Capturing Cell Heterogeneity in Representations of Cell Populations for Image-Based Profiling Using Contrastive Learning
PLOS COMPUTATIONAL BIOLOGY(2024)
关键词
Cell Heterogeneity,Cellular Imaging,Phenotypic Profiling,Spatial Profiling,Bioimage Analysis
AI 理解论文
溯源树
样例
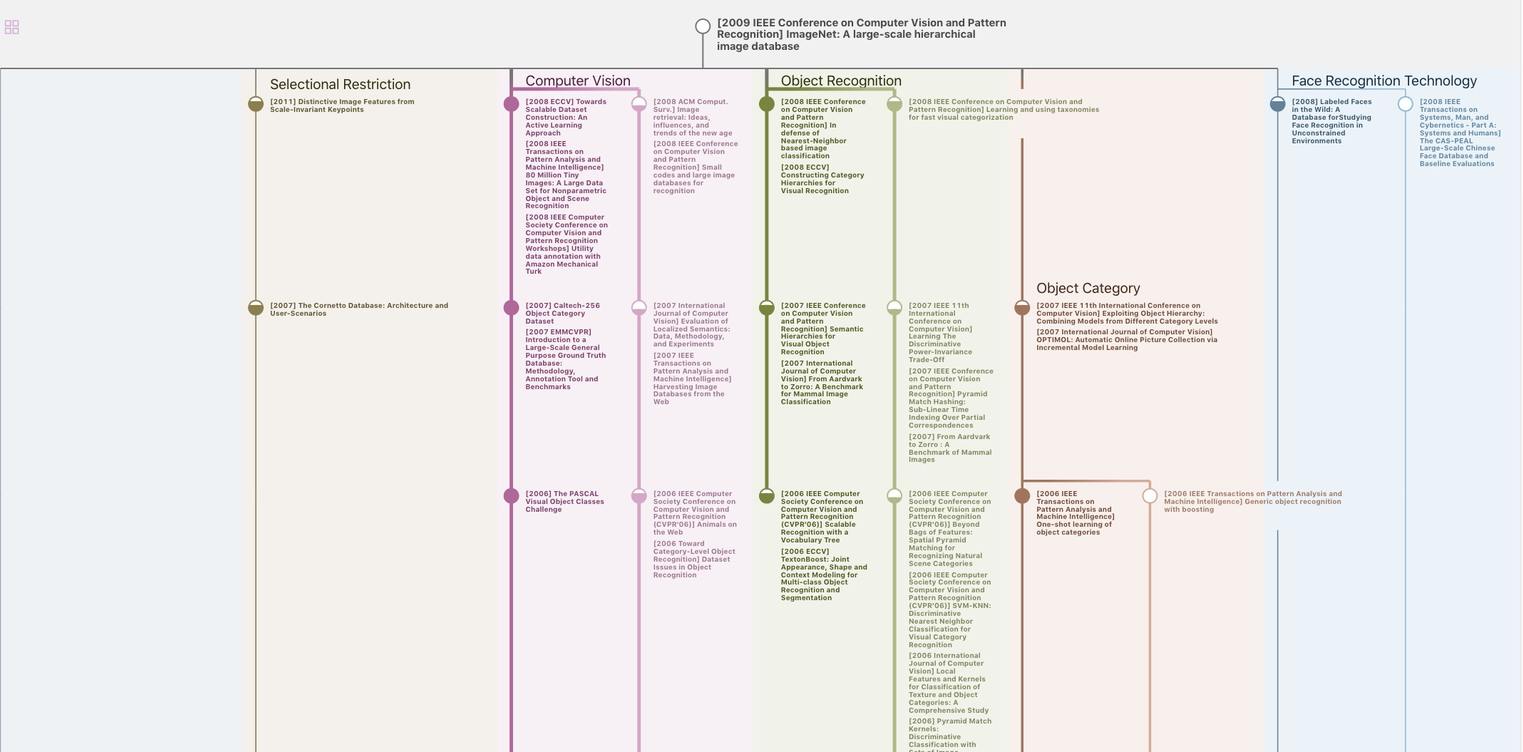
生成溯源树,研究论文发展脉络
Chat Paper
正在生成论文摘要