Cross-Representation Loss-Guided Complex Convolutional Network for Speech Enhancement of VHF Audio
IEEE TRANSACTIONS ON INSTRUMENTATION AND MEASUREMENT(2023)
关键词
Speech enhancement,Convolution,Convolutional neural networks,Decoding,Spectrogram,Signal to noise ratio,Learning systems,Complex convolution network,cross-representation loss,nonrecursive temporal modeling,speech enhancement,very high-frequency (VHF) audio
AI 理解论文
溯源树
样例
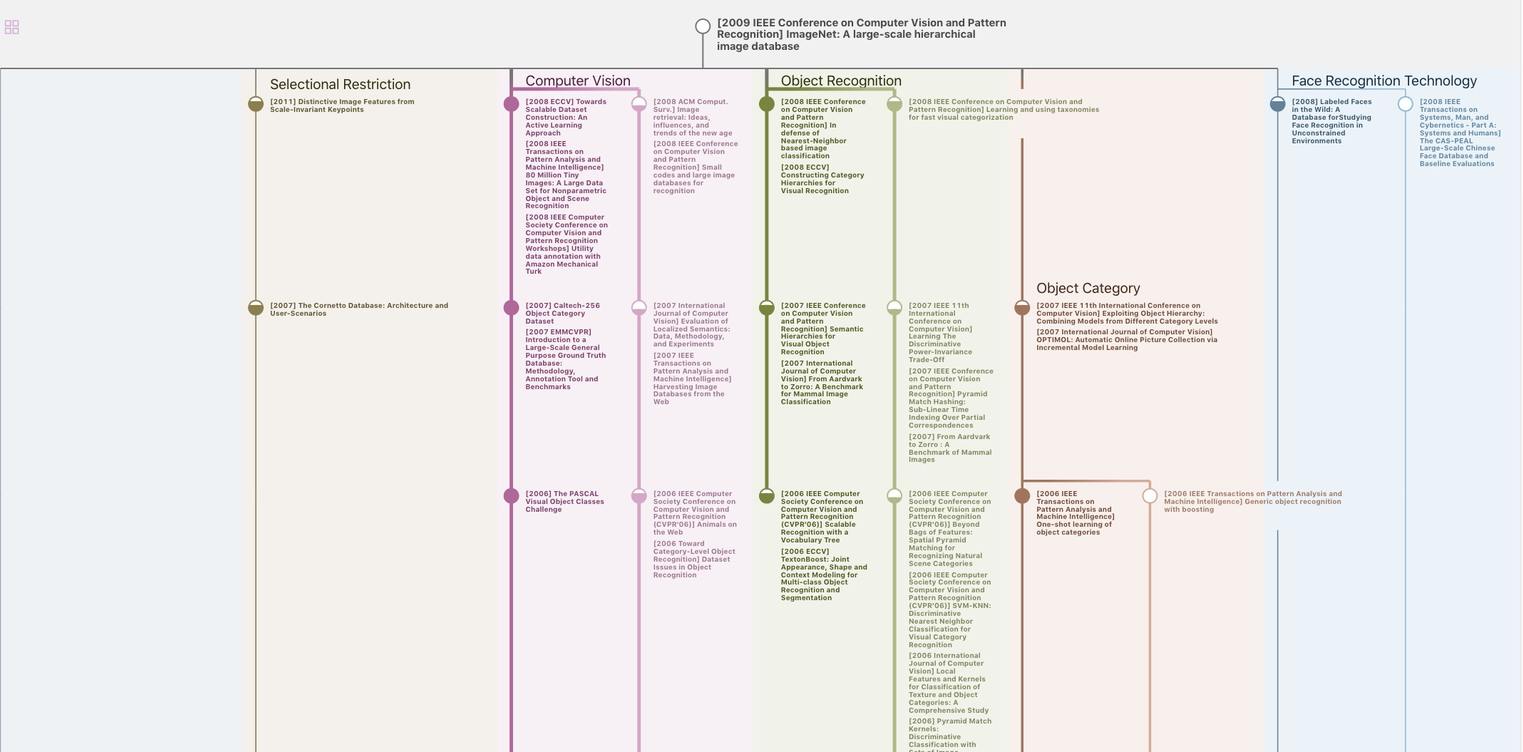
生成溯源树,研究论文发展脉络
Chat Paper
正在生成论文摘要