Integrative Learning of Disentangled Representations from Single-Cell RNA-sequencing Datasets
biorxiv(2023)
Key words
Droplet-based Sequencing,Cell Heterogeneity,Single-Cell
AI Read Science
Must-Reading Tree
Example
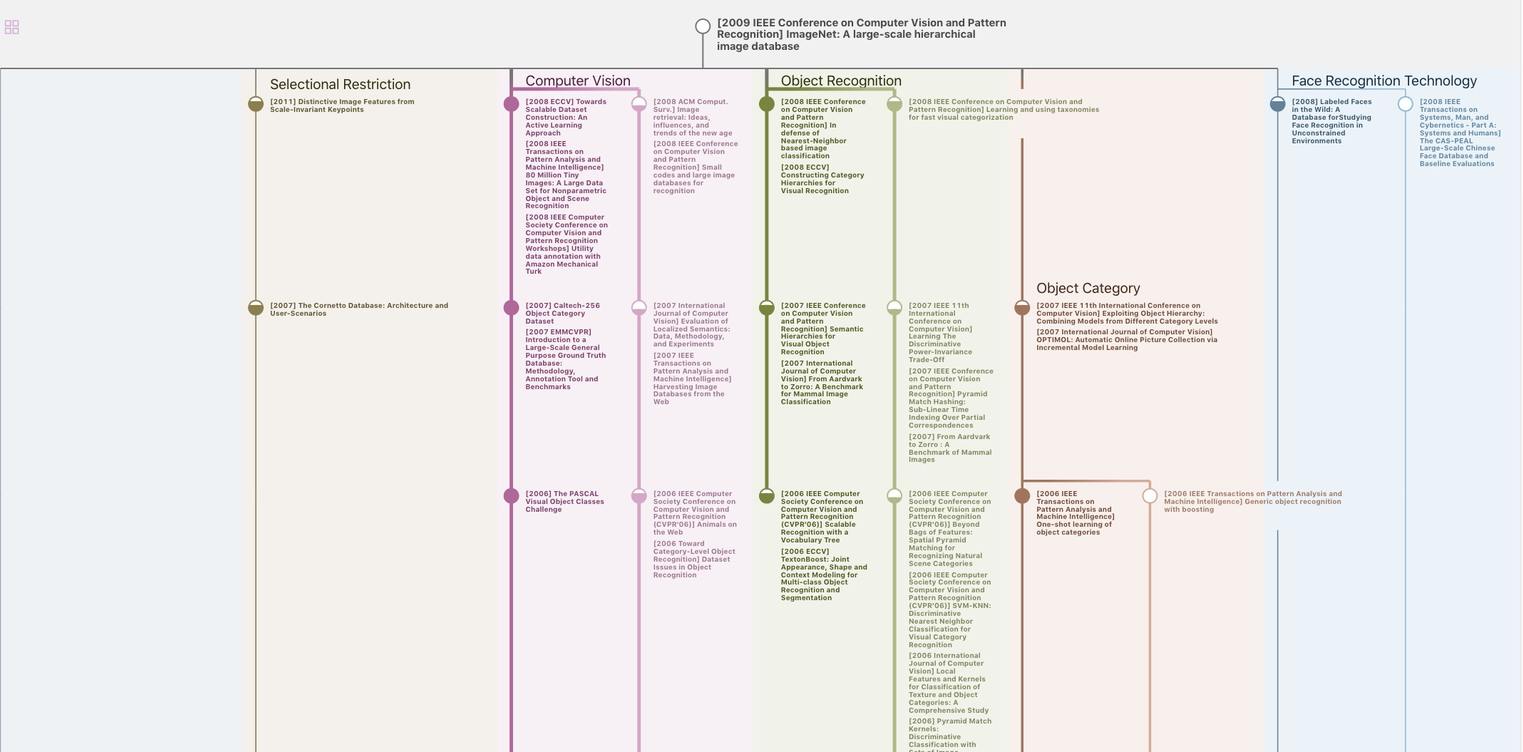
Generate MRT to find the research sequence of this paper
Chat Paper
Summary is being generated by the instructions you defined