Evaluation of the Hierarchical Correspondence between the Human Brain and Artificial Neural Networks: A Review
Biology(2023)
摘要
Simple Summary Artificial neural networks, inspired by the human brain, have demonstrated human-level performance across multiple task domains, raising the exciting possibility of them returning insights to neuroscientists about the human brain. However, artificial neural networks cannot be directly compared to the brain due to inherent differences in structure and computation. Here, we review the variety of approaches that researchers have thus far taken to evaluate the correspondence between the two, organized across multiple levels of analysis (node, layer, network, and behavior). In surveying these approaches, we note some of the insights uncovered, their limitations, and future directions in a domain of research that is developing quickly and with few established standards and practices. Our aim is to provide a systemized overview and guidance toward establishing a firmer theoretical and methodological framework in this emerging field.Abstract Artificial neural networks (ANNs) that are heavily inspired by the human brain now achieve human-level performance across multiple task domains. ANNs have thus drawn attention in neuroscience, raising the possibility of providing a framework for understanding the information encoded in the human brain. However, the correspondence between ANNs and the brain cannot be measured directly. They differ in outputs and substrates, neurons vastly outnumber their ANN analogs (i.e., nodes), and the key algorithm responsible for most of modern ANN training (i.e., backpropagation) is likely absent from the brain. Neuroscientists have thus taken a variety of approaches to examine the similarity between the brain and ANNs at multiple levels of their information hierarchy. This review provides an overview of the currently available approaches and their limitations for evaluating brain-ANN correspondence.
更多查看译文
关键词
human brain,hierarchical correspondence,artificial neural networks,neural networks
AI 理解论文
溯源树
样例
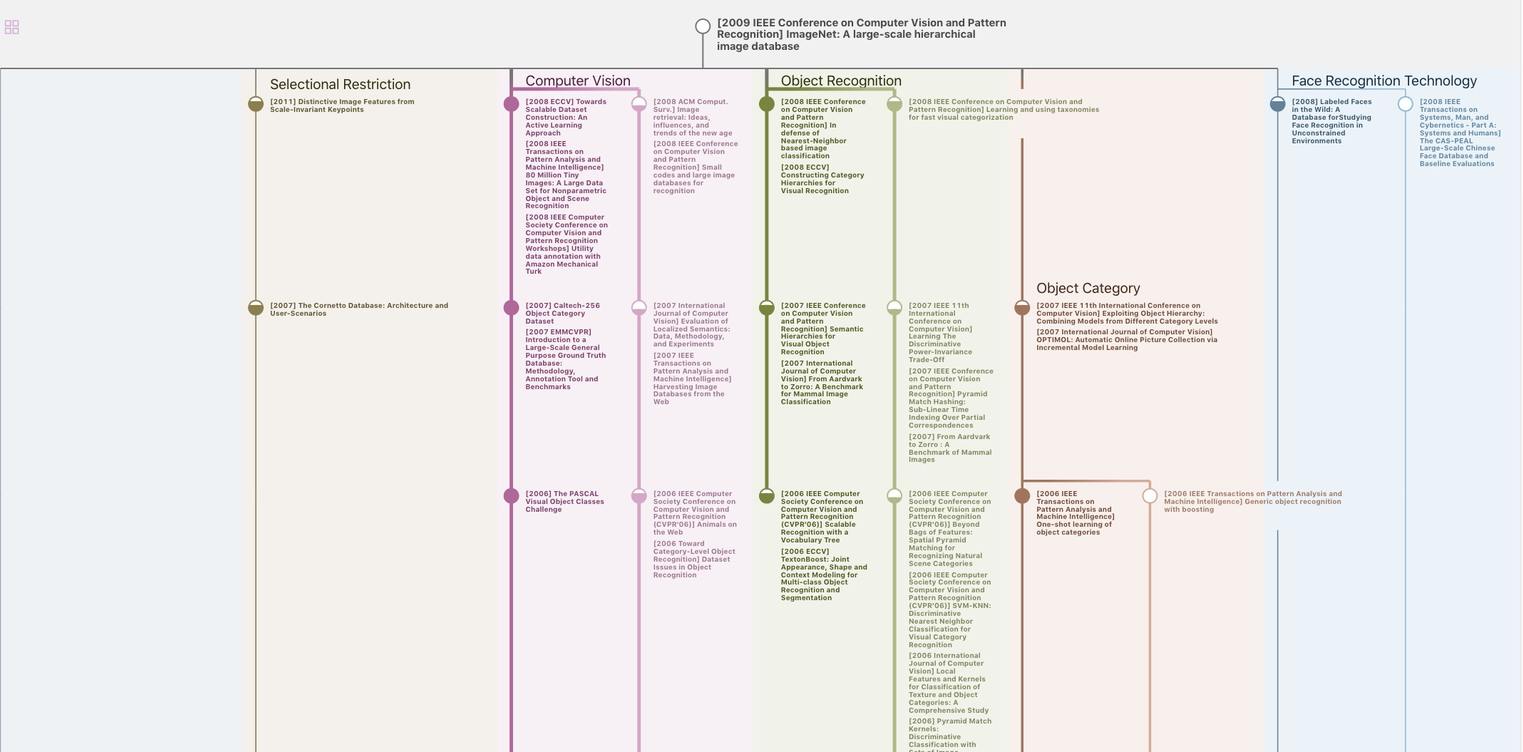
生成溯源树,研究论文发展脉络
Chat Paper
正在生成论文摘要