Augmentation Robust Self-Supervised Learning for Human Activity Recognition
ICASSP 2023 - 2023 IEEE International Conference on Acoustics, Speech and Signal Processing (ICASSP)(2023)
摘要
Human Activity Recognition (HAR) is widely applied on wearable devices in our daily lives. However, acquiring high-quality wearable sensor data set with ground-truths is challenging due to the high cost in collecting data and necessity of domain experts. In order to achieve generalization from limited data, we study augmentation-based Self-Supervised Learning (SSL) for data from wearable devices. However, there is an issue in one of the most popular SSL approaches, contrastive learning: it is sensitive to the choice of data augmentations. To resolve this, we first propose to combine contrastive learning with generative learning, which is robust to augmentations. Second, we propose an automatic augmentation policy search method to discover the most promising augmentation policy. We empirically verify our approaches on three public HAR datasets. Experimental results show that our proposed SSL approach is robust to augmentations, and delivers higher accuracy than contrastive learning. Additionally, with the searched augmentation policy we are able to further improve the accuracy of HAR task.
更多查看译文
关键词
Human Activity Recognition,Self-supervised learning
AI 理解论文
溯源树
样例
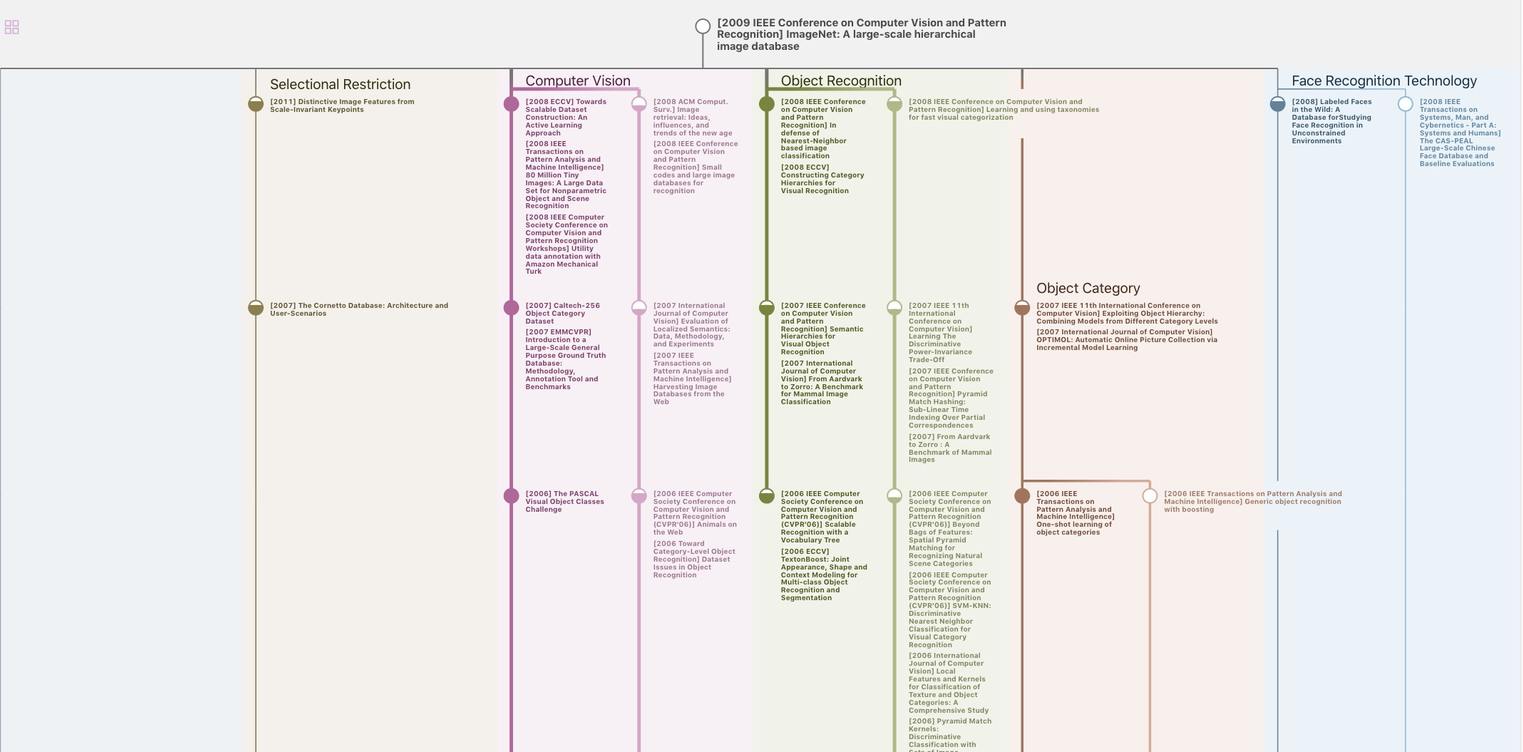
生成溯源树,研究论文发展脉络
Chat Paper
正在生成论文摘要