Intelligent prediction of rolling bearing remaining useful life based on probabilistic DeepAR-Transformer model
MEASUREMENT SCIENCE AND TECHNOLOGY(2024)
摘要
Remaining useful life (RUL) prediction for rolling bearings requires highly accurate and stable long-term prediction capabilities in equipment health management, which demands that the prediction model has strong data reasoning and regression performance. However, it is difficult to accurately capture long-term dependencies via traditional convolutional neural network because the information loss and insufficient analysis are unavoidable during the feature extraction process. An end-to-end time series forecasting method called D-former for RUL prediction of rolling bearings is proposed in this paper. The method mainly consists of DeepAR and a multi-layer encoder, so it is able to extract degradation features directly from the original signal. This method has the following salient features: (1) the designed multi-head attention mechanism can highlight important feature information and realize parallel computing, so the method is extremely suitable for processing long-term time series; (2) the important time feature information is rearranged through DeepAR, so the method has the excellent domain adaptability, and it can achieve accurate prediction of rolling bearing RUL under different working conditions. The verification experiment was implemented on the IEEE PHM 2012 dataset and the XJTU-SY bearing dataset. The experimental results show that the proposed D-former method is actually superior to the existing mainstream RUL prediction methods.
更多查看译文
关键词
remaining useful life prediction,rolling bearing,DeepAR,Transformer
AI 理解论文
溯源树
样例
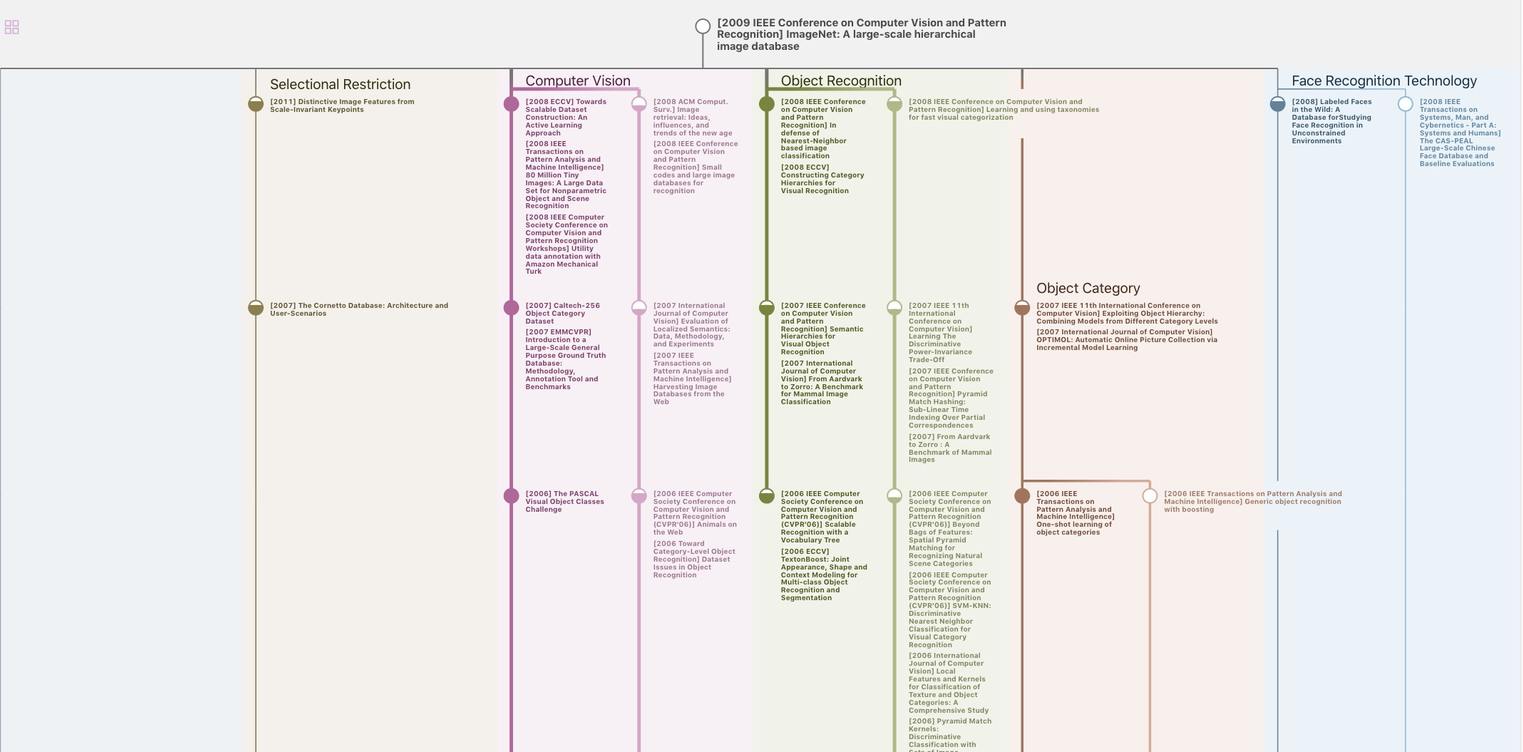
生成溯源树,研究论文发展脉络
Chat Paper
正在生成论文摘要