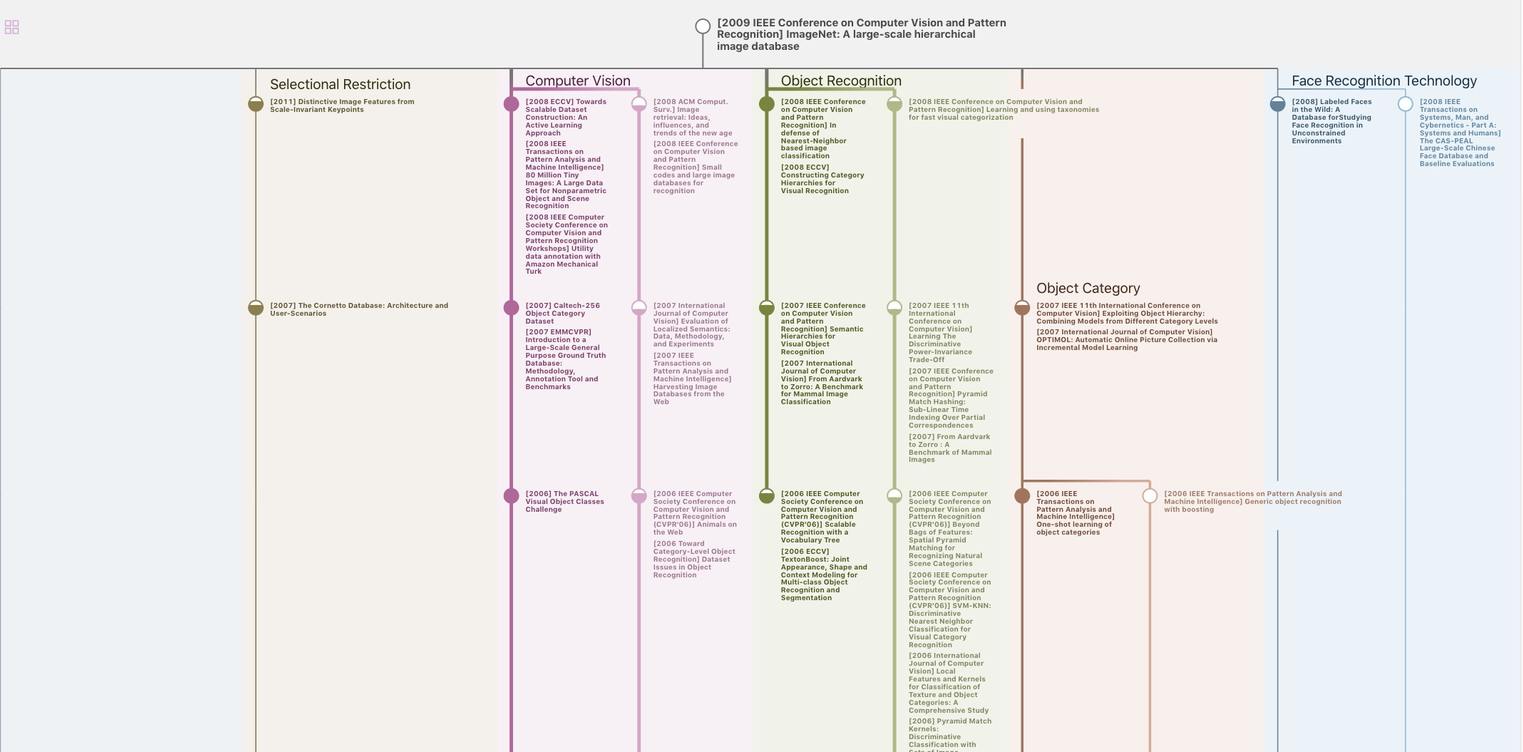
生成溯源树,研究论文发展脉络
Chat Paper
正在生成论文摘要