Knowledge-driven learning, optimization, and experimental design under uncertainty for materials discovery
Patterns(2023)
摘要
Summary: Significant acceleration of the future discovery of novel functional materials requires a fundamental shift from the current materials discovery practice, which is heavily dependent on trial-and-error campaigns and high-throughput screening, to one that builds on knowledge-driven advanced informatics techniques enabled by the latest advances in signal processing and machine learning. In this review, we discuss the major research issues that need to be addressed to expedite this transformation along with the salient challenges involved. We especially focus on Bayesian signal processing and machine learning schemes that are uncertainty aware and physics informed for knowledge-driven learning, robust optimization, and efficient objective-driven experimental design. The bigger picture: Thanks to the rapid advances in artificial intelligence, AI for science (AI4Science) has emerged as one of the new promising research directions for modern science and engineering. In this review, we focus on recent efforts to develop knowledge-driven Bayesian learning and experimental design methods for accelerating the discovery of novel functional materials as well as enhancing the understanding of composition-process-structure-property relationships. We specifically discuss the challenges and opportunities in integrating prior scientific knowledge and physics principles with AI and machine learning (ML) models for accelerating materials and knowledge discovery. The current state-of-the-art methods in knowledge-based prior construction, model fusion, uncertainty quantification, optimal experimental design, and symbolic regression are detailed in the review, along with several detailed case studies and results in materials discovery.
更多查看译文
关键词
optimization,discovery,learning,materials,knowledge-driven
AI 理解论文
溯源树
样例
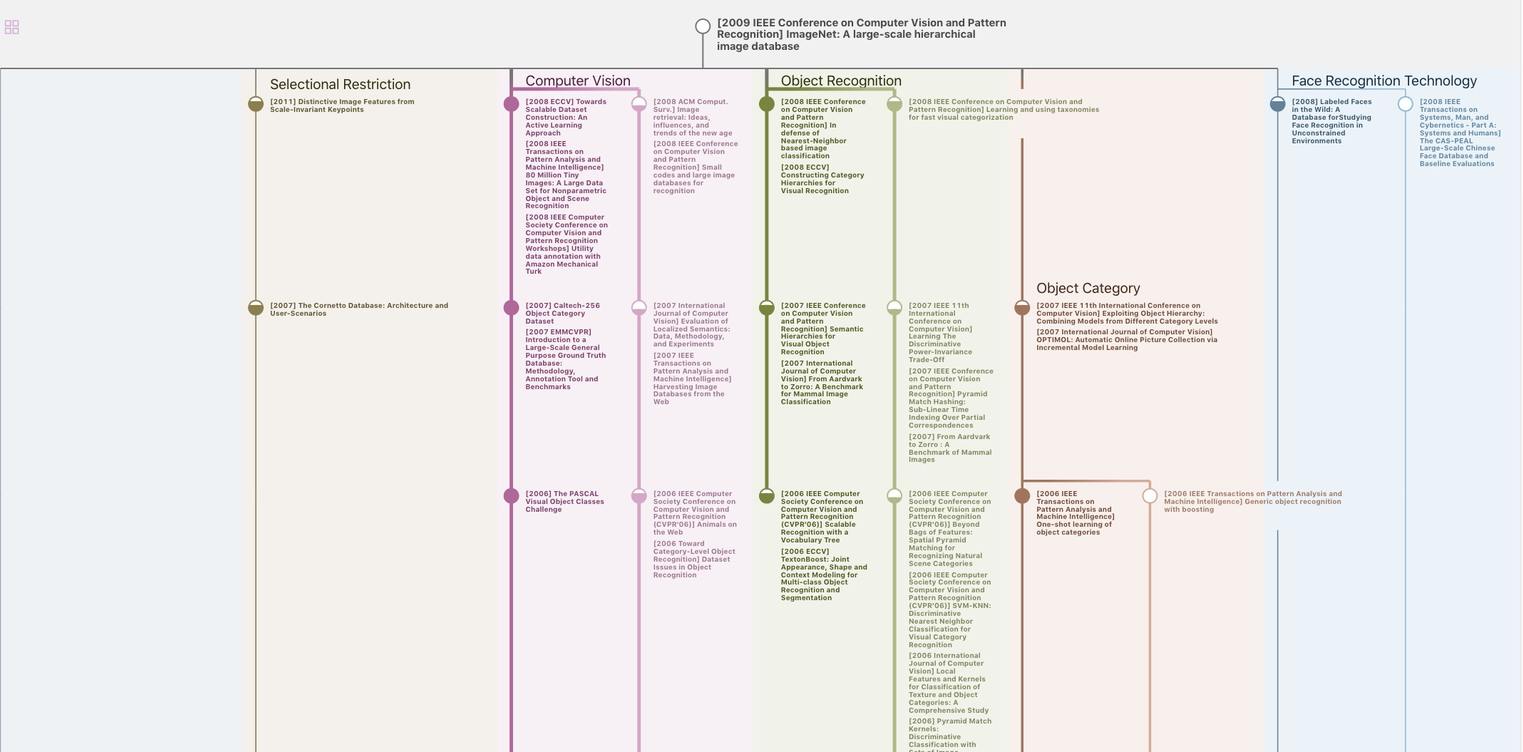
生成溯源树,研究论文发展脉络
Chat Paper
正在生成论文摘要