GLoCNet: Robust Feature Matching with Global–Local Consistency Network for Remote Sensing Image Registration
IEEE TRANSACTIONS ON GEOSCIENCE AND REMOTE SENSING(2023)
关键词
Feature extraction,Image registration,Remote sensing,Deep learning,Distortion,Task analysis,Sensors,Feature matching,global transformation consistency (GTC),local neighborhood consistency (LNC),remote sensing,transformation patterns
AI 理解论文
溯源树
样例
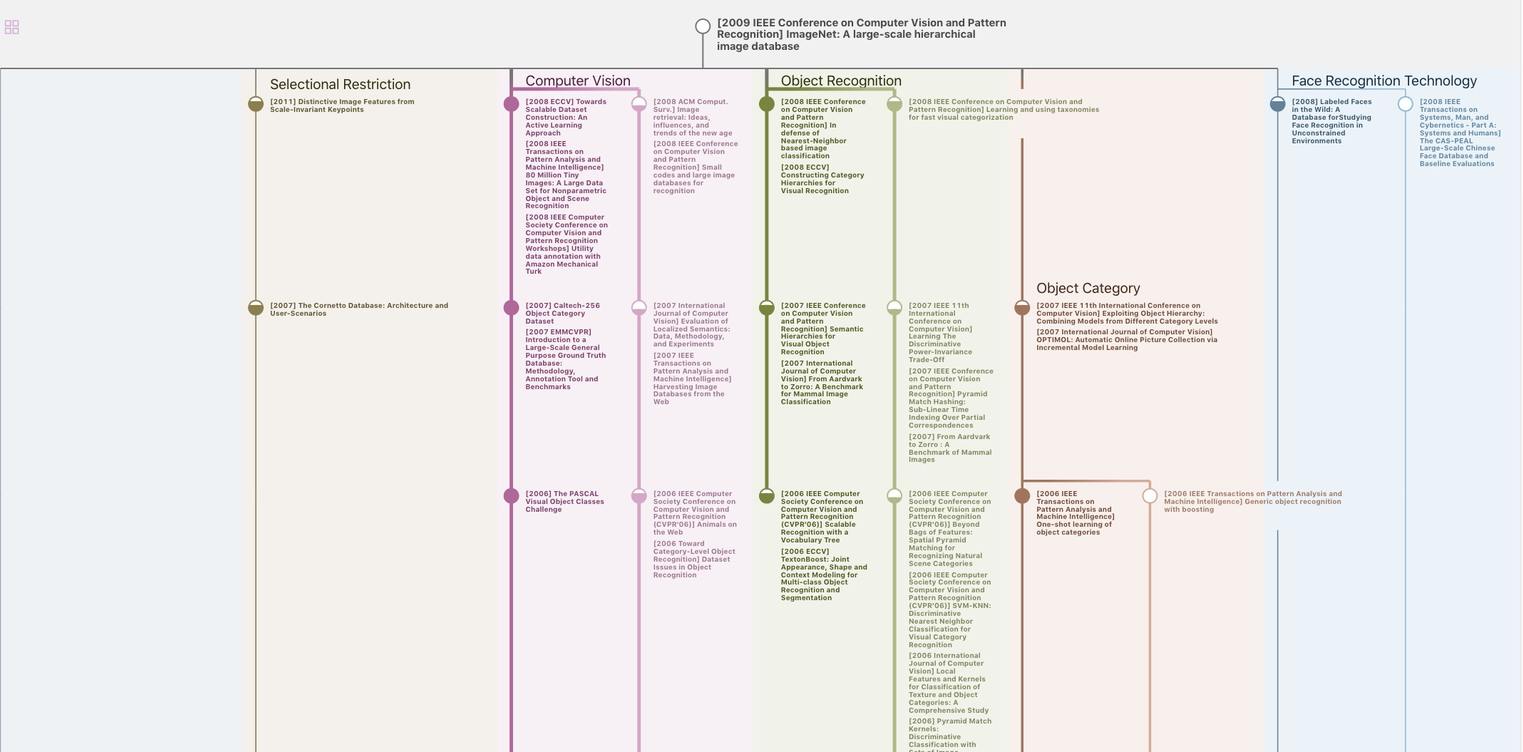
生成溯源树,研究论文发展脉络
Chat Paper
正在生成论文摘要