RA-GAN Data Enhancement Method to Improve the Performance of Lung Parenchyma Segmentation
2023 IEEE 3rd International Conference on Electronic Technology, Communication and Information (ICETCI)(2023)
关键词
lung parenchyma segmentation,conditional generative adversarial network,small samples,visual transformer,data enhancement
AI 理解论文
溯源树
样例
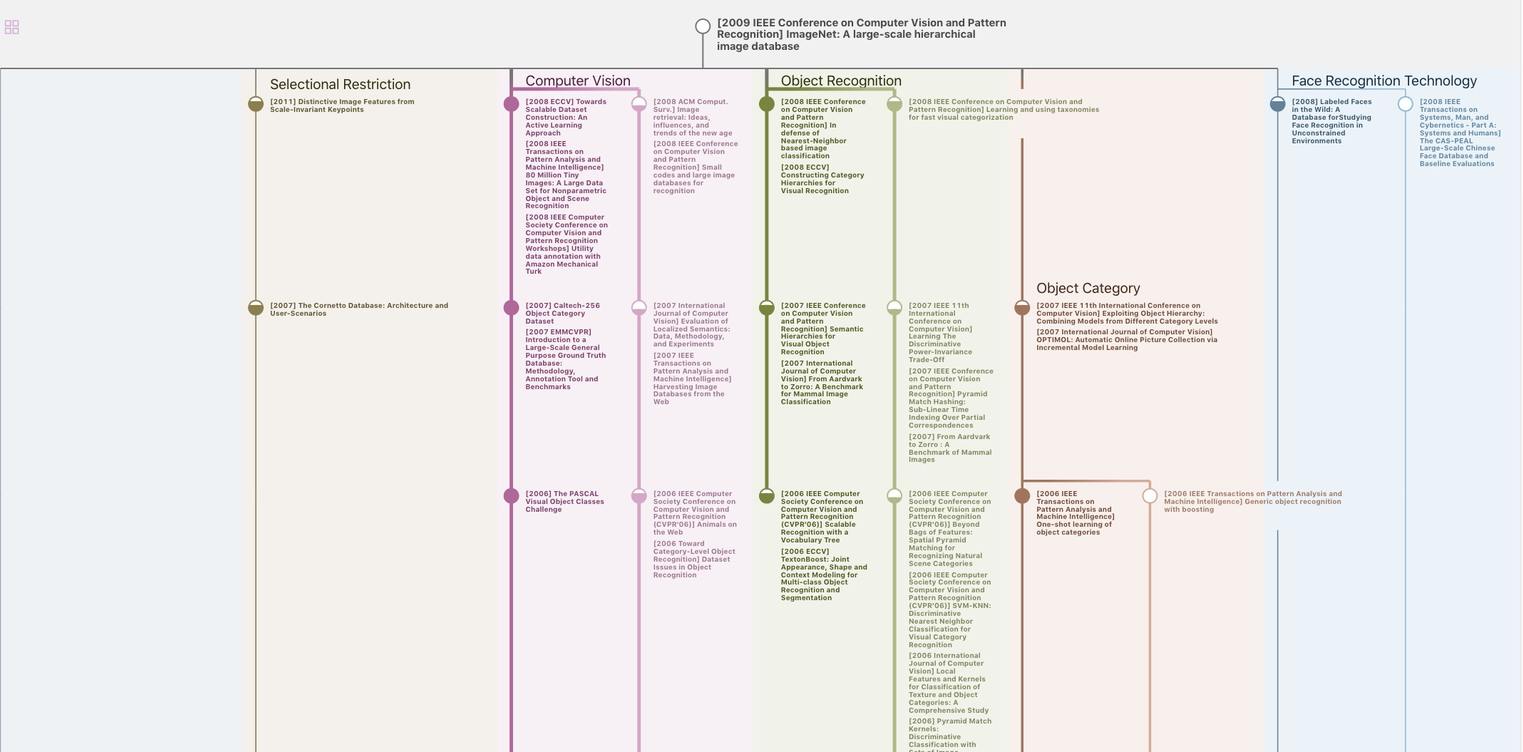
生成溯源树,研究论文发展脉络
Chat Paper
正在生成论文摘要