Learning Occlusion Disentanglement with Fine-grained Localization for Occluded Person Re-identification
PROCEEDINGS OF THE 31ST ACM INTERNATIONAL CONFERENCE ON MULTIMEDIA, MM 2023(2023)
关键词
Image Retrieval,Person Re-Identification,Occlusion Disentanglement,Fine-grained Localization
AI 理解论文
溯源树
样例
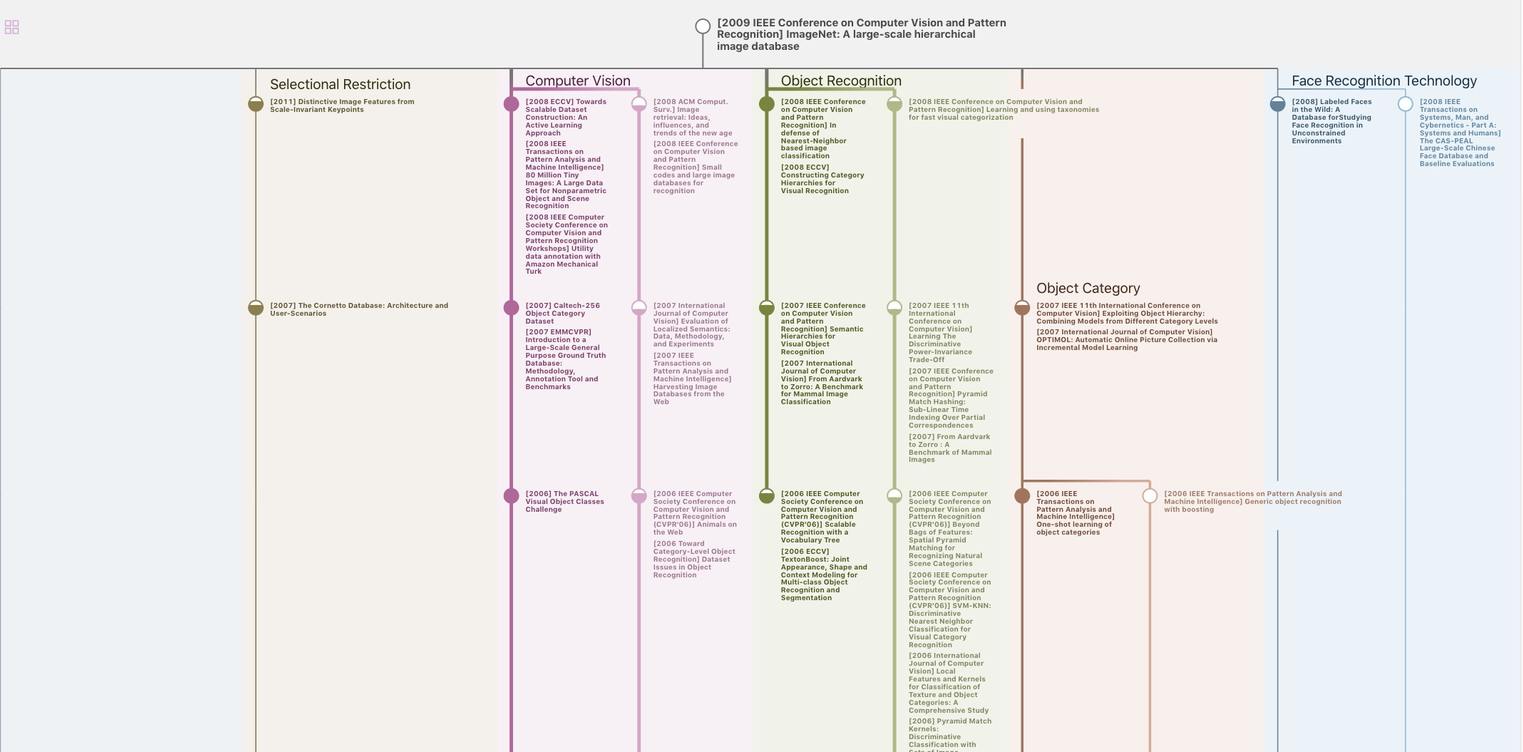
生成溯源树,研究论文发展脉络
Chat Paper
正在生成论文摘要