Predicting Fatigue Crack Growth Metrics from Fractographs: Towards Fractography by Computer Vision
INTERNATIONAL JOURNAL OF FATIGUE(2023)
Key words
Neural networks,Titanium alloys,Fractography,Fatigue crack growth,Machine learning
AI Read Science
Must-Reading Tree
Example
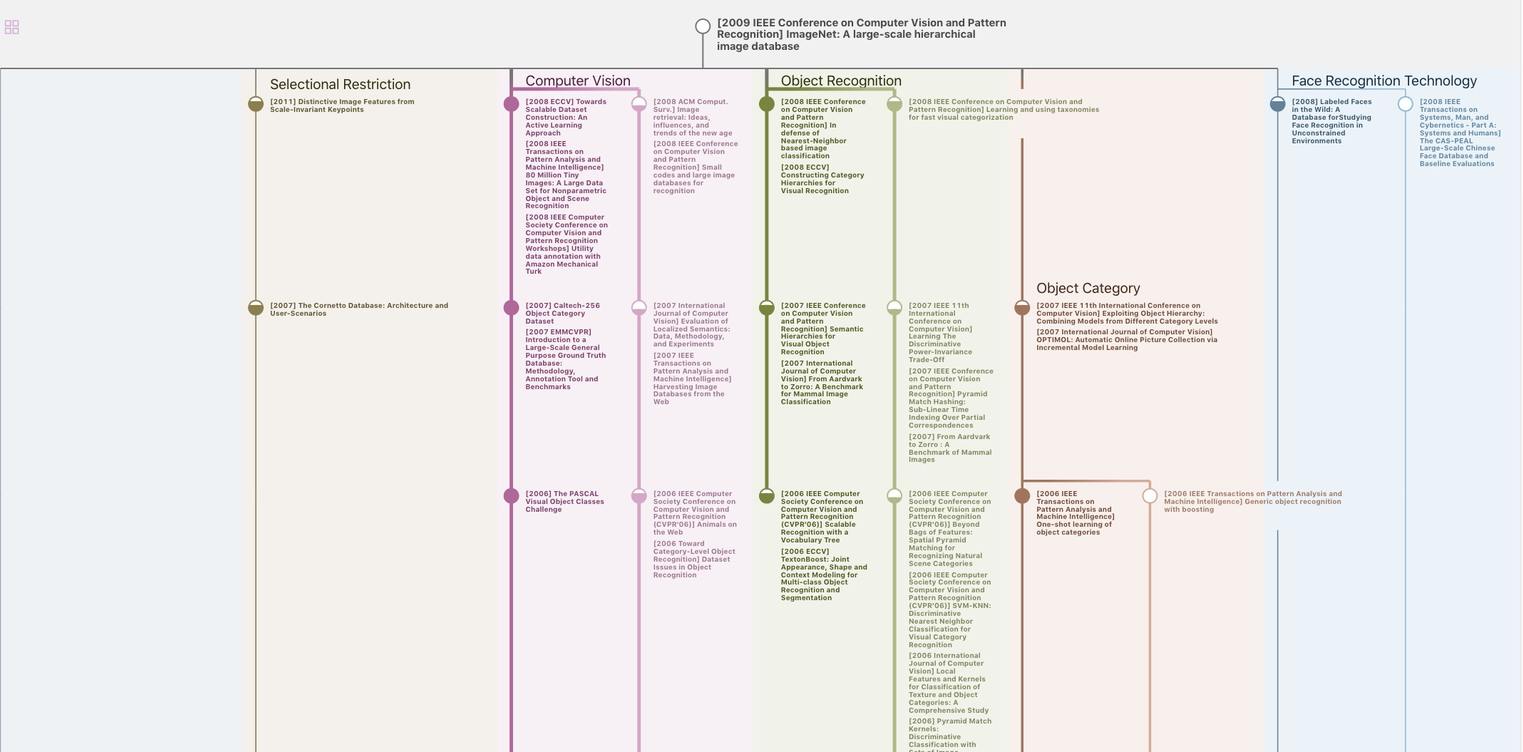
Generate MRT to find the research sequence of this paper
Chat Paper
Summary is being generated by the instructions you defined