MP27-01 TARGETED DEPLETION OF FIDGETIN-LIKE 2 (FL2) PROMOTES NERVE REGENERATION AND IMPROVES BOTH ERECTILE AND BLADDER FUNCTION OUTCOMES IN A RODENT MODEL OF RADICAL PROSTATECTOMY
Journal of Urology(2023)
AI 理解论文
溯源树
样例
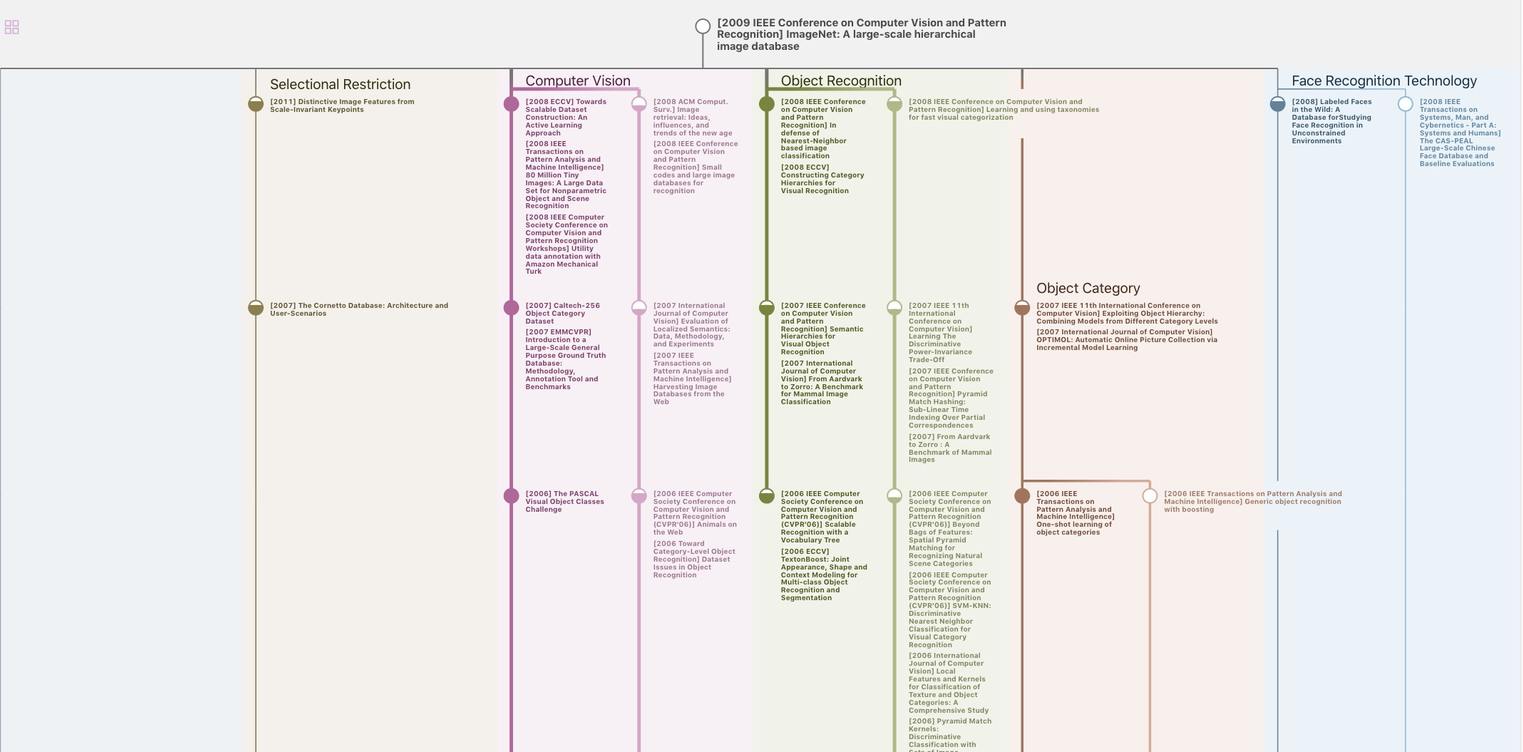
生成溯源树,研究论文发展脉络
Chat Paper
正在生成论文摘要