Minimising redundancy, maximising relevance: HRV feature selection for stress classification
EXPERT SYSTEMS WITH APPLICATIONS(2024)
摘要
Heart rate variability serves as a valuable indicator and biomarker for stress detection and monitoring. Feature selection, which aims to identify relevant features from a large set of variables, is a crucial preprocessing step towards this. However, this task becomes challenging due to high dimensionality and the presence of irrelevant and redundant attributes. The Minimum Redundancy and Maximum Relevance (mRMR) feature selection method addresses this challenge by selecting relevant features while controlling redundancy. This paper presents extensions and evaluated versions of the mRMR feature selection methods for stress detection using Heart Rate Variability (HRV) measures. The proposed feature selection methods extend the traditional mRMR by replacing the Pearson correlation redundancy with non-linear feature redundancy measures capable of capturing more complex relationships between variables. An extensive empirical evaluation is conducted on the proposed mRMR extensions, comparing them with four other baseline feature selection methods using three publicly available datasets. The experimental results demonstrate the effectiveness of incorporating the non-linear feature redundancy measure into the feature selection process.
更多查看译文
关键词
Feature selection,Machine learning,Stress detection,Heart rate variability,Minimum redundancy and maximum relevance
AI 理解论文
溯源树
样例
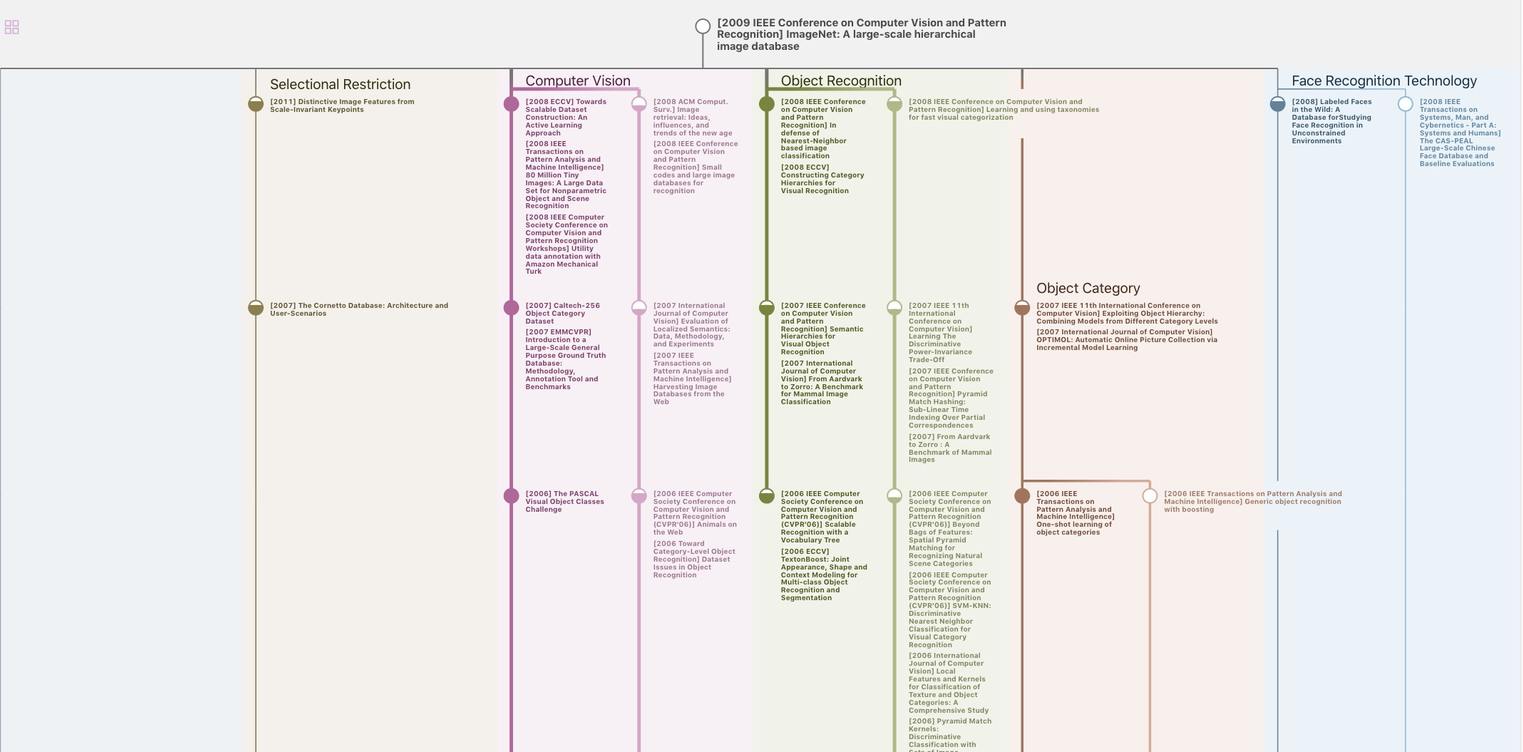
生成溯源树,研究论文发展脉络
Chat Paper
正在生成论文摘要