A Lightweight Network Based on Improved YOLOv5s for Insulator Defect Detection
Electronics(2023)
摘要
Insulators on transmission lines can be damaged to different degrees due to extreme weather conditions, which threaten the safe operation of the power system. In order to detect damaged insulators in time and meet the needs of real-time detection, this paper proposes a multi-defect and lightweight detection algorithm for insulators based on the improved YOLOv5s. To reduce the network parameters, we have integrated the Ghost module and introduced C3Ghost as a replacement for the backbone network. This enhancement enables a more efficient detection model. Moreover, we have added a new detection layer specifically designed for small objects, and embedded an attention mechanism into the network, significantly improving its detection capability for smaller insulators. Furthermore, we use the K-means++ algorithm to recluster the prior boxes and replace Efficient IoU Loss as the new loss function, which has better matching and convergence on the insulator defect dataset we constructed. The experimental results demonstrate the effectiveness of our proposed algorithm. Compared to the original algorithm, our model reduces the number of parameters by 41.1%, while achieving an mAP@0.5 of 94.8%. It also achieves a processing speed of 32.52 frames per second. These improvements make the algorithm well-suited for practical insulator detection and enable its deployment in edge devices.
更多查看译文
关键词
improved yolov5s,lightweight network
AI 理解论文
溯源树
样例
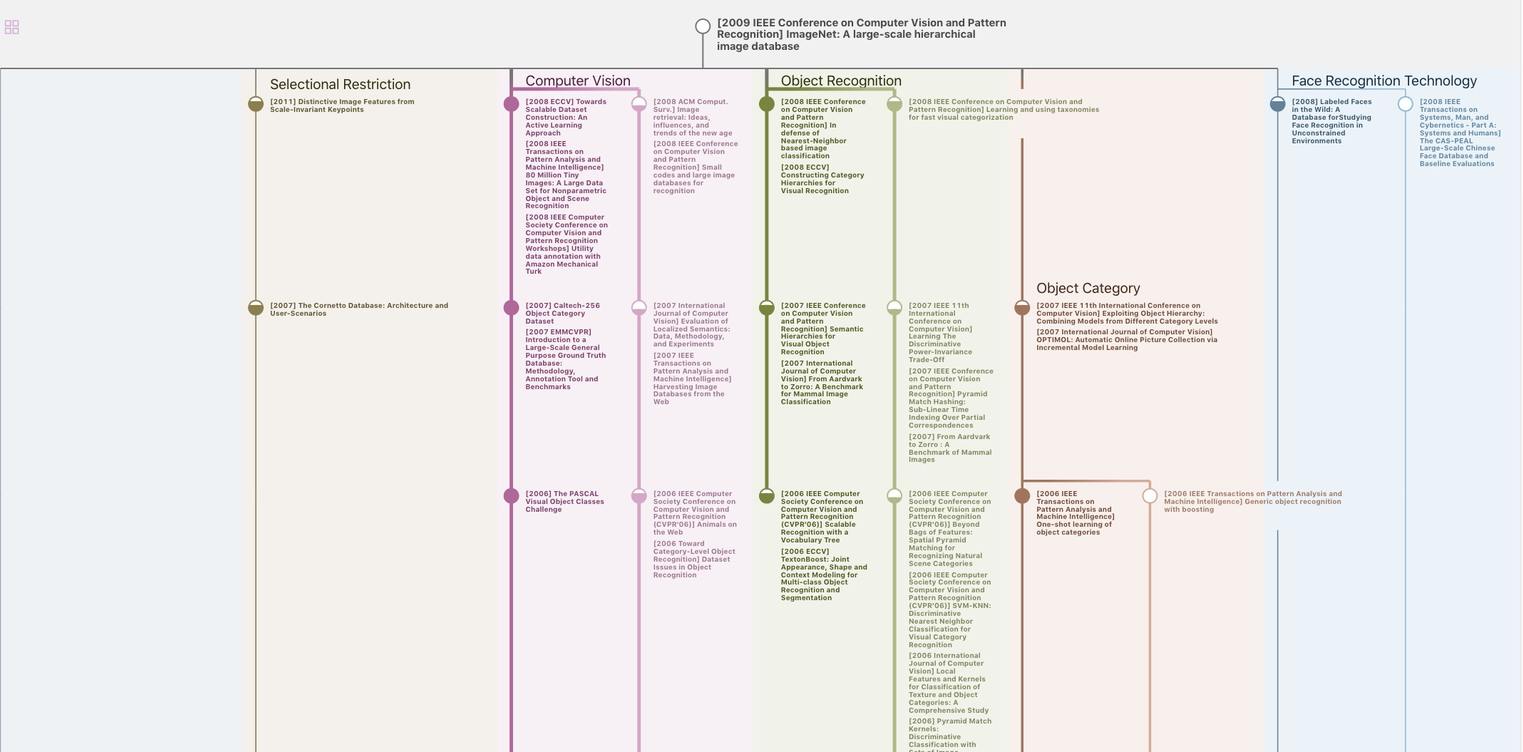
生成溯源树,研究论文发展脉络
Chat Paper
正在生成论文摘要