Invited Paper: In-Sensor Radio Frequency Computing for Energy-Efficient Intelligent Radar
2023 IEEE/ACM INTERNATIONAL CONFERENCE ON COMPUTER AIDED DESIGN, ICCAD(2023)
摘要
Radio Frequency Neural Networks (RFNNs) have demonstrated advantages in realizing intelligent applications across various domains. However, as the model size of deep neural networks rapidly increases, implementing large-scale RFNN in practice requires an extensive number of RF interferometers and consumes a substantial amount of energy. To address this challenge, we propose to utilize low-rank decomposition to transform a large-scale RFNN into a compact RFNN while almost preserving its accuracy. Specifically, we develop a Tensor-Train RFNN (TT-RFNN) where each layer comprises a sequence of low-rank third-order tensors, leading to a notable reduction in parameter count, thereby optimizing RF interferometer utilization in comparison to the original large-scale RFNN. Additionally, considering the inherent physical errors when mapping TT-RFNN to RF device parameters in real-world deployment, from a general perspective, we construct the Robust TT-RFNN (RTT-RFNN) by incorporating a robustness solver on TT-RFNN to enhance its robustness. To adapt the RTT-RFNN to varying requirements of reshaping operations, we further provide a reconfigurable reshaping solution employing RF switch matrices. Empirical evaluations conducted on MNIST and CIFAR-10 datasets show the effectiveness of our proposed method.
更多查看译文
关键词
Radio frequency neural network,tensor-train decomposition,model compression,efficiency,robustness
AI 理解论文
溯源树
样例
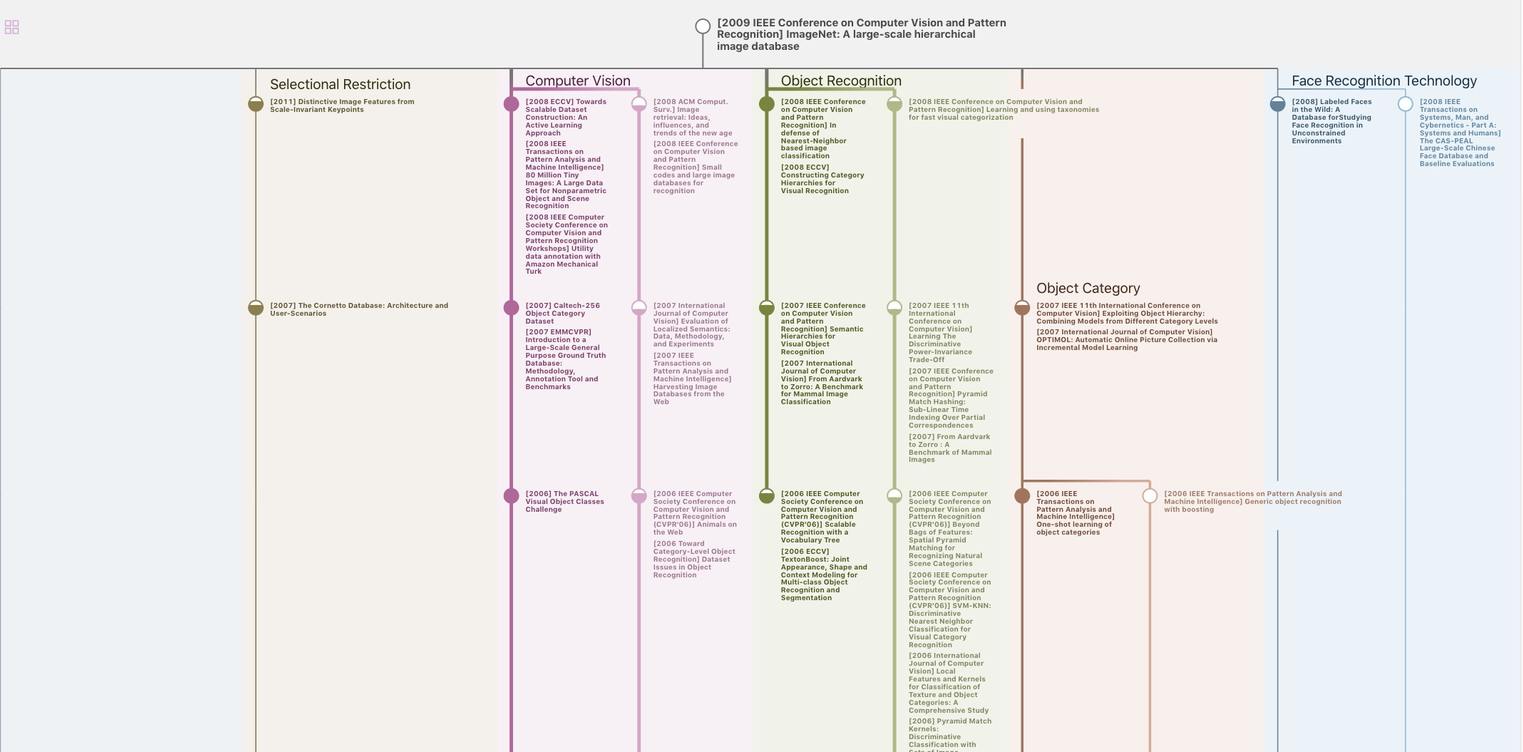
生成溯源树,研究论文发展脉络
Chat Paper
正在生成论文摘要