Automatic Multi-Class Collective Motion Recognition Using a Decision Forest Extracted from Neural Networks
2023 IEEE Region 10 Symposium (TENSYMP)(2023)
Key words
Shallow Neural Network,Deep Neural Network,Knowledge Extraction,Automatic Collective Behaviour Recognition,Multi-Class Classification
AI Read Science
Must-Reading Tree
Example
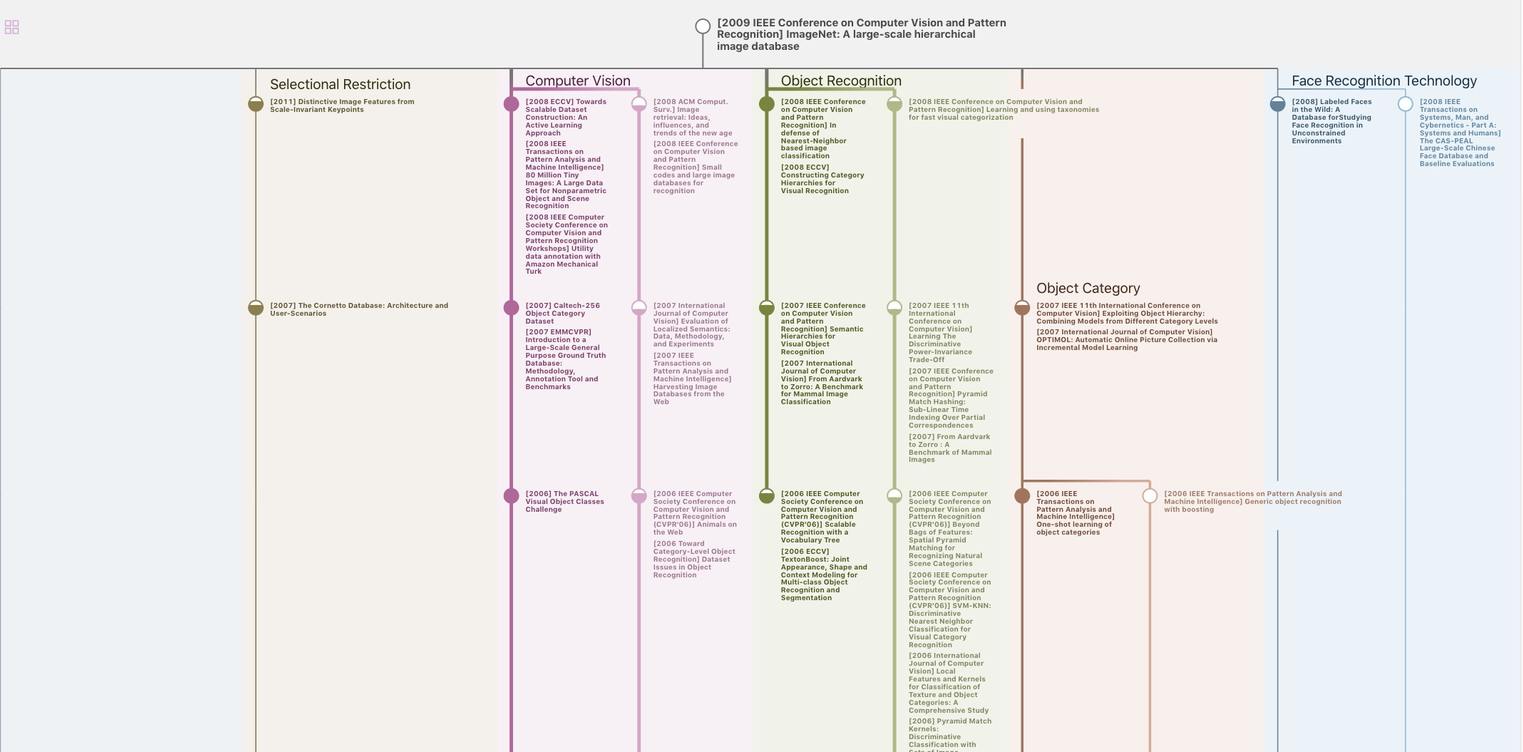
Generate MRT to find the research sequence of this paper
Chat Paper
Summary is being generated by the instructions you defined