Coupling Makes Better: an Intertwined Neural Network for Taxi and Ridesourcing Demand Co-Prediction
IEEE TRANSACTIONS ON INTELLIGENT TRANSPORTATION SYSTEMS(2024)
Key words
Demand prediction,ridesourcing,temporal and spatial dependencies,intertwined neural network,deep models
AI Read Science
Must-Reading Tree
Example
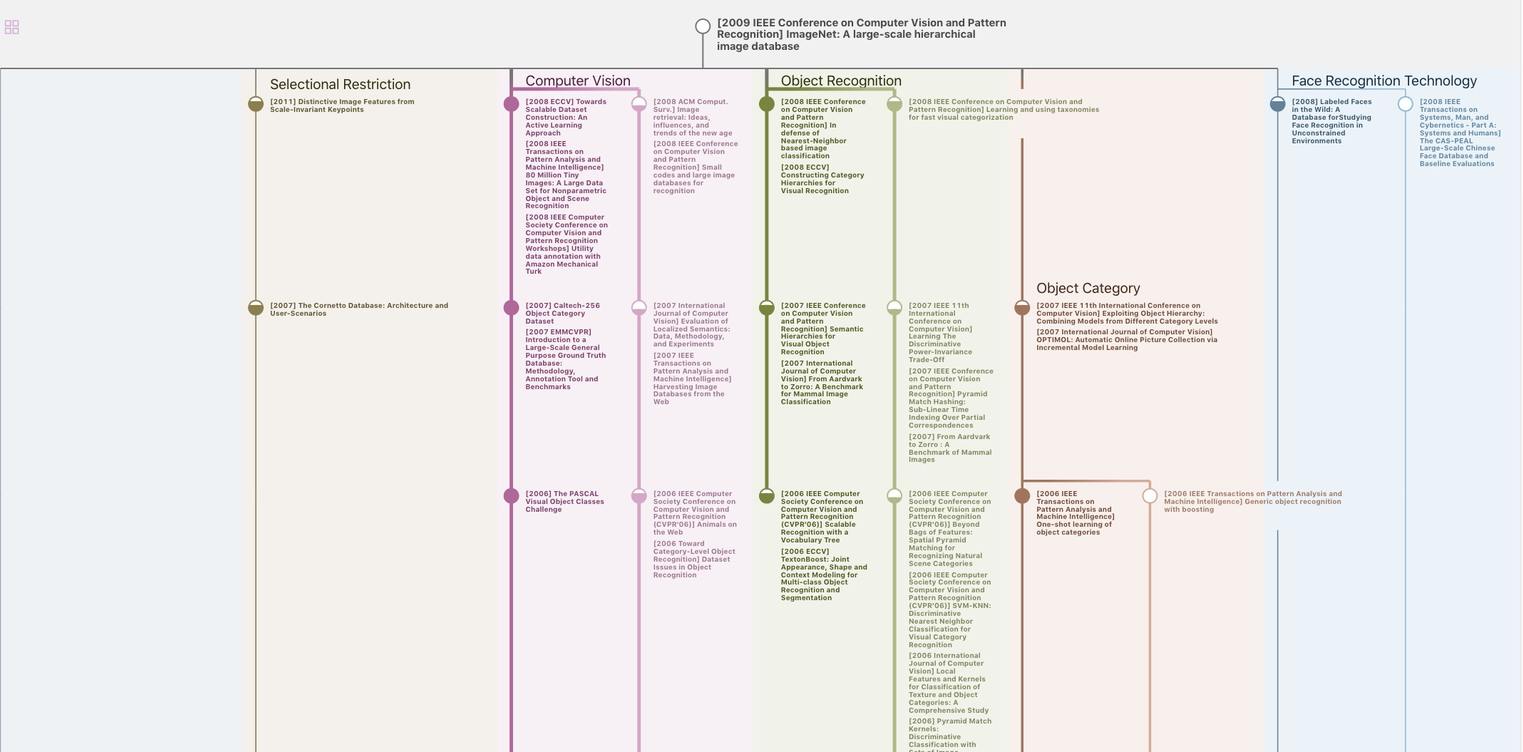
Generate MRT to find the research sequence of this paper
Chat Paper
Summary is being generated by the instructions you defined