Hypothesis Generation For Rare and Undiagnosed Diseases Through Clustering and Classifying Time-Versioned Biological Ontologies
bioRxiv (Cold Spring Harbor Laboratory)(2023)
摘要
Rare diseases affect 1-in-10 people in the United States and despite increased genetic testing, up to half never receive a diagnosis. Even when using advanced genome sequencing platforms to discover variants, if there is no connection between the variants found in the patient's genome and their phenotypes in the literature, then the patient will remain undiagnosed. When a direct variant-phenotype connection is not known, putting a patient's information in the larger context of phenotype relationships and protein-protein-interactions may provide an opportunity to find an indirect explanation. Databases such as STRING contain millions of protein-protein-interactions and HPO contains the relations of thousands of phenotypes. By integrating these networks and clustering the entities within we can potentially discover latent gene-to-phenotype connections. The historical records for STRING and HPO provide a unique opportunity to create a network time series for evaluating the cluster significance. Most excitingly, working with Children's Hospital Colorado we provide promising hypotheses about latent gene-to-phenotype connections for 38 patients with undiagnosed diseases. We also provide potential answers for 14 patients listed on MyGene2. Clusters our tool finds significant harbor 2.35 to 8.72 times as many gene-to-phenotypes edges inferred from known drug interactions than clusters finds to be insignificant. Our tool, BOCC, is available as a web app and command line tool here: https://github.com/MSBradshaw/BOCC.
### Competing Interest Statement
The authors have declared no competing interest.
更多查看译文
关键词
undiagnosed diseases,clustering,time-versioned
AI 理解论文
溯源树
样例
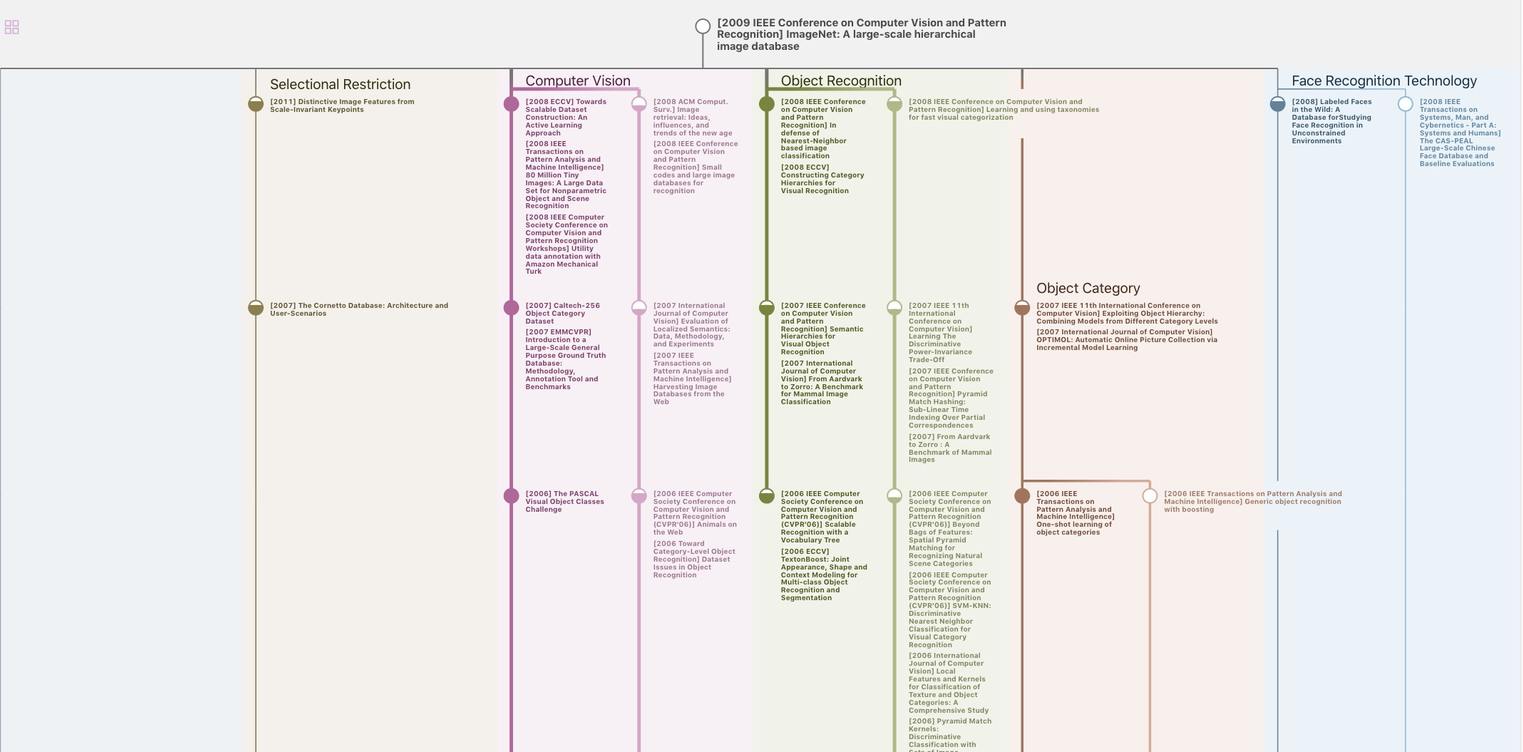
生成溯源树,研究论文发展脉络
Chat Paper
正在生成论文摘要