Robust Multi-Model Subset Selection
arxiv(2023)
摘要
Modern datasets in biology and chemistry are often characterized by the
presence of a large number of variables and outlying samples due to measurement
errors or rare biological and chemical profiles. To handle the characteristics
of such datasets we introduce a method to learn a robust ensemble comprised of
a small number of sparse, diverse and robust models, the first of its kind in
the literature. The degree to which the models are sparse, diverse and
resistant to data contamination is driven directly by the data based on a
cross-validation criterion. We establish the finite-sample breakdown of the
ensembles and the models that comprise them, and we develop a tailored
computing algorithm to learn the ensembles by leveraging recent developments in
l0 optimization. Our extensive numerical experiments on synthetic and
artificially contaminated real datasets from genomics and cheminformatics
demonstrate the competitive advantage of our method over state-of-the-art
sparse and robust methods. We also demonstrate the applicability of our
proposal on a cardiac allograft vasculopathy dataset.
更多查看译文
AI 理解论文
溯源树
样例
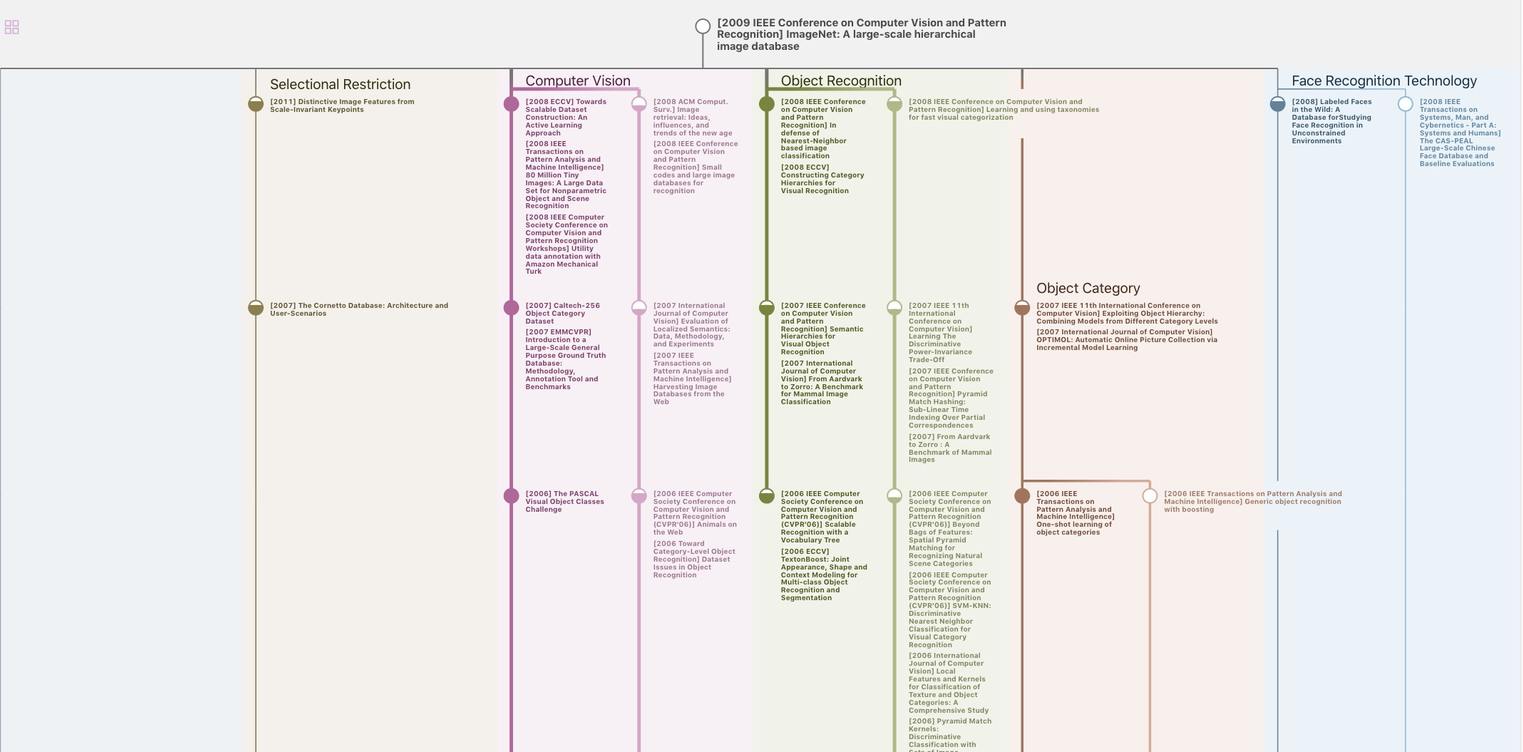
生成溯源树,研究论文发展脉络
Chat Paper
正在生成论文摘要