Rice Yield Prediction Using Sentinel-1 Radar Vegetation Indices and XGBoost
2023 SAR in Big Data Era (BIGSARDATA)(2023)
关键词
Rice yield,SAR,Sentinel-1,Radar vegetation indices,Machine learning
AI 理解论文
溯源树
样例
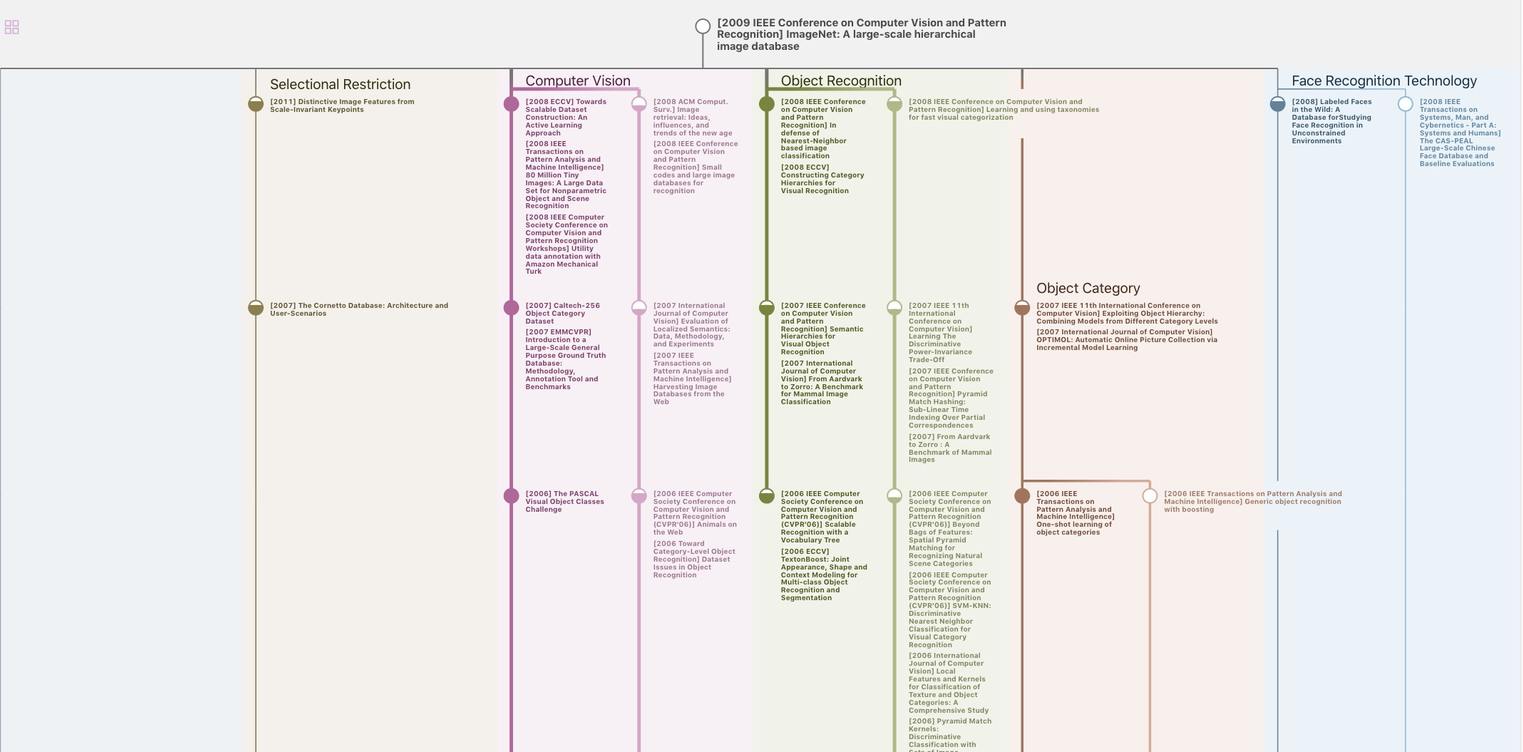
生成溯源树,研究论文发展脉络
Chat Paper
正在生成论文摘要
2023 SAR in Big Data Era (BIGSARDATA)(2023)