Towards Publicly Accountable Frontier LLMs: Building an External Scrutiny Ecosystem under the ASPIRE Framework.
CoRR(2023)
摘要
With the increasing integration of frontier large language models (LLMs) into society and the economy, decisions related to their training, deployment, and use have far-reaching implications. These decisions should not be left solely in the hands of frontier LLM developers. LLM users, civil society and policymakers need trustworthy sources of information to steer such decisions for the better. Involving outside actors in the evaluation of these systems - what we term 'external scrutiny' - via red-teaming, auditing, and external researcher access, offers a solution. Though there are encouraging signs of increasing external scrutiny of frontier LLMs, its success is not assured. In this paper, we survey six requirements for effective external scrutiny of frontier AI systems and organize them under the ASPIRE framework: Access, Searching attitude, Proportionality to the risks, Independence, Resources, and Expertise. We then illustrate how external scrutiny might function throughout the AI lifecycle and offer recommendations to policymakers.
更多查看译文
AI 理解论文
溯源树
样例
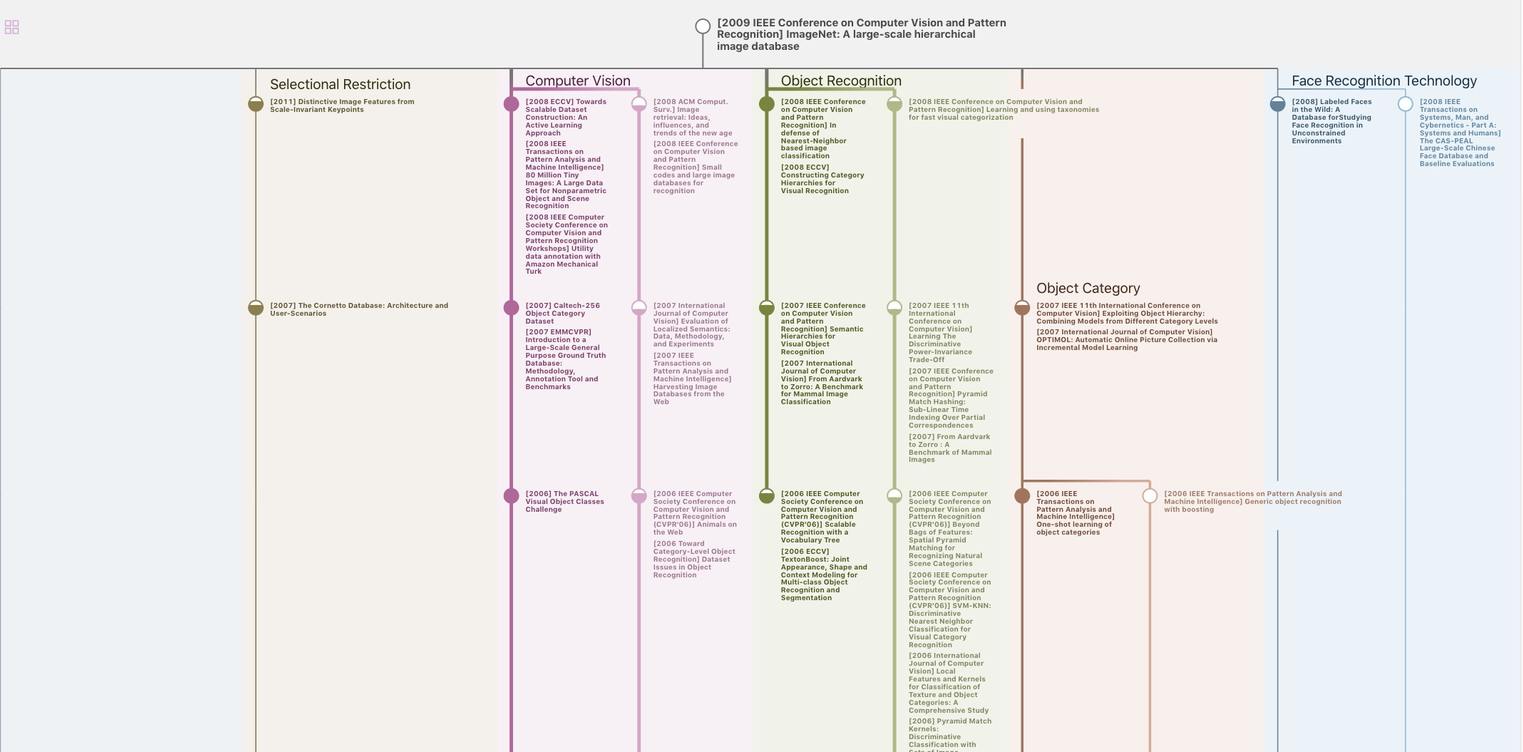
生成溯源树,研究论文发展脉络
Chat Paper
正在生成论文摘要