Group Distributionally Robust Knowledge Distillation
MACHINE LEARNING IN MEDICAL IMAGING, MLMI 2023, PT II(2024)
摘要
Knowledge distillation enables fast and effective transfer of features learned from a bigger model to a smaller one. However, distillation objectives are susceptible to sub-population shifts, a common scenario in medical imaging analysis which refers to groups/domains of data that are underrepresented in the training set. For instance, training models on health data acquired from multiple scanners or hospitals can yield subpar performance for minority groups. In this paper, inspired by distributionally robust optimization (DRO) techniques, we address this shortcoming by proposing a group-aware distillation loss. During optimization, a set of weights is updated based on the per-group losses at a given iteration. This way, our method can dynamically focus on groups that have low performance during training. We empirically validate our method, GroupDistil on two benchmark datasets (natural images and cardiac MRIs) and show consistent improvement in terms of worst-group accuracy.
更多查看译文
关键词
Invariance,Knowledge Distillation,Sub-population Shift,Classification
AI 理解论文
溯源树
样例
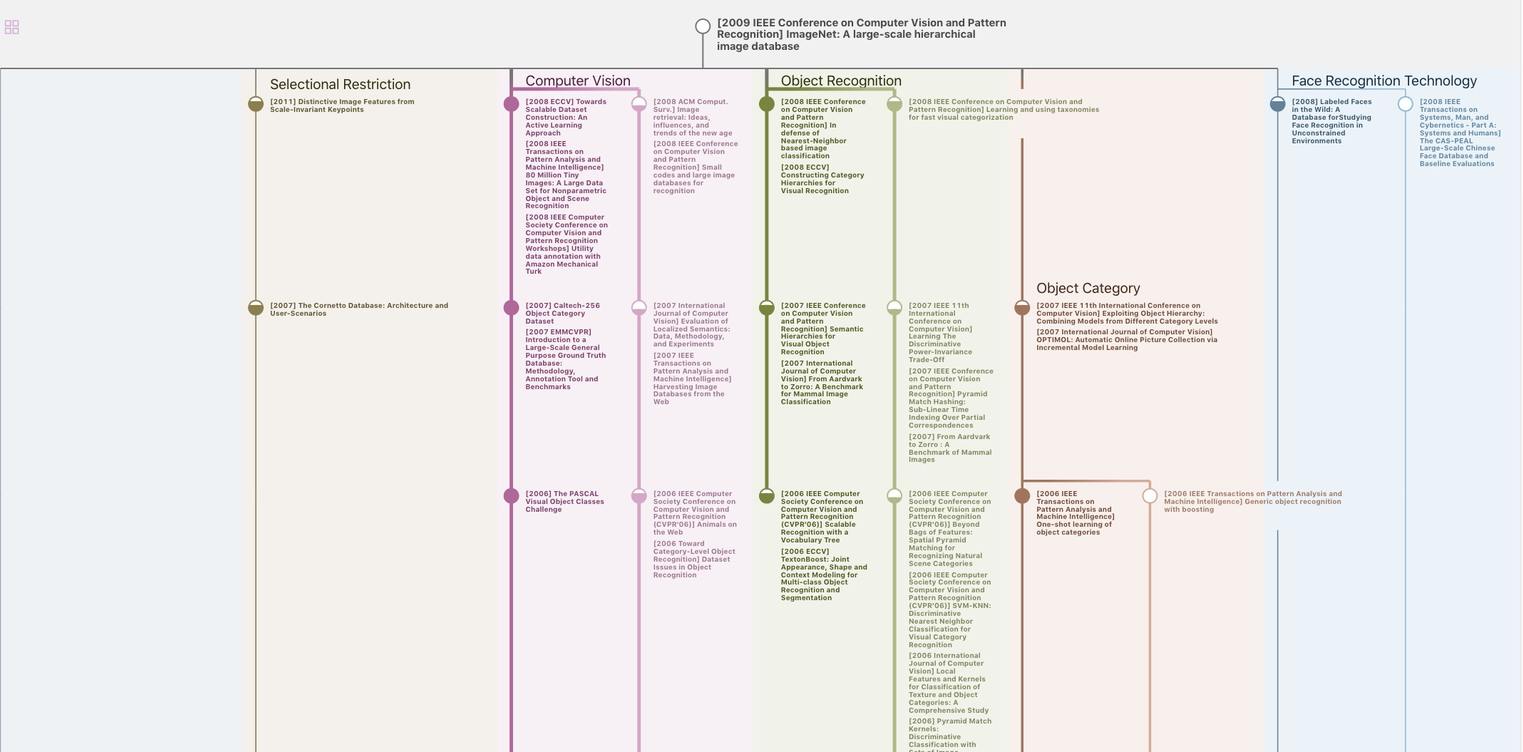
生成溯源树,研究论文发展脉络
Chat Paper
正在生成论文摘要