Radiomics Signature Based on Support Vector Machines for the Prediction of Pathological Complete Response to Neoadjuvant Chemoradiotherapy in Locally Advanced Rectal Cancer
Cancers(2023)
关键词
radiomics signature,nomogram,pathological complete response (pCR),neoadjuvant chemoradiotherapy (nCRT),locally advanced rectal cancer (LARC)
AI 理解论文
溯源树
样例
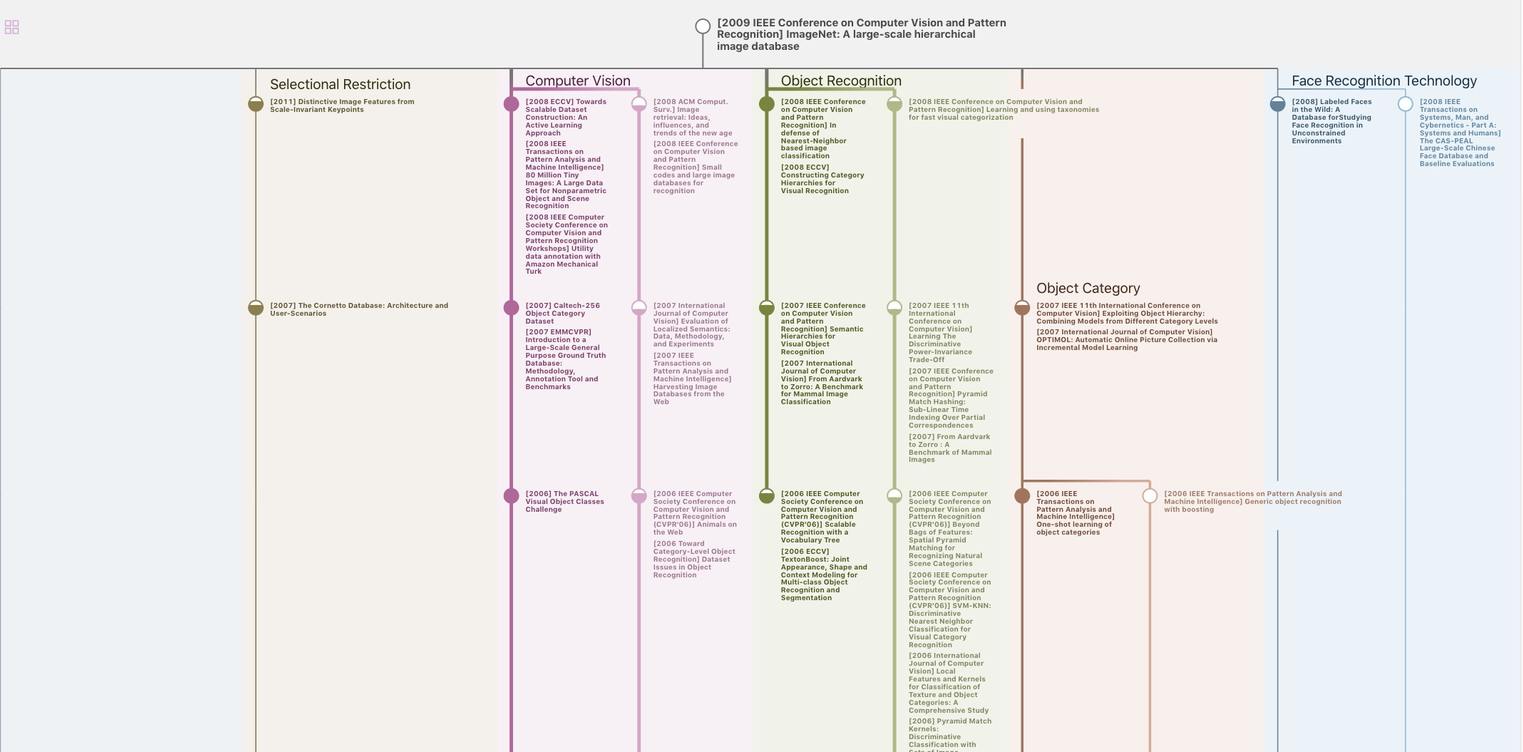
生成溯源树,研究论文发展脉络
Chat Paper
正在生成论文摘要